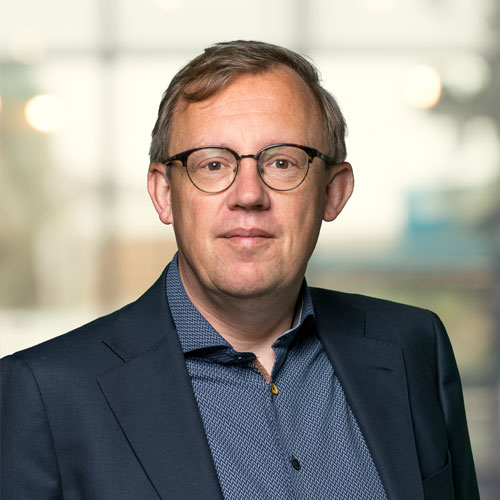
Frank Phillipson
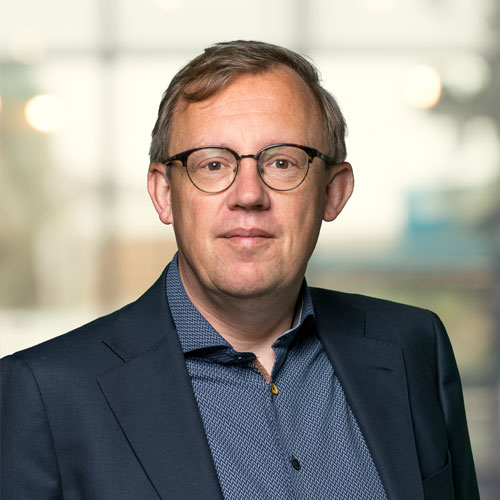
Frank Phillipson is senior scientist and professor at Maastricht University in Computational Operations Research, specialized in optimization in telecommunication, energy and logistics networks. Most of our current work is focused on finding new computational techniques for optimization and other hardware accelerators.
Professorship chair
Computational operations research (Maastricht University).
Research area
Many combinatorial Operations Research and Data Science problems are hard to solve, inherently. These problems can be found in several domains, such as network optimization and scheduling in ICT networks (underlying Smart Grids), Logistics and Transportation, and energy networks, planning and scheduling in industrial processes, and in Defense operations.
Adding uncertainty to the used data and to the realizations, makes solving these problems even harder. Currently, also the systems that are underlying these problems are getting more and more complex, interdependent and evolving in time, increasing the need for (more) decentralized or even self-optimizing, adaptive methods. It is important to find the right balance between (fully) decentral and central control in those systems, where decentral control may lead to sub-optimal solutions and central control may lack information. Adaptive methods ask for shorter calculation times. Both systems with human interaction and self-controlling systems, faced with uncertainty, are not adaptive (enough) if calculation times are long. To realize this, we have to combine all mathematical tools we can think of. From the algorithm side we have to combine methods and solution approaches from Operations Research and Data Science, e.g., exact methods (e.g., solving integer linear programming problems and non-linear programming problems), problem-based heuristics, hyper- or meta-heuristics, bio-based heuristics, machine and deep learning (e.g., reinforcement learning), and stochastic or robust optimization. We should use combinations of these methods, which will amplify their mutual strength and their explainability. Internationally this is also seen as the field of Decision Intelligence, augmenting Data Science with theory from other areas such as decision theory and management science.
This all has to be seen in the light of the evolution of computing technology. The availability of faster CPUs, the rise of GPUs and cloud computing already brought us many opportunities. This evolution will go on, in the direction of quantum computing, which could give a spectacular speed up in heuristics and exact optimization techniques: in the nearby future on the adiabatic quantum computer, using quantum annealing; followed by the gated quantum computer, which is expected to deliver its quantum supremacy by Grover’s search algorithm and its descendants.
Top publications
- ‘Data granularity and the optimal planning of Distributed Generation’, L. Kools and F. Phillipson, Energy, 2016.
- ‘Machine learning in the quantum era’, N.M.P. Neumann, F. Phillipson, R. Versluis, Digitale Welt – Quantum Computer special, 2019
- ‘Mathematical Formulation of Quantum Circuit Design Problems in Networks of Quantum Computers’, J. Mulderij, R. van Houte, T. Attema, I. Chiscop, F. Phillipson, Quantum Information Processing, vol. 19, 141, 2020
- ‘Power network optimization: a quantum approach’, G. Colucci, S. van der Linde, and F. Phillipson. IEEE Access (2023)
Den Haag - New Babylon
Anna van Buerenplein 1
2595 DA The Hague
The Netherlands
Postal address
P.O. Box 96800
2509 JE The Hague
The Netherlands