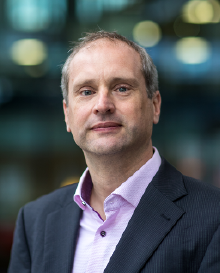
Frank Willems
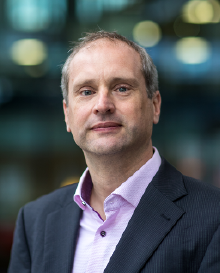
To accelerate the transition to climate neutral mobility, the development of self-learning control systems for sustainable powertrains is crucial to guarantee minimal energy consumption and operational costs over life time and to minimize development time and costs.
Professorship chair
Self-learning powertrains (Faculty Mechanical Engineering, Control Systems Technology, Eindhoven University of Technology).
Research area
The majority of goods are transported by trucks and ships, which are propelled by heavy-duty powertrains. These powertrains are mainly powered by internal combustion engines. In the coming decades, internal combustion engines will remain the primary power source. To support the transition to climate neutral mobility, the complexity of future powertrains will increase due to electrification and the introduction of waste heat recovery and highly efficient combustion concepts.
Control systems are the brain of the powertrain. These systems play an essential role in minimizing fuel consumption, in making vehicle performance robust for real-world driving conditions, and in enabling the use of a wide range of sustainable fuels, including green hydrogen. As powertrain development time and costs will reach unacceptable levels with conventional control methods, industry is facing a turning point in the near future.
Development of self-learning powertrains is crucial to deal with the complexity and diversity of future ultra-clean and efficient vehicles and to minimize development time and costs. This requires integration of energy and emission management strategies at system level. My research concentrates on the development of self-learning control concepts, in which the energy efficiency of the total powertrain is optimized online by the application of smart sensors and route information.
Top publications
- Willems, R., Willems, F., Deen, N., & Somers, B. (2021). Heat release rate shaping for optimal gross indicated efficiency in a heavy-duty RCCI engine fueled with E85 and diesel. Fuel, 288, Article 119656. https://doi.org/10.1016/j.fuel.2020.119656.
- Xia, B. de Jager, M. Donkers & F. Willems (2020). Robust constrained optimization for RCCI engines using nested penalized particle swarm. Control Engineering Practice, 99, [104411]. https://doi.org/10.1016/j.conengprac.2020.104411.
- Willems (2018). Is cylinder pressure-based control required to meet future HD legislation? IFAC-PapersOnLine, 51(31), 111-118. https://doi.org/10.1016/j.ifacol.2018.10.021
Helmond - Automotive Campus
Automotive Campus 30
5708 JZ Helmond
The Netherlands
Postal address
P.O. Box 756
5700 AT Helmond
The Netherlands