Fair Machine Learning combats biases
An AI tool bases its calculations on data. If the data is biased, the calculations will be biased. If there was once a male preference within a profession, then this will be adopted by AI tools for recruitment. So the AI tool may wrongly give a better judgement to men. This can be prevented by de-correlating the data from gender. Gender and possible related proxies will no longer be predictive for job suitability. TNO expects to use Fair Machine Learning to select appropriate candidates in a fair and unbiased manner.
TNO makes generative adversarial network models using fair machine learning
TNO carries out the de-correlation for Fair Machine Learning using a Generative Adversarial Network (GAN) model. This model tries to balance two conflicting criteria:
- Minimising the number of changes to the dataset
- Making sure that somebody’s gender is no longer identifiable from the remaining characteristics
When weighing up the criteria, the model generalises the existing characteristics of individuals into more general characteristics. An example would be generalising postcodes according to neighbourhoods, neighbourhoods according to cities and cities according to countries. The end result is a dataset in which a person’s gender (criterion 2) is practically unrecognisable. In short, the gender bias has disappeared from the dataset.
Fair machine learning is relevant to all forms of discrimination arising from historical data
Fair Machine Learning is relevant to all forms of discrimination and prejudice that arise from the use of biased data. In addition to recruitment and selection, it is also important that the AI algorithm is fair when it comes to supervision, inspection and enforcement tasks. Gender, religion and ethnicity should not be used as selection characteristics.
If used responsibly, AI machine learning tools can increase efficiency and effectiveness when finding comparable individuals for all kinds of selection tasks. However, historical biases (which are less striking without these AI tools) are being structurally and systematically furthered by them. Fair Machine Learning reduces and prevents such discrimination.
Get inspired
Working on reliable AI
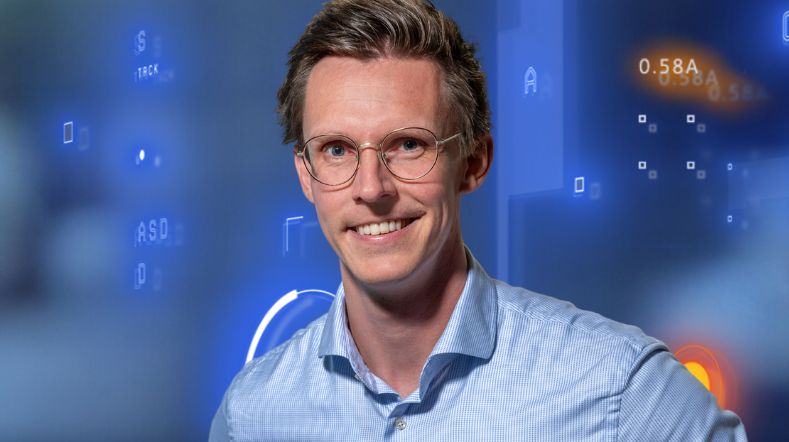
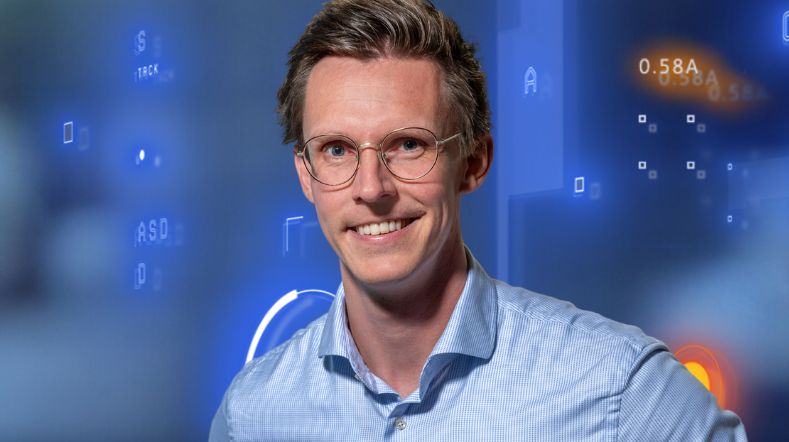
AI model for personalised healthy lifestyle advice
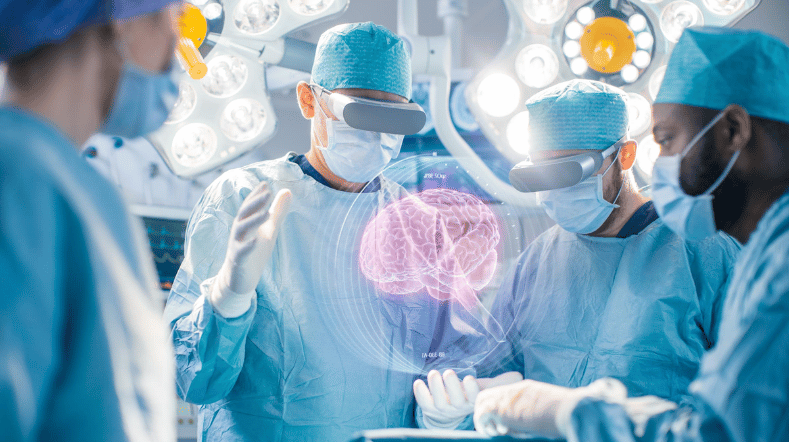
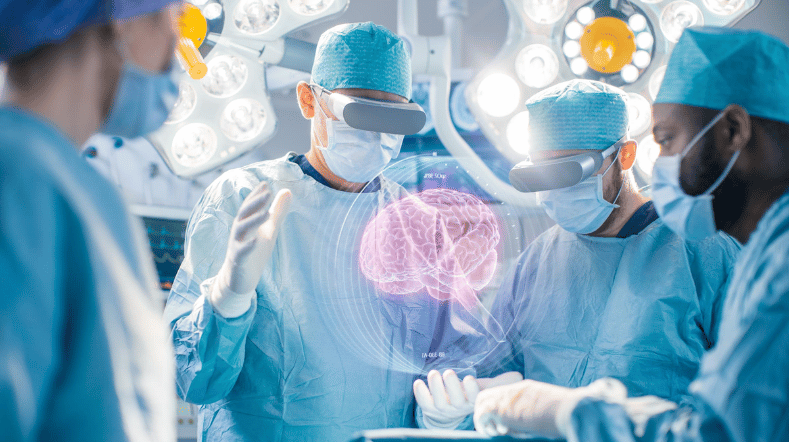
AI in training: FATE develops digital doctor's assistant
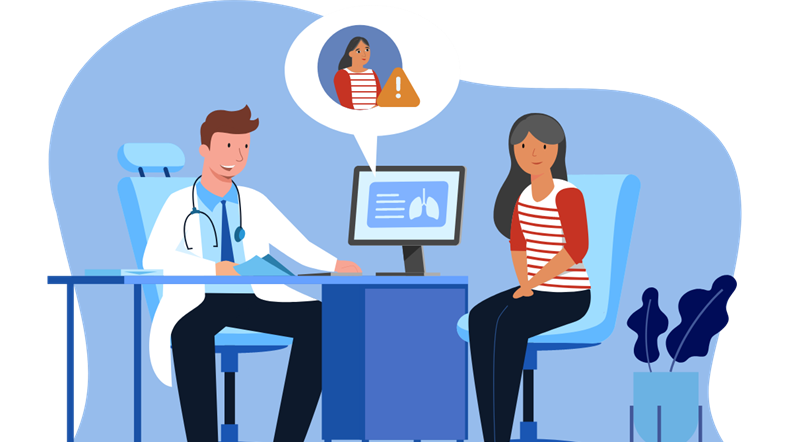
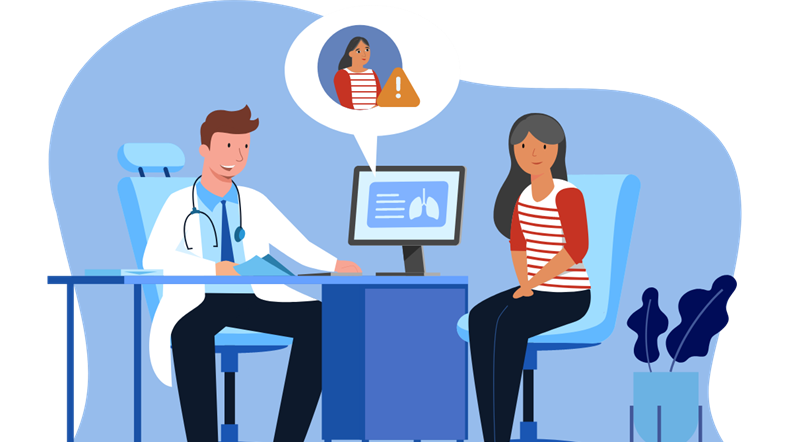
Boost for TNO facilities for sustainable mobility, bio-based construction and AI
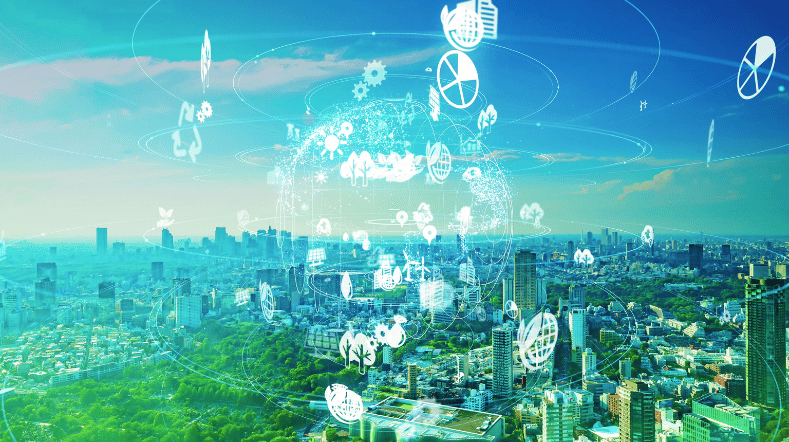
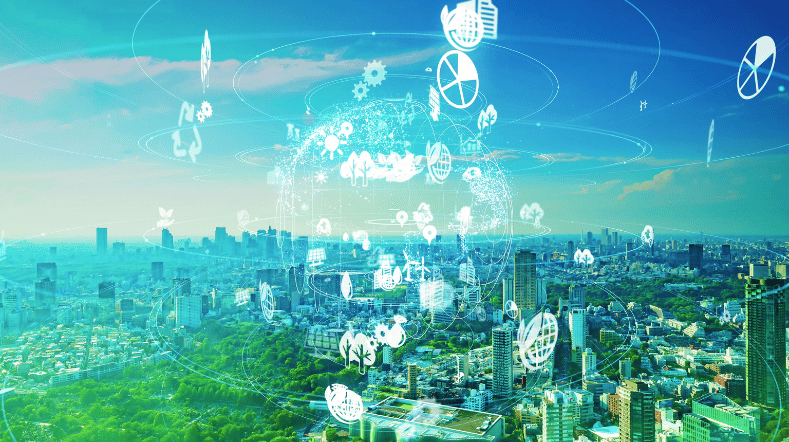
GPT-NL boosts Dutch AI autonomy, knowledge, and technology
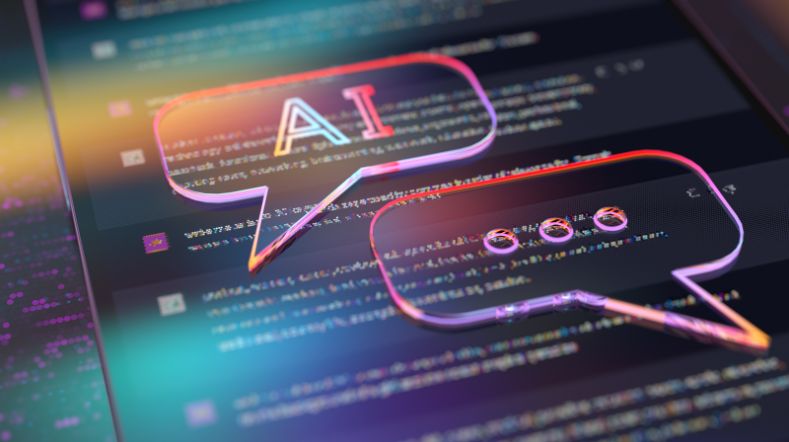
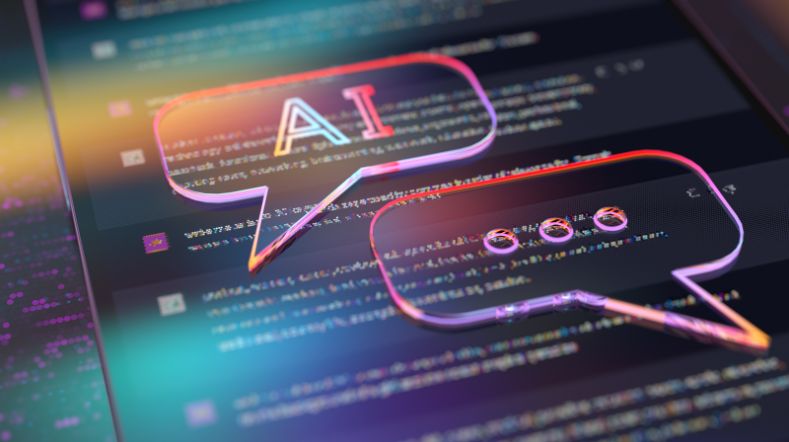