Artificial intelligence
We use artificial intelligence (AI) to develop innovative solutions that keep us healthy and safe. Find out more about our research programmes.
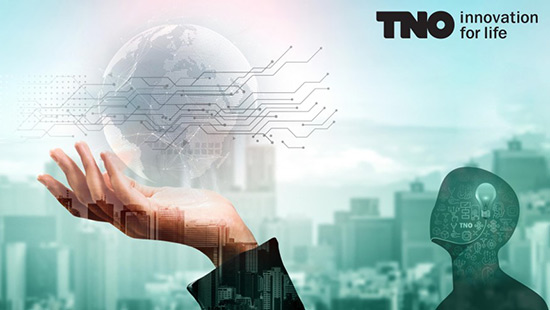
Appl.AI: from research to application
Within the Appl.AI research programme, we are working to make AI systems work in a world full of uncertainties. In addition, we focus on efficient cooperation between humans and machines.
Our latest developments
44 resultaten, getoond 1 t/m 5
Working on reliable AI
His research helps make AI models reliable, even when there is little data available for learning. Meet Friso Heslinga, computer vision scientist and one of the key researchers and nominee for TNO’s Young Excellent Researcher award.
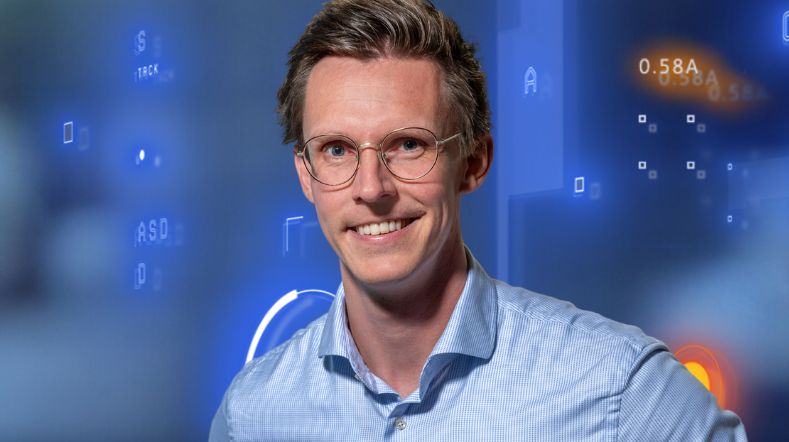
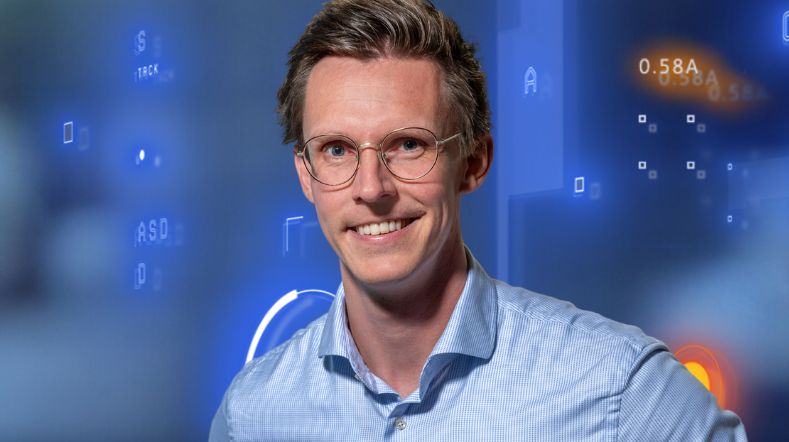
AI model for personalised healthy lifestyle advice
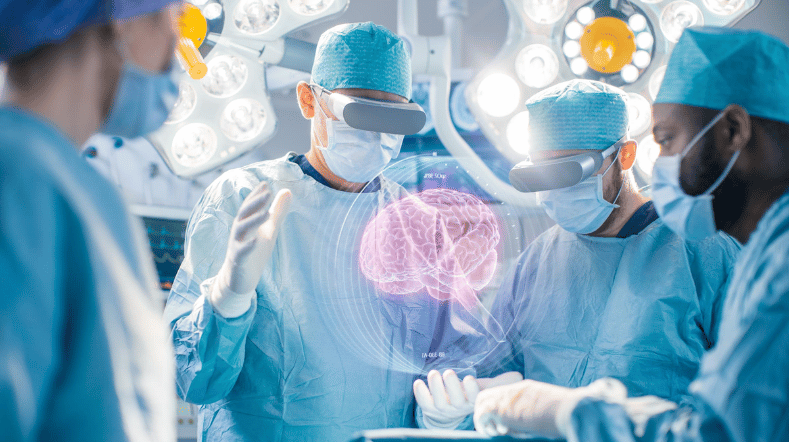
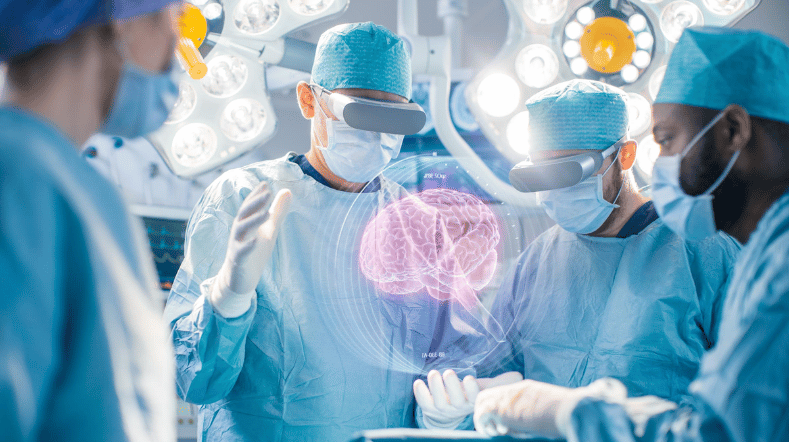
AI in training: FATE develops digital doctor's assistant
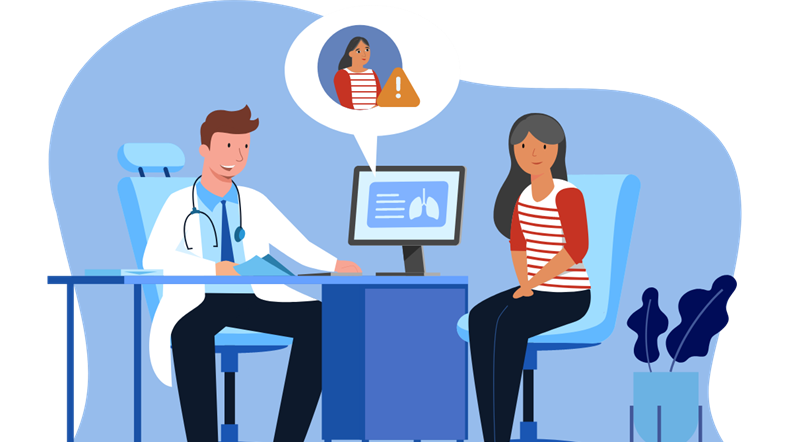
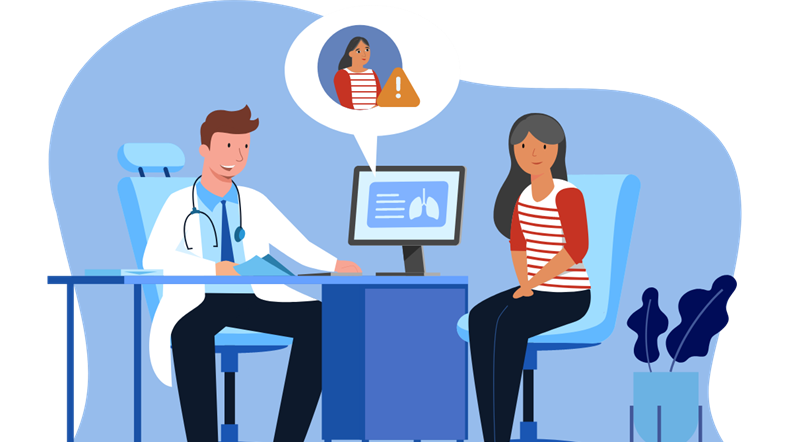
Boost for TNO facilities for sustainable mobility, bio-based construction and AI
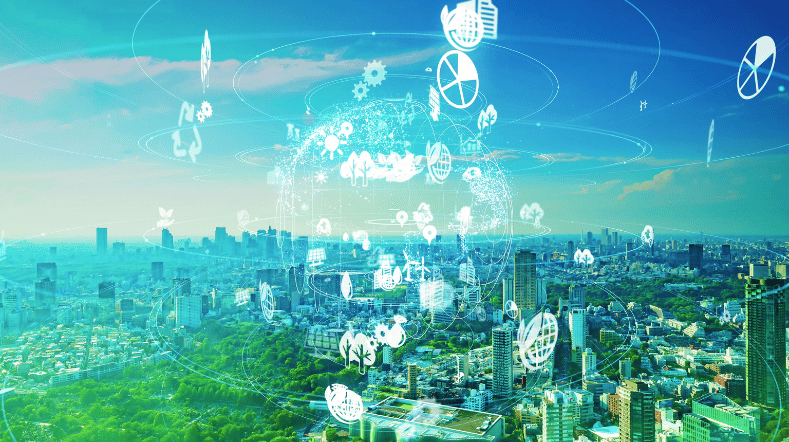
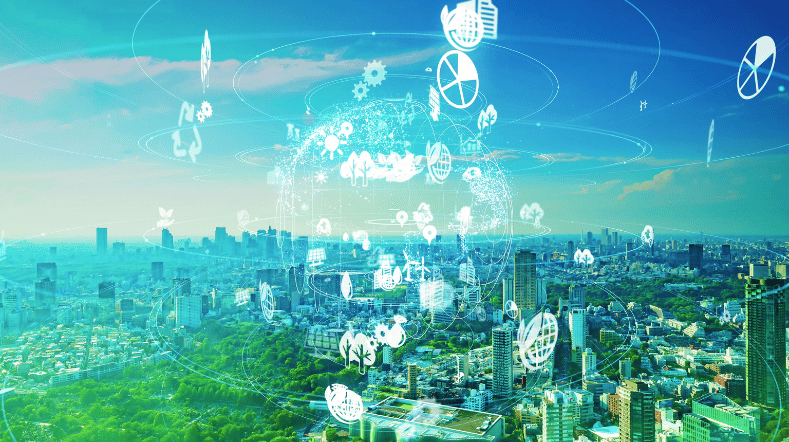
GPT-NL boosts Dutch AI autonomy, knowledge, and technology
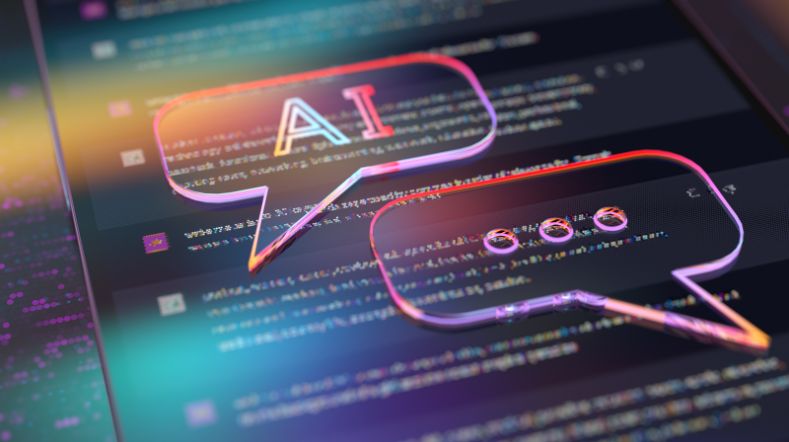
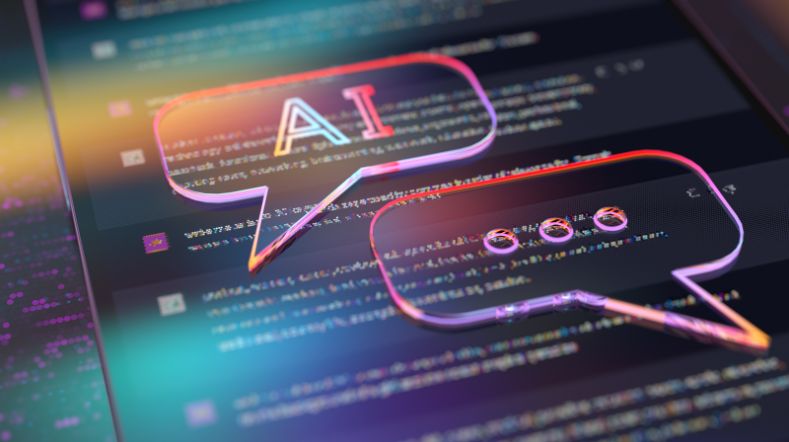