Federated learning: get to know privacy-preserving data analysis
The use of privacy-sensitive data has an enormous potential to help improve society. Examples include new insights that help us with the energy transition or our health. However, you cannot use these data without the permission of citizens or companies, not even if it would benefit them. Fortunately, there is a privacy-friendly way to solve that problem in certain cases: Federated Learning.
What is Federated Learning?
Federated Learning is a decentralised and privacy-friendly form of machine learning. This means that there is no need for a central database to hold all of the sensitive data, so these data cannot be leaked. Instead of bringing the data to the machine learning model, Federated Learning brings the machine learning model to the data.
In this way, the training of the models is broken down into sub-calculations that are performed locally at an organisation. After carrying out the calculations, only the anonymised (intermediate) results are shared with the organisations conducting the research, not the privacy-sensitive data itself.
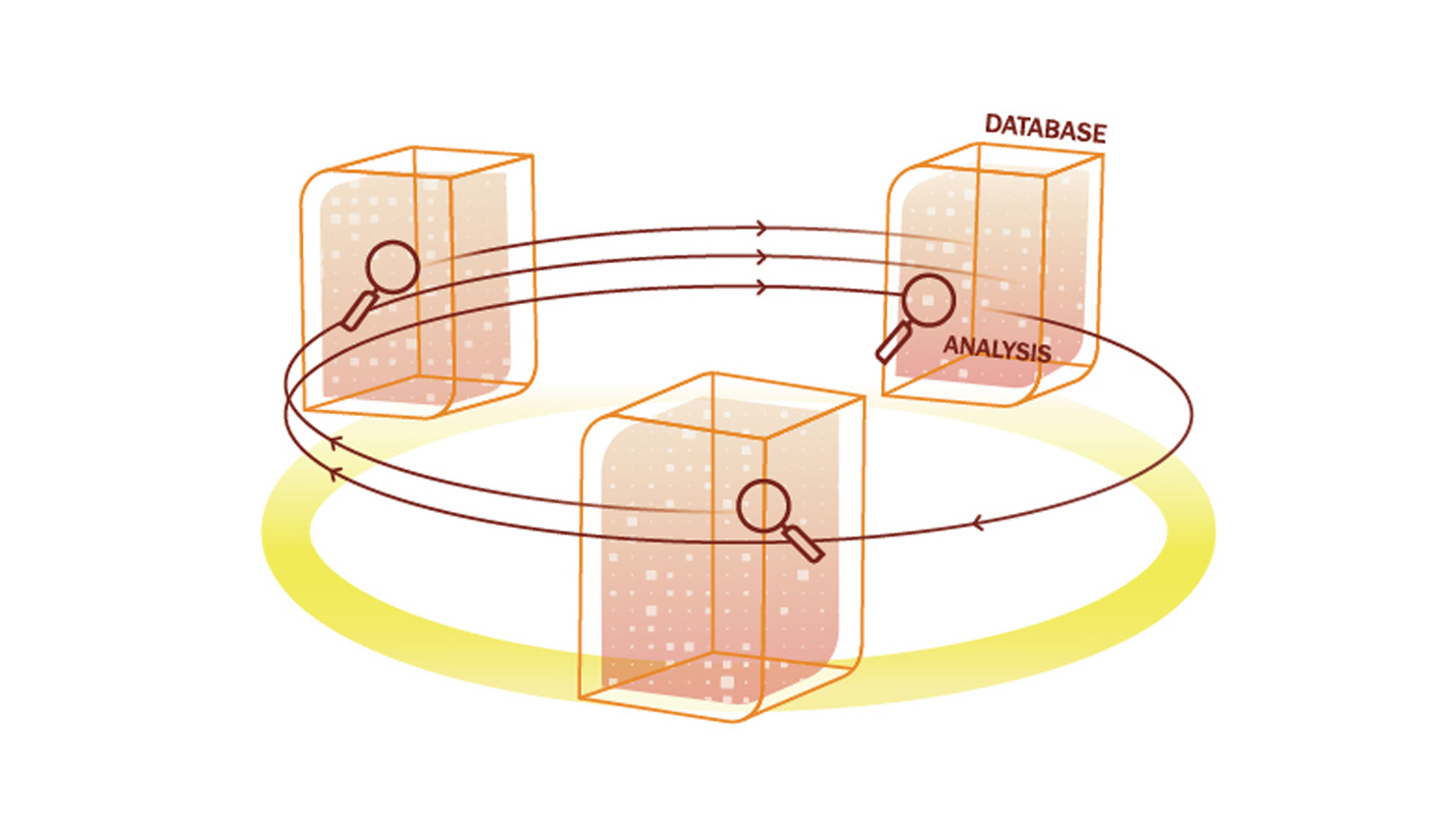
Whitepaper: "Finally, a privacy-friendly way to harness data"
Discover how you can use data without violating privacy
Which problems does Federated Learning solve?
Federated Learning solves two major problems of data analysis: improved qualitative analyses for society and safeguarding of citizens’ rights in relation to privacy.
These days, analysing large amounts of data is easier than ever before. Computing power is increasing and algorithms are becoming ever more advanced. Although more and more data is available for valuable analyses, there are an increasing number of societal objections to the use of sensitive data.
What can organisations gain from Federated Learning?
Federated Learning allows you to harness data without violating privacy. The amount of available data is greater as you can analyse data from multiple databases. In turn, the results of a study are more reliable. This means better predictions and better models, leading to much more informed (policy) decisions.
An example? Take cancer research. With Federated Learning, you can analyse anonymised data on things like successful treatment methods for each type of cancer in different people across all hospitals without violating patient privacy.
What does TNO do in the field of Federated Learning and how can you work with TNO?
No less than 8.5% of the world’s population has diabetes, 90% of which is Type 2. This is particularly distressing as it can often be prevented by different lifestyle choices, for example.
How can machine learning (ML) help? By identifying risk groups and warning them as early as possible, i.e. before Type 2 develops. To do that, the ML model needs to be trained as well as possible on a large number of people with different lifestyles and medical conditions in multiple age groups.
To do this properly, you need many different data sources from healthcare institutions. However, medical data is so well protected under the GDPR that sharing is almost impossible – let alone storing all that sensitive data in a central database to run models on it.
How Federated Learning contributes
TNO has collaborated with the organisation Lifelines to create a Federated Logistic Regression model to predict the emergence of Type 2 diabetes for people aged between two and eleven. Via Lifelines, data from 167,000 people in the Netherlands were analysed in a privacy-friendly manner.
The data comes from various sources, including data from proteins tested in the lab and surveys of people regarding their lifestyle. This resulted in a Federated Learning model that works almost as well as a globally trained model. The results are promising and justify further research.
The energy transition is already a huge topic and will only grow larger in the coming years. An important issue is predicting energy demand in order to match supply and demand.
You have to know how much grid capacity is needed in order to be able to deliver a supply that matches the energy demand, preferably per neighbourhood so that smart supply can be implemented. But requesting your energy consumption is difficult. It can cause privacy issues, like a third party may know when you are not at home.
How Federated Learning contributes
Together with partners like Strukton, TNO has been working on a model for predicting a neighbourhood’s energy demand. This is done in a privacy-friendly manner using Federated Learning. This means that the personal data of households do not have to be viewed by researchers or stored at a central location, thereby preventing loss of privacy
Instead, the energy demand per neighbourhood can now be analysed and predicted in a privacy-friendly manner. On this basis, it can be determined how much capacity is needed. What a result! With the help of FL, the energy demand of a neighbourhood can be predicted without violating the privacy of citizens and with the aim of guaranteeing a stable electricity supply.
Get inspired
Digitalisation and sustainability: how AI can help
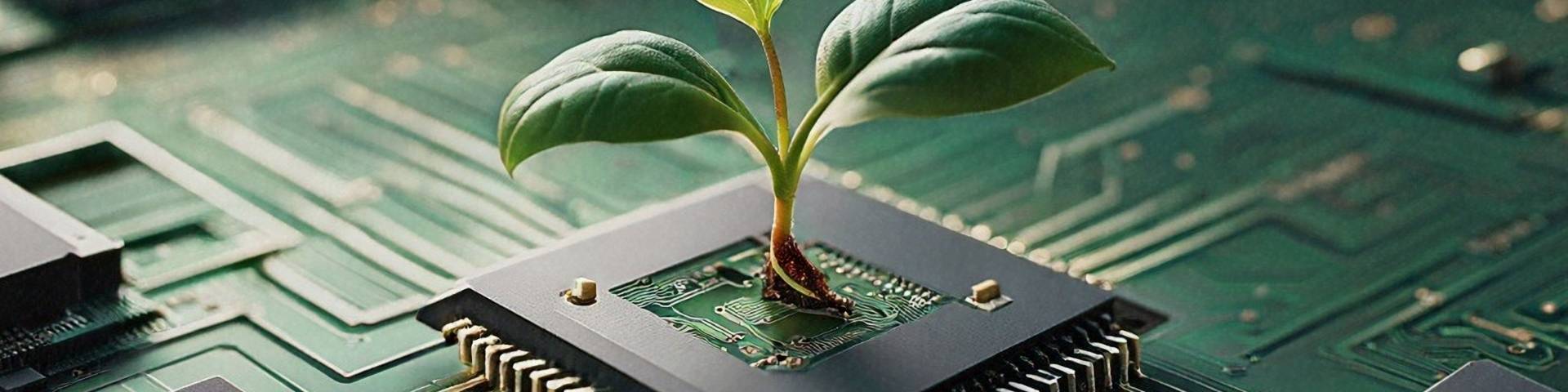
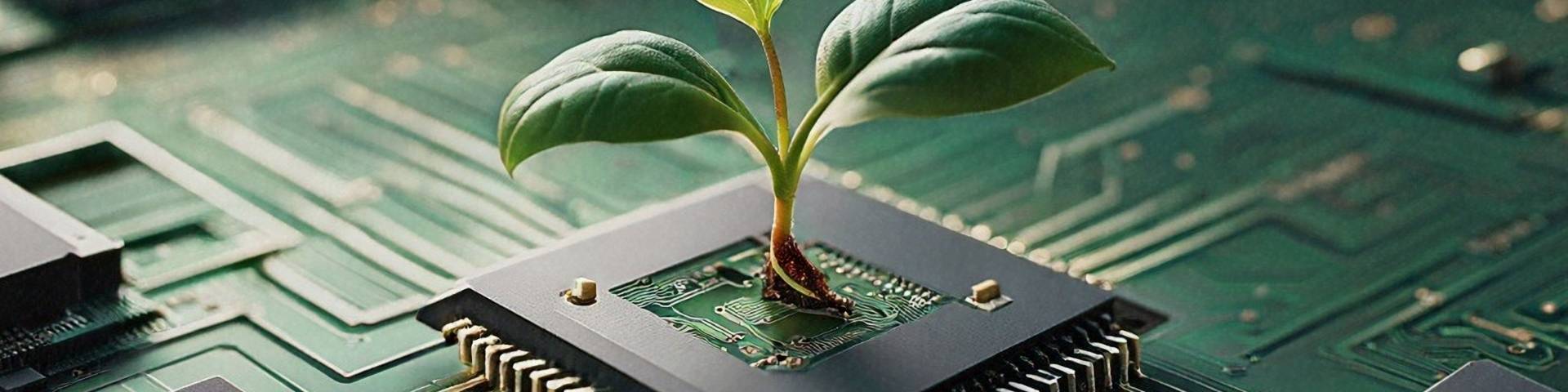
Time setter story: Kallol Das
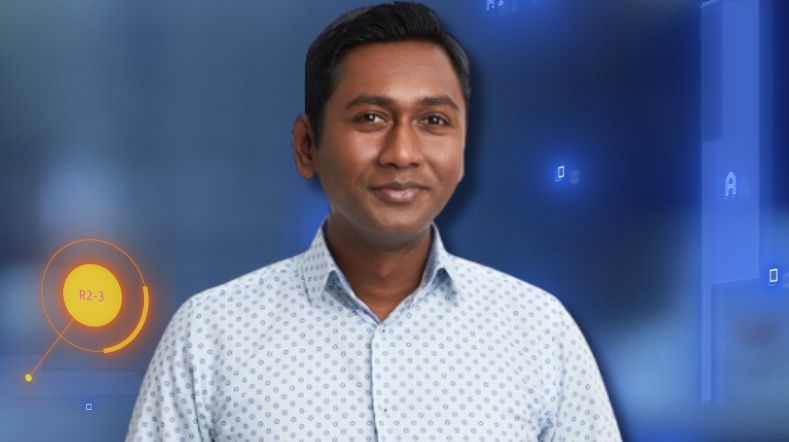
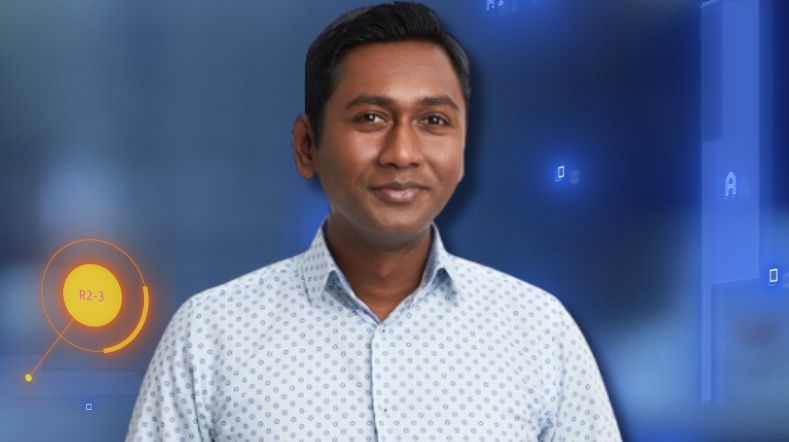
Digital Product Passport
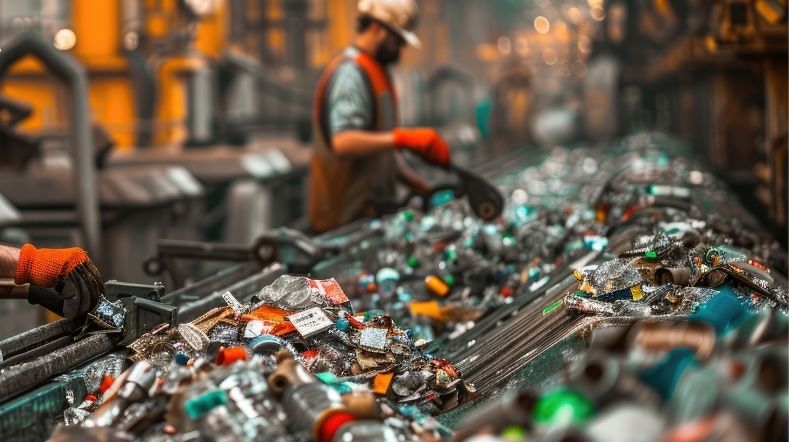
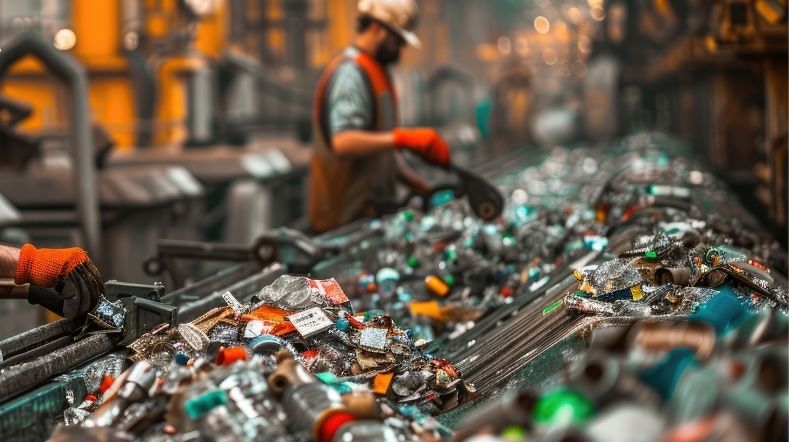
Time setter story: Annemieke Kips
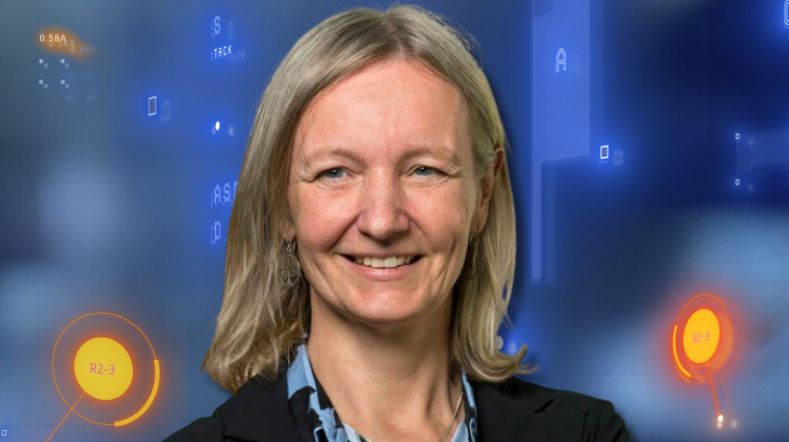
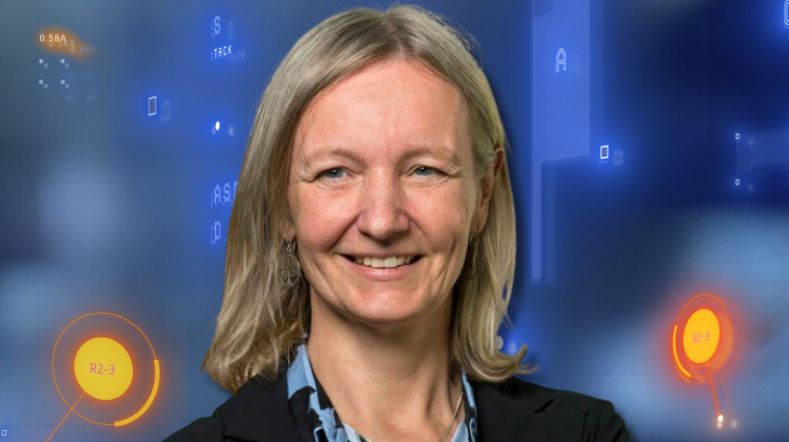
Time setter story: Belma Turkovic
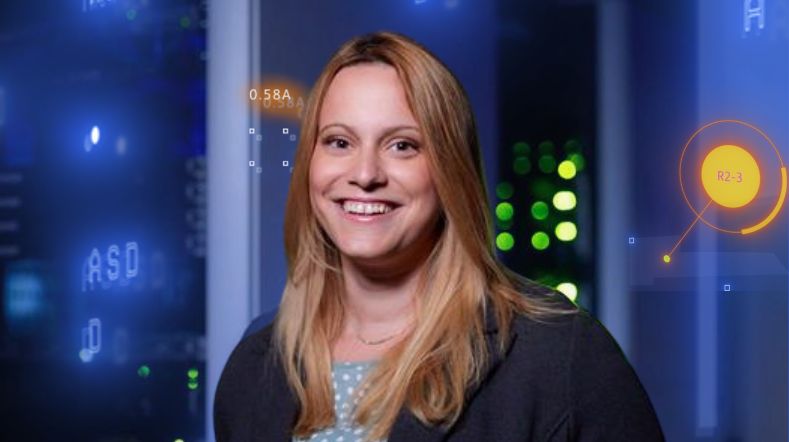
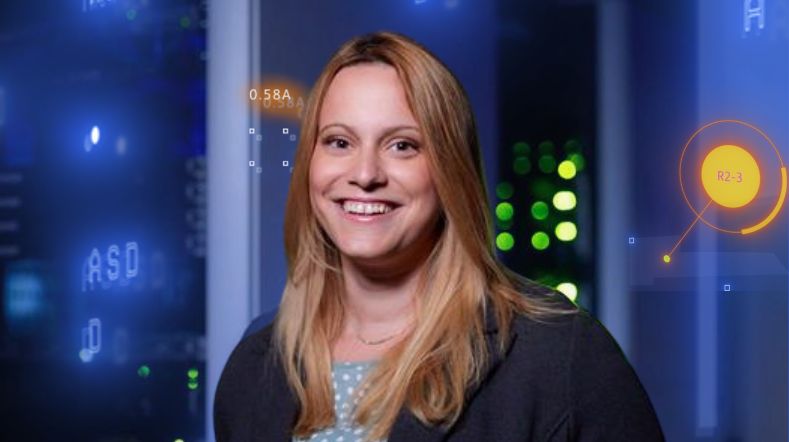