Diagnosing for printer maintenance with AI
When exactly does a building or item of equipment require maintenance? Carrying out too much preventive maintenance can be costly. But if you leave it too long, the expense of an emergency repair can be much greater. And we all know that machines have a habit of breaking down at exactly the wrong time. So predictive maintenance is high on the list of priorities of the manufacturing industry. Here, too, artificial intelligence (AI) can make all the difference.
A customer is itching to receive the printed matter he has ordered. The printers are running flat out – as are all the other machines. It is not just tough quality requirements that the graphic industry has to meet, but in many cases tight deadlines as well.
That makes it an ideal sector for introducing predictive maintenance based on artificial intelligence. And that is exactly what TNO and Canon Production Printing (formerly Océ) are currently aiming to do. Together, they are carrying out research into an AI system that stands out in terms of reliable diagnoses and prognoses for professional printers.
AI that understands printers
A lot of different data is needed to be able to estimate the condition of a machine or machine components. But much of that data is incomplete or unreliable. To be able to extract the right conclusions from this mix of data, you need AI that is entirely at home in the world of printers.
This calls for 'hybrid AI' – a combination of data-learning AI and domain knowledge. The AI system is able to modify the likelihood of causal links in the domain model on the basis of a machine’s user data. It can also work out what problems could occur and in what kind of time frame.
Still essential: human expertise
And what about people? The part they play should not be underestimated. Machine learning, then, is just one part of the story. It is precisely the combination of artificial intelligence and human expertise that make this solution so powerful. The big challenge here is to develop an AI system that is capable of accurately combining the input from people and machines alike.
Get inspired
GPT-NL boosts Dutch AI autonomy, knowledge, and technology
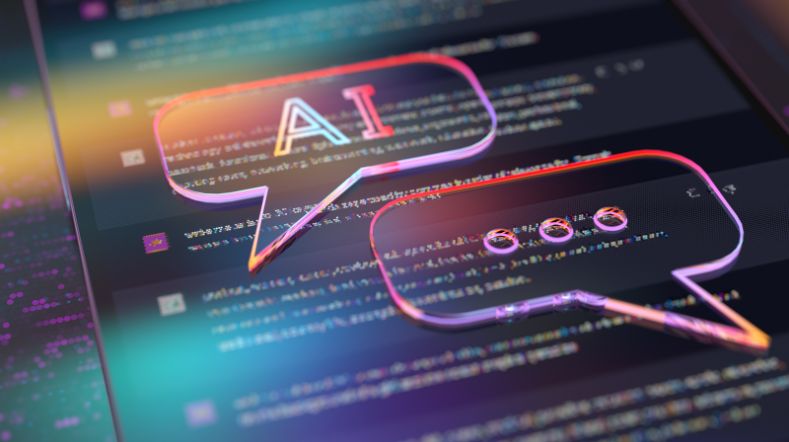
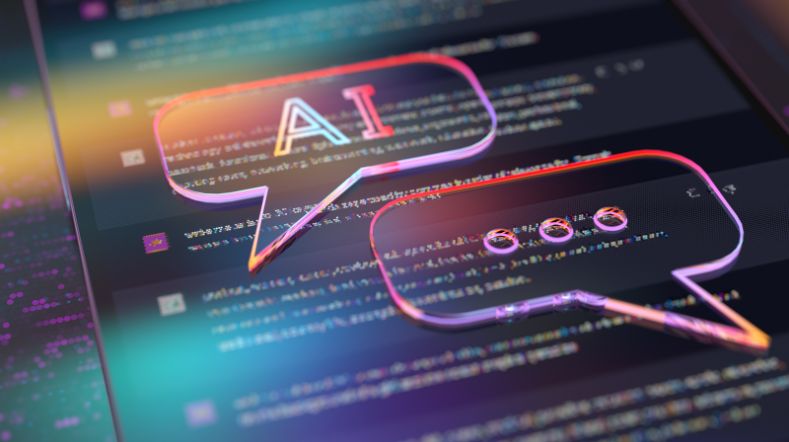
System integration for robots in greenhouses
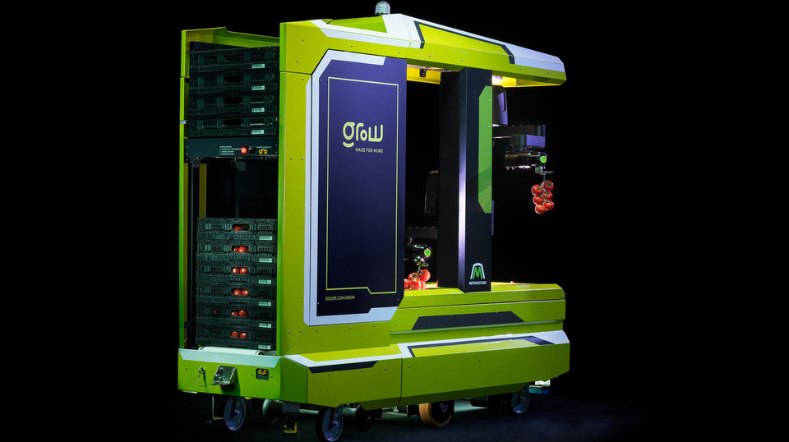
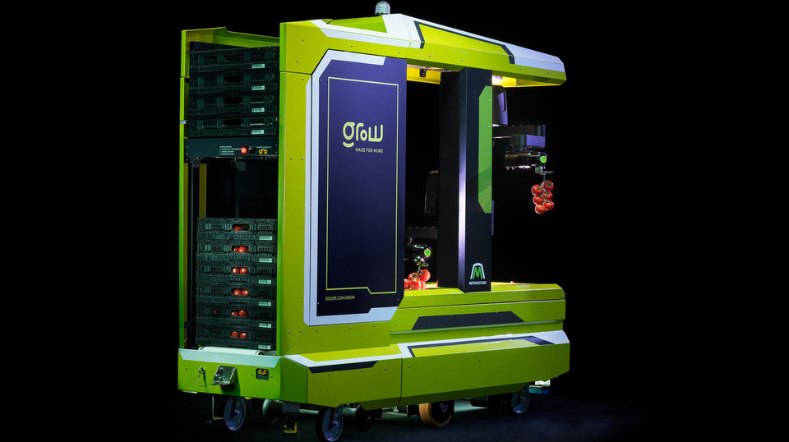
AutoAdapt: Self-adaptive machine learning to propel us into the future
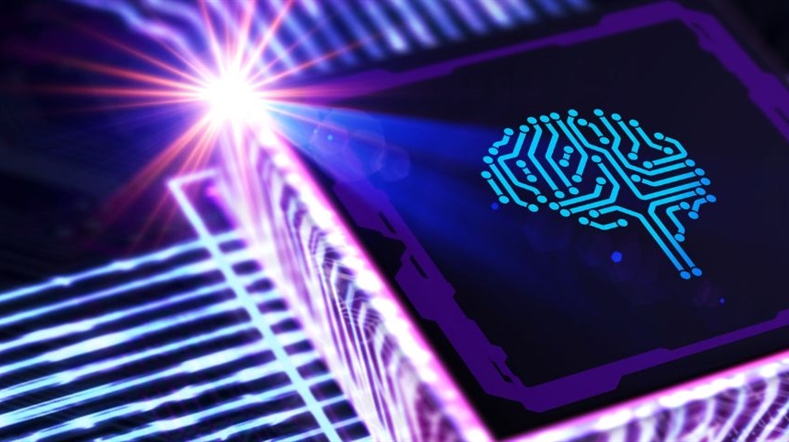
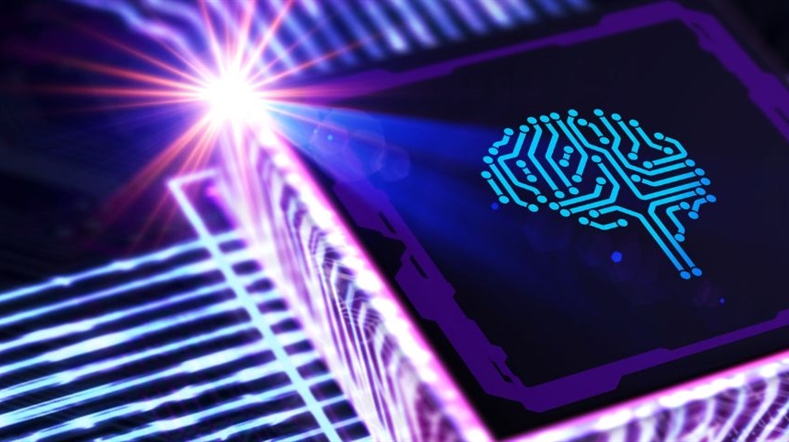
Developing moral models for AI systems
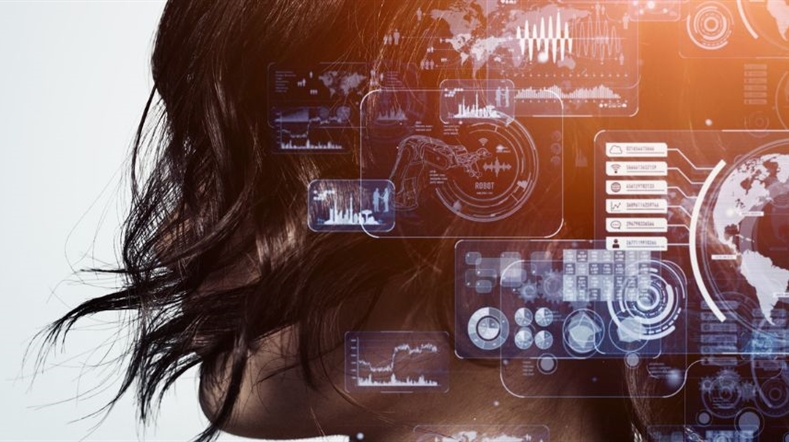
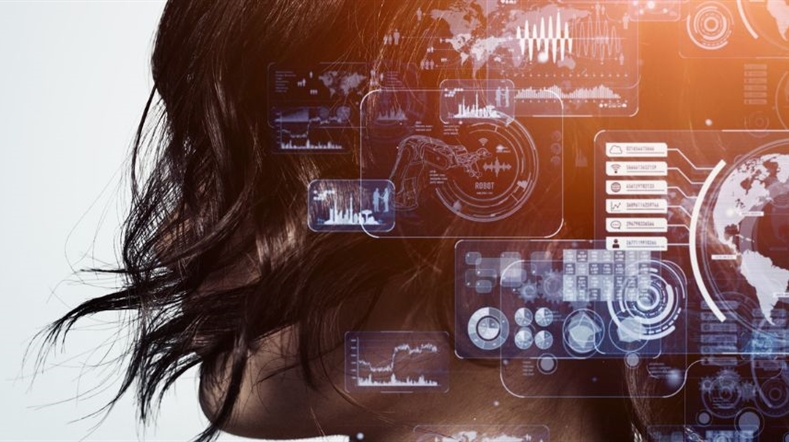
AI Systems Engineering & Lifecycle Management
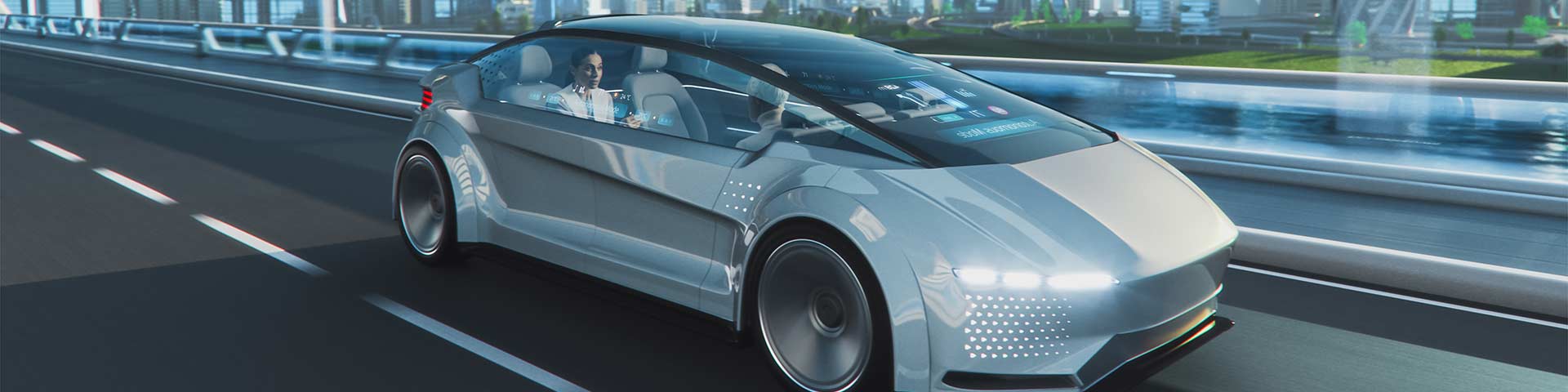
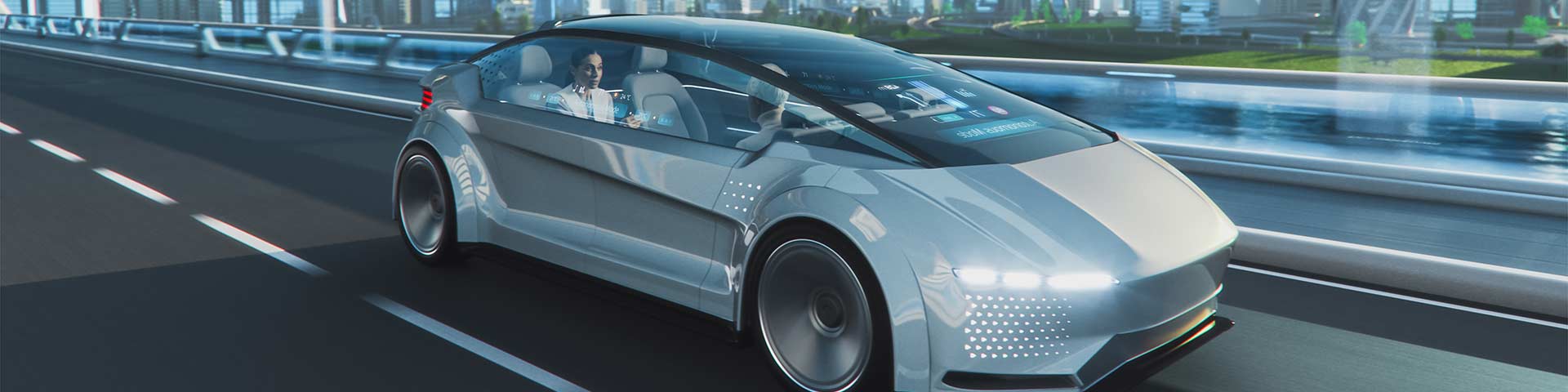