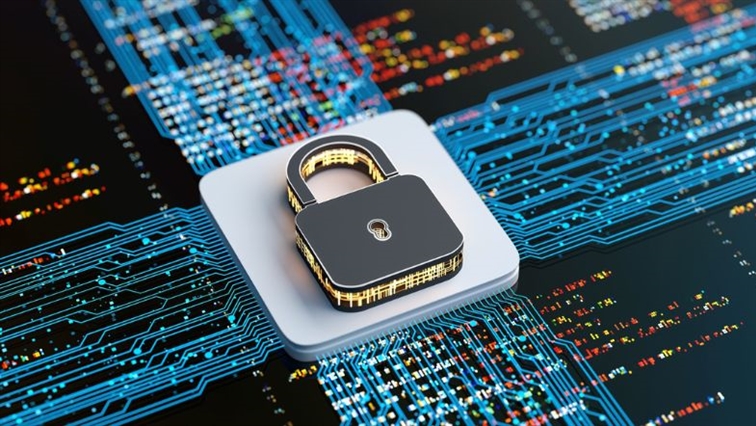
Defending against Adversarial AI attacks: an overview
As AI applications and capabilities continue to progress rapidly, so do efforts into exploiting its vulnerabilities, mainly through the Adversarial AI research field. As these trends persist, AI robustness becomes an increasingly vital topic for those developing, implementing or simply working with Machine Learning models.
To harness the potential of AI while ensuring security, defences against these Adversarial AI attacks should be developed and implemented. However, while the current research field is progressing rapidly, it features a distinct lack of structure and clarity, making it difficult for defenders to effectively select the appropriate defences for their Machine Learning models. Additionally, this lack of overview in this research field could risk research efforts not addressing the developments within the field.
Defending against Adversarial AI attacks
Building on last year’s whitepaper (pdf), which reviewed the five main types of Adversarial AI attacks, TNO now contributes a first overview of existing defences against all five types of attacks targeting Machine Learning models in the cyber domain. Using the insights this overview presents, the whitepaper outlines several overarching trends occurring in the defence field. This equips developers and decision-makers with vital knowledge for selecting the appropriate defences for their models and highlights for researchers the aspects of the defence field that are still lacking.
Although this overview provides the previously missing structure and clarity, ongoing research is necessary to properly evaluate the defenses and verify their claimed performance, which remains a significant challenge in this field.
Read more about:
Robustness of Machine Learning Systems: an Overview of Defences against Adversarial AI Attacks.