LANCELOT: new collaboration between IKNL and TNO to enable privacy preserving analyses on cancer-related data.
The LANCELOT project is part of an ongoing strategic collaboration between the Netherlands Comprehensive Cancer Organisation (IKNL) and TNO, together with Janssen Pharmaceutica NV, to develop data infrastructure and AI solutions to enable the use of sensitive health data. LANCELOT will help to reduce the impact of cancer while preserving patients’ privacy. LANCELOT focuses on problems where the data of patients are spread across different organizations, for example at the General Practitioner, the hospital and the pharmacy databases. We are looking for additional partners in healthcare, to bring our solutions to the next level by performing pilot studies, embedding the solutions within legal agreements, and using data in a privacy-preserving manner.
New insights into cancer are needed to help reduce the impact of cancer, by improving cancer care and prevention. This requires broad and rich data, for instance to develop models to evaluate treatment outcomes and the benefit of new therapeutic options using Real World Data. The Netherlands Cancer Registry, maintained by IKNL, with data from nearly all patients with cancer in the Netherlands, is a relevant data set for this purpose, but also data sets from other sources are needed.
For example, data recorded by General Practitioners may provide an understanding of the symptoms and condition of the patient before and at the time of diagnosis as well as of the treatment outcomes. Also, data on health status and cancer diagnosis could be related with long term outcomes such as quality of life and societal participation. In the future, prediction models developed on this data may be used to support (shared) decision making, for instance on which therapy to offer to patients.
New privacy-preserving technologies for data analysis
However, bringing such ‘vertically partitioned’ data (e.g., the cancer registration with data from primary care) together in a traditional way could compromise patient and institutional privacy. Emerging technologies like federated learning and secure Multi-Party Computation (MPC) enable analyses on data sets without having to share or reveal the underlying sensitive data.
However, state of the art does not yet support complex analyses needed for studies with so-called vertically partitioned data. Therefore, IKNL and TNO, together with Janssen, aim to investigate and develop new solutions to enable relevant analyses of multiple vertically partitioned data sets, without the need to share these data sets or compromise privacy. For Janssen and TNO, this investigation is part of an overarching intention to intensify our collaborations on health care related topics.
Open-source solutions
The goal of this collaboration is to design open-source privacy-preserving solutions that are generically applicable on vertically partitioned data sets by making use of MPC. We use (realistic) synthetic data for algorithm development and testing, to ensure that these solutions can be applied on real data in the future. We publish our solutions open source to make sure that others can contribute to and make use of them.
In particular, we develop new Proof-of-Concepts methods to:
- securely match patients in different data sets in the absence of unique common patient identifiers,
- perform data exploration on the partitioned data, and
- train a relevant machine learning model on the data, with a specific focus to balance accuracy and computation time.
The first results from LANCELOT, to securely train a machine learning model, are already made available open source. Developed solutions will be integrated into Vantage6, the open source Personal Health Train solution, and the intention is to test these solutions in an experimental setting on synthetic data.
As example use case, this project focuses on patients with non-small cell lung cancer. The developed solutions will help to train models on data from different sources to learn the impact of different factors (including patient characteristics and tumor characteristics) on the benefit of novel therapies. Over time, these models may be used to support (shared) decision-making on which therapy to offer to patients.
LANCELOT is partly funded by PPS-surcharge for Research and Innovation of the Dutch Ministry of Economic Affairs and Climate Policy.
Let yourself be inspired
TNO launches open source tool for automated cyber security
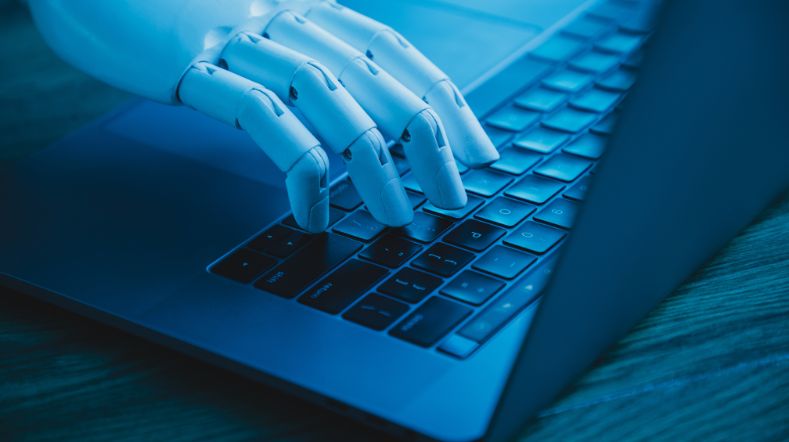
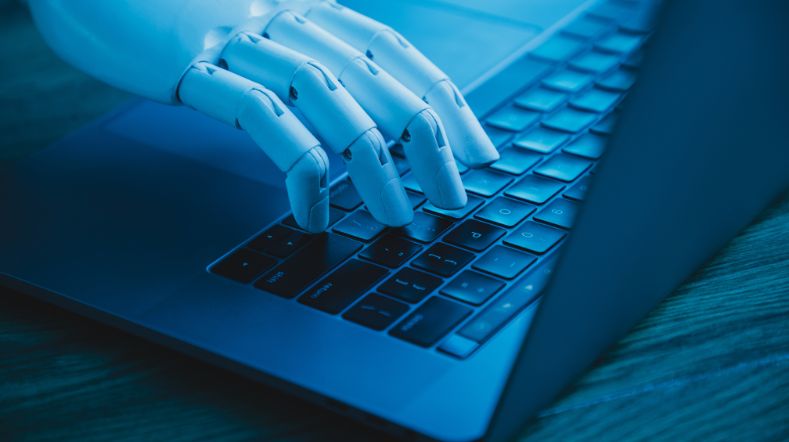
Quantum Application Lab receives grant for quantum computing application development
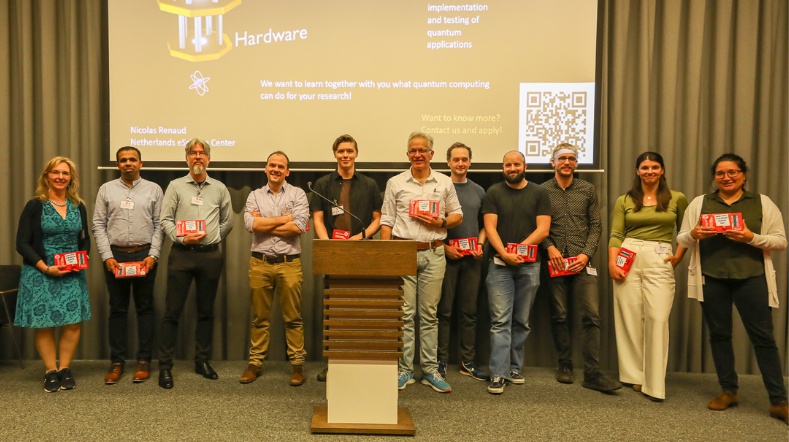
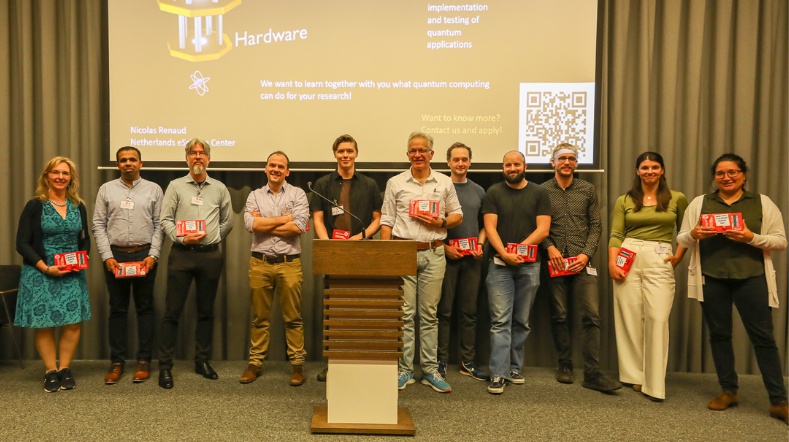
Successful demonstration system for automated cyber security in healthcare
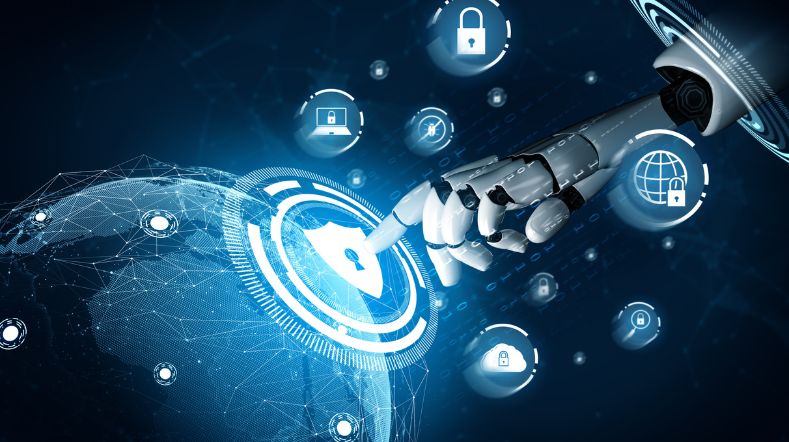
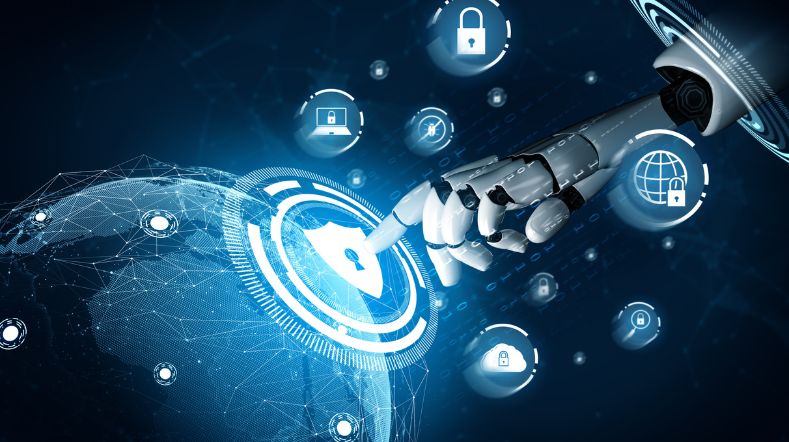
The future of cyber security: autonomous system of systems
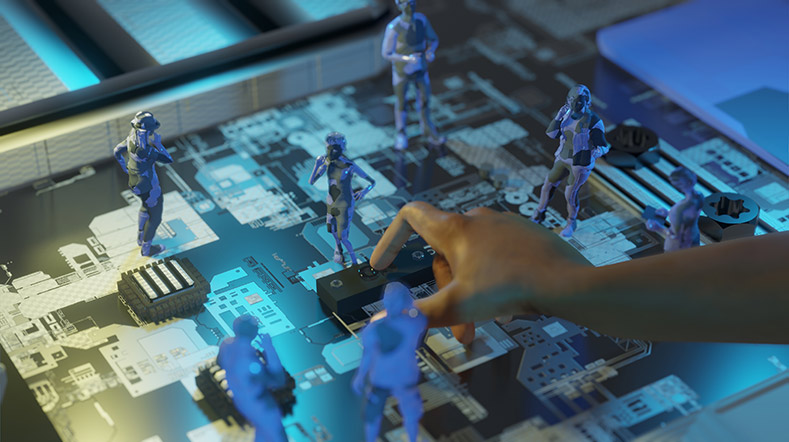
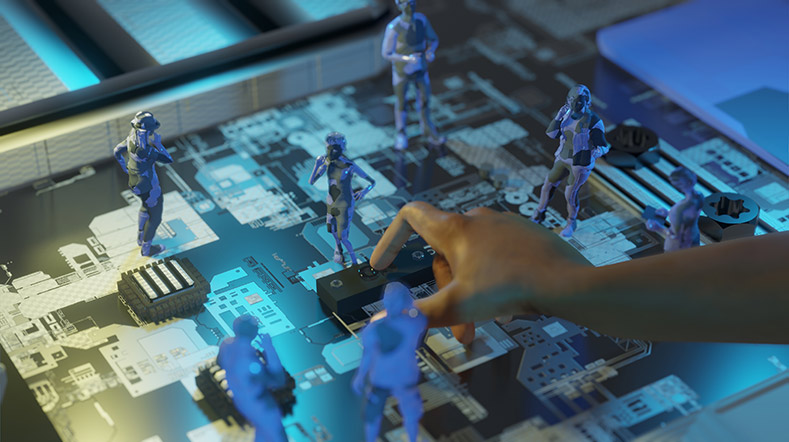
TNO’s view of 2030: Digital privacy and security for everyone

