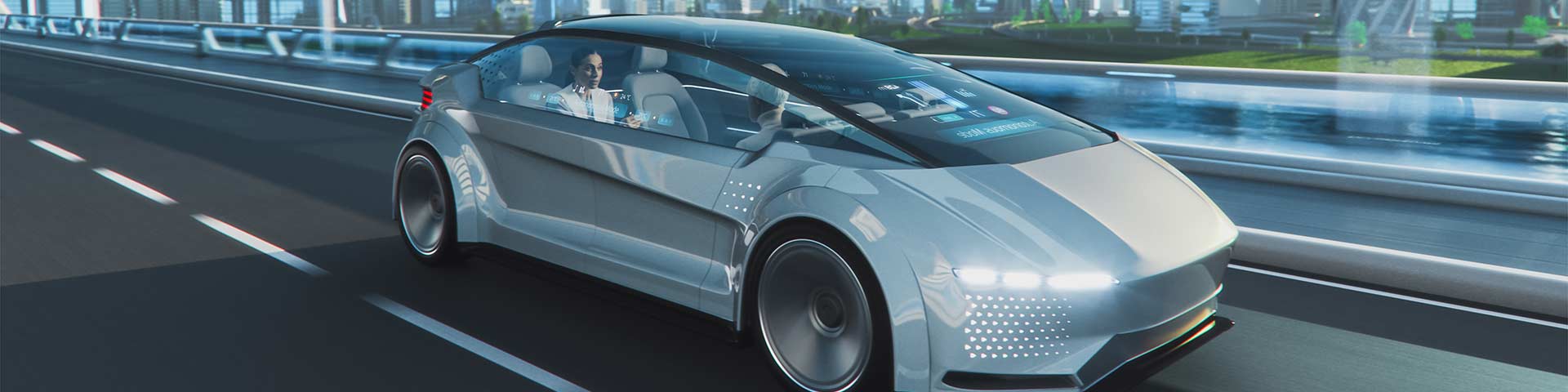
AI Systems Engineering & Lifecycle Management
To what extent can an AI system adapt to new laws and user requirements? And is such an AI system flexible enough to quickly respond to societal developments? For engineers, developing future-proof AI systems is a tremendous challenge.
Future-proof AI-systems
To start with, engineers must consider the needs and requirements of an AI system’s stakeholder and the environment in which it will be deployed. Furthermore, the right moral, ethical, and legal choices must be made and recorded.
And this doesn’t just need to happen during the development phase; doing so is most important during an AI system’s actual deployment. After all, the goal is to arrive at AI solutions that can demonstrably quickly and safely adapt to the demands and requirements of the future and its changing environments.
Continuously keeping an AI system up to date requires multidisciplinary engineers working closely with all stakeholders andmaking both technical and organisational adjustments.
AI learning
A key feature of AI is the fact that it’s a dynamic technology, featuring self-learning algorithms with ever-improving problem-solving capacities.
However, the more advanced AI systems become, the harder it becomes for humans to assess their reliability and capacity to give the right advice and make the right decisions in all foreseen circumstances. For some stakeholders, it even means drastically changing their way of working.
Learning how algorithms work
RDW, the Netherlands Vehicle Authority, is one of many organisations currently in full learning mode to keep up with the myriad implications of AI’s rapid development.
With an increasing number of organisations claiming it will soon be possible to safely drive self-driving cars on public roads, RDW inspectors need to not only understand the mechanical parts of cars, but also the algorithms that enable the (semi-)autonomous controlling of these vehicles.
A key question here is how type approvals should be set up in the future. After all, what’s the value of these approvals when vehicles receive new functionalities after a software update that affect their driving characteristics and road safety?
And this is just one of many examples of how AI may turn an existing situation on its head.
Four concerns for the lifecycle of autonomous AI
TNO is committed to developing autonomous AI systems that can be deployed safely and reliably throughout their entire lifecycle. To achieve this, we’ve distinguished four areas of attention:
during an AI system’s development, considering the requirements that will ensure its safe and reliable deployability and maintainability.
ensuring that AI algorithms can be reliably evaluated to demonstrate, both during their development phase and while in operation, that they meet all set requirements.
efficiently and effectively determining where and how to perform maintenance on AI algorithms (possibly in response to errors or changing requirements).
implementing and integrating ethical and legal guidelines in AI algorithms to comply with changing frameworks.
Get inspired
Boost for TNO facilities for sustainable mobility, bio-based construction and AI
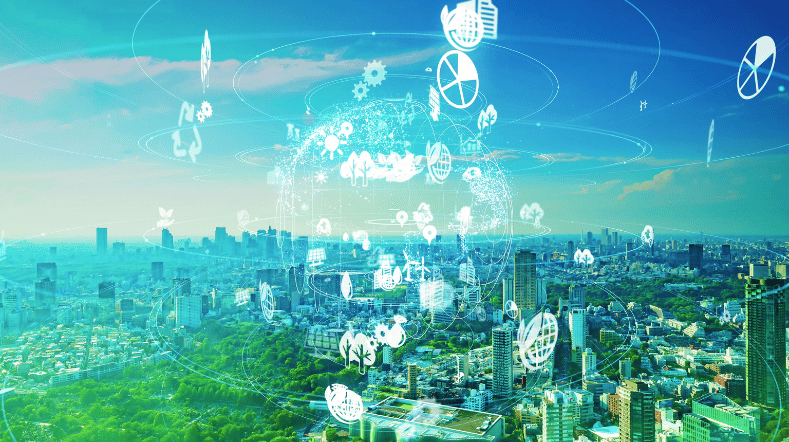
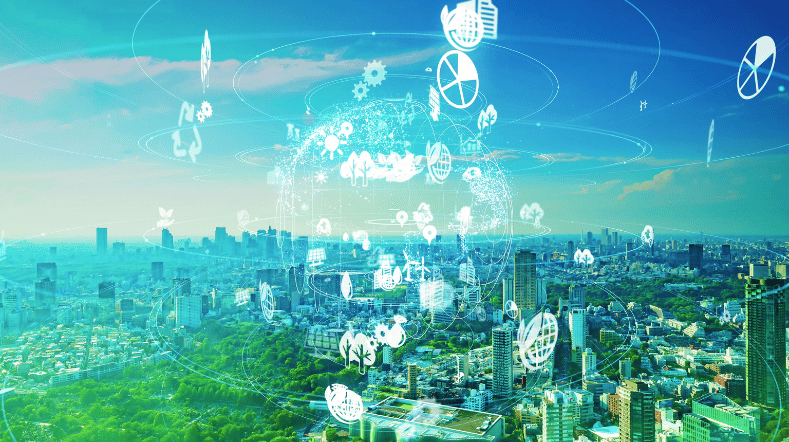
The Netherlands starts realisation GPT-NL, its own open AI-language model
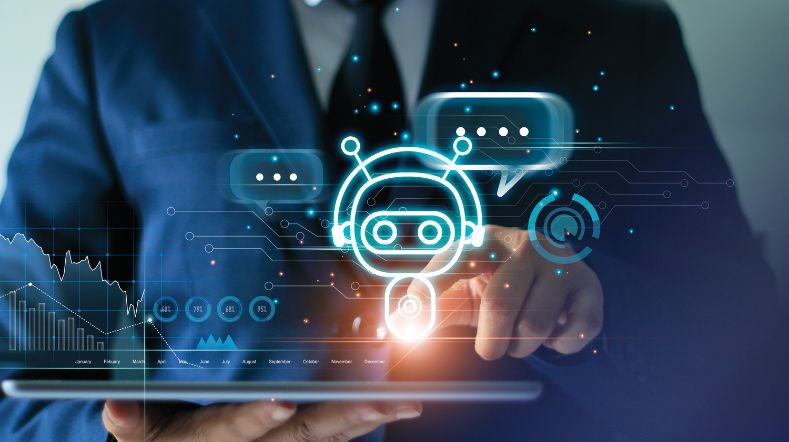
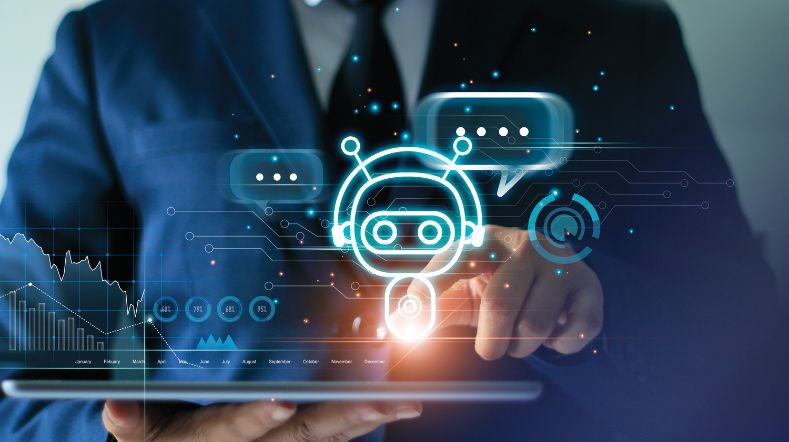
New AI Lab for effective and responsible oversight
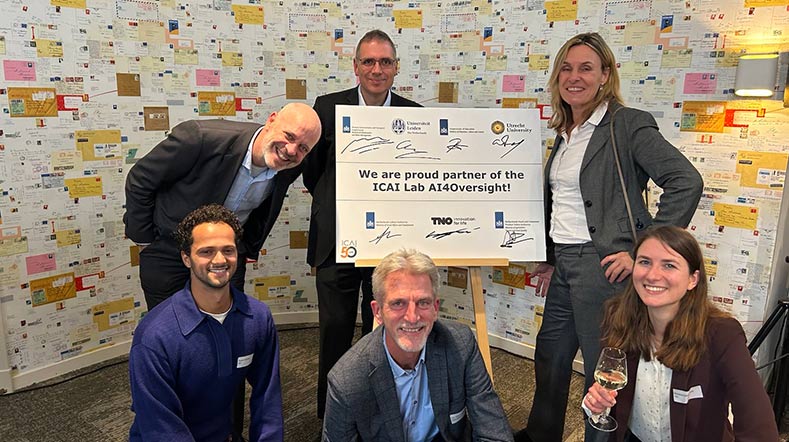
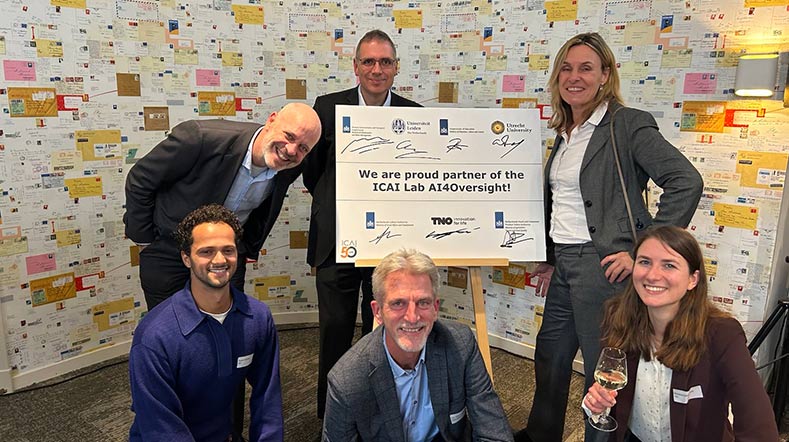
First overview of cyberattack techniques by AI against AI
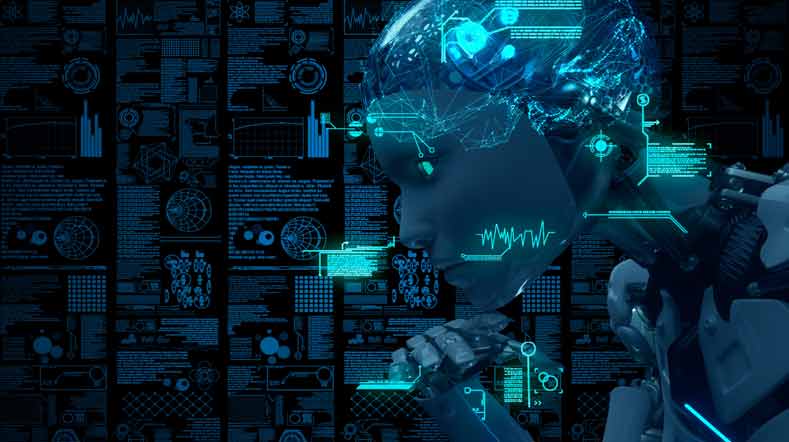
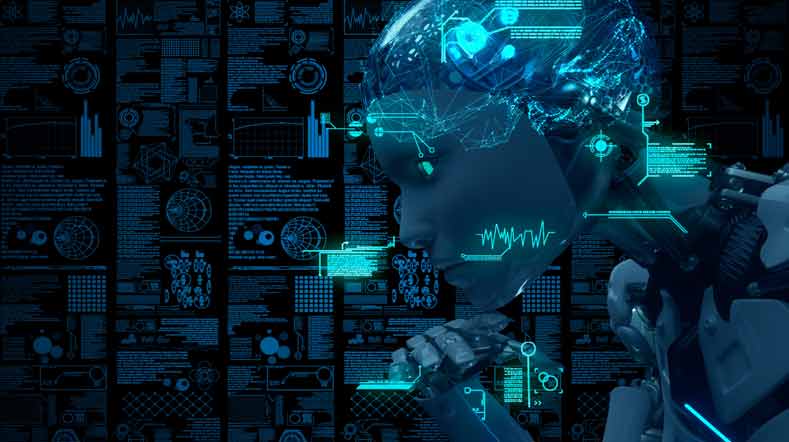