Poverty reduction with privacy technology: the 13 most pressing questions
The number of Dutch citizens living below the poverty line is continuing to rise. According to calculations by the CPB, 5.8% of the population will be below the poverty line by 2024. To address poverty in a targeted way, help must reach the right people. Technology can help with that. TNO is collaborating with the Dutch government on technologies that can contribute to poverty reduction in a responsible and safe way. We will explain exactly how that works.
Freek Bomhof, senior consultant at TNO, specialises in responsible data sharing. He will answer 13 frequently asked questions on poverty reduction using technology.
To properly help citizens, the government must first get a good picture of people with financial problems. That insight comes from being able to combine data from multiple agencies.
Technology can help with that. Using technology, we can gain insights from data without having to see the data ourselves. This allows us to actively help people without violating their privacy.
We know this by combining insights from multiple data sources. If someone only has a health insurance debt, this does not necessarily cause immediate problems. But when the utility bills can no longer be paid and debts start piling up, help is desirable. If someone has debts with multiple agencies and a low income, that person probably needs help.
If it’s not allowed, it won’t happen. The privacy law, the GDPR, is very clear about that. There must always be a so-called ‘legal ground’ for sharing personal data.
But even if there is such a legal ground, data sharing should be done with great care. After all, we are dealing with potentially highly sensitive personal data. Privacy-enhancing technologies (PETs) ensure that no more can be done with the data than is legally permissible.
Selling data illegally, ‘just looking into’ the personal data of a well-known Dutch citizen, or doing some additional analysis that has nothing to do with fighting poverty? These are all impossible when using PETs.
By using privacy-enhancing technologies. Using this technology, the agencies do not share data in a readable form. They only share the results of a pre-agreed joint calculation on those data. By combining and enriching the results of such calculations from different organisations, new insights emerge. In this way, insight is gained into who needs help.
Yes, and not only that; they also appear to work incredibly well. We saw this, for example, in a pilot we conducted with the UWV and SVB to help people who do not receive a full state pension.
Moreover, these are proven technologies that are already being used in many areas, not just to reduce poverty.
These are privacy-enhancing technologies (PETs), a collective name for various technologies that allow organisations to use each other’s data without violating people’s privacy.
These include, for example:
- Multi-party computation (MPC);
- Federated learning (FL);
- Synthetic data generation
We will explain these technologies below.
Multi-Party Computation
You can think of MPC as a digital ‘toolbox’, full of cryptographic techniques. These encryption techniques allow multiple parties to jointly compute data, as if they had a shared database. Because of this encryption, parties can never see each other’s data.
> This video explains exactly how MPC works.
Federated Learning
When organisations conduct collaborative research using machine learning, there is usually one central database where all the data are stored.
Thanks to federated learning, this is no longer necessary, meaning data can no longer leak. The data are not sent to the ‘machine learning’ model for computation. Instead, federated learning brings the model to the data.
Training the models is thus broken down into partial calculations that are performed locally at an organisation. Afterwards, only the insights ‘learned’ by the model are shared with other organisations, not the privacy-sensitive data themselves.
Synthetic data
Synthetic data are generated by first creating a model from personal data, which are then used to generate new, simulated data.
As such, data are used that can no longer be traced back to an individual. This is particularly useful for the testing and setting up of systems. With synthetic data, you don’t need the original, sensitive personal data to still be able to determine whether your data analysis is working properly and reliably.
The foundation of PETs is cryptography (i.e. encryption). This technique has been used to protect communications for decades. Think of the ‘lock’ in your internet browser telling you that your data are encrypted. PETs use a special form of encryption that cannot be broken (which can be mathematically demonstrated).
It’s true that you can apply these technologies in many more areas, not just to reduce poverty. In fact, you can use them anywhere where personal data are shared. However, data sharing occurs in very many places within the government, and it takes time to apply PETs everywhere. It is also sometimes challenging to design the right form of encryption. Several PETs are already being successfully used by organisations, but sometimes more research is needed.
No. Even if personal data are encrypted, they are still personal data. If something is not allowed by the GDPR, then it will also be prohibited using PETs. As such, it is not some kind of loophole. Conversely, if data processing is permitted, PETs will ensure optimal compliance with the GDPR.
The original data always remain with the various organisations. If there is a reason to check whether the processing has occurred properly, you can always return to the source. The difference with the standard way of working is that copies of the data are no longer stored everywhere, making inconsistencies less likely to occur.
PETs are techniques that can be employed in any data application. If desired, one could also misuse these techniques. For instance, by selecting individuals based on discriminatory suspicions of fraud, as occurred during the benefits scandal. PETs, therefore, cannot prevent a situation like the benefits scandal.
However, the process of implementing PETs might potentially raise concerns earlier. Because their implementation always involves more in-depth technical analyses. This includes a thorough evaluation of information leaks and careful consideration of whether the obtained information can be used in an ethically responsible manner. Thus, the process incorporates numerous checkpoints.
Developments have been very rapid. A few years ago, the technology was not practically applicable, but now, very many of the applications are operationally available.
In the Netherlands, there are several companies, such as Roseman Labs and Linksight, that offer ready-to-use PETs.
PETs are not yet well suited for different types of more complex data. There is also a lot of work still to be done on standardisation to allow PETs to be more easily deployed more widely. That wider use is really needed: The Netherlands is selling itself short by not optimally using and protecting sensitive data.
Get inspired
HERACLES lays foundation for secure data sharing in healthcare sector
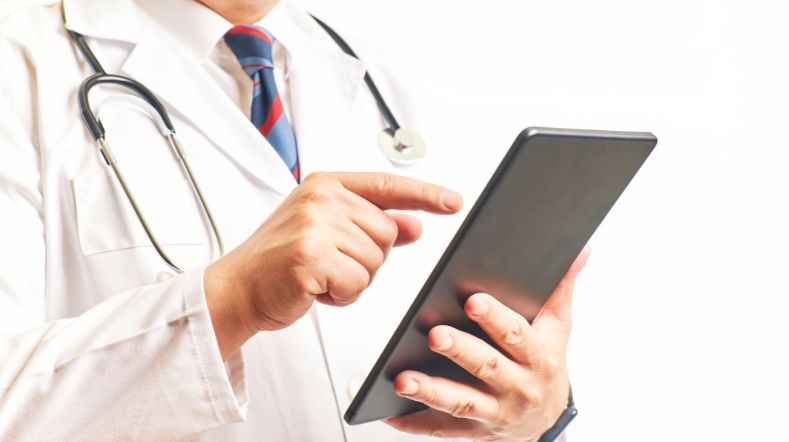
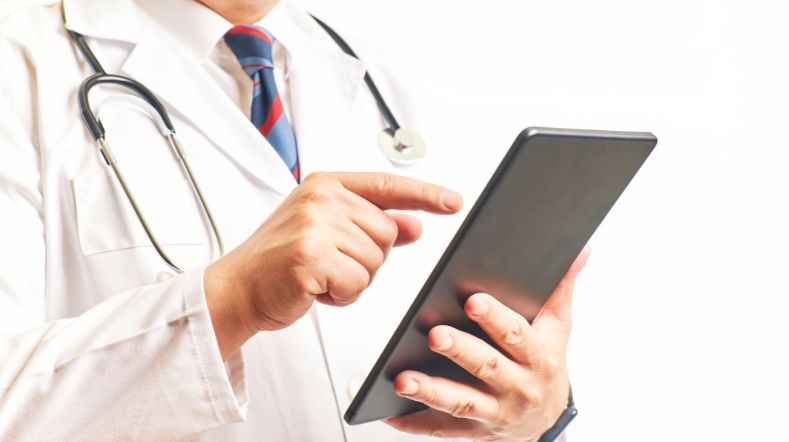
Authorities learn with NICPET to seize opportunities of secure data sharing
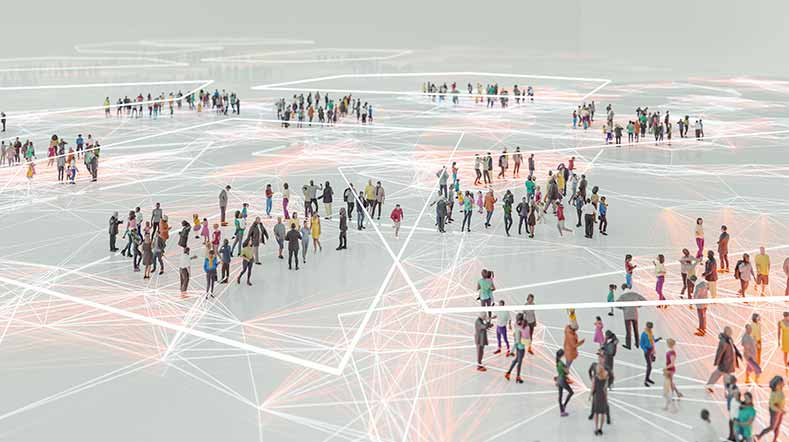
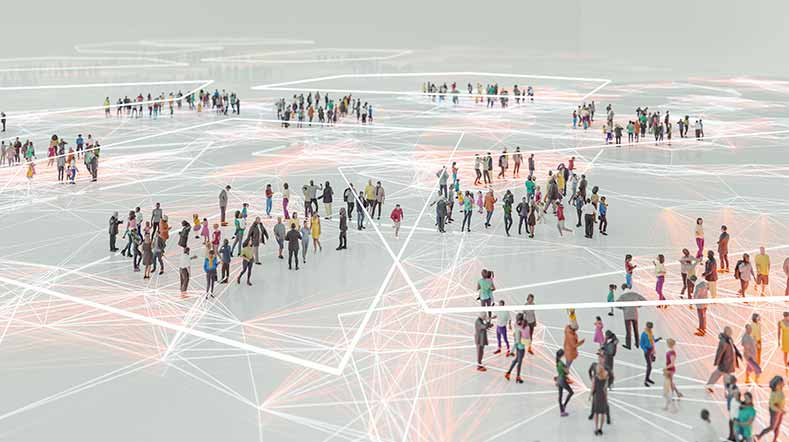
Poverty data insights
Technological and legal innovation essential to improve government services
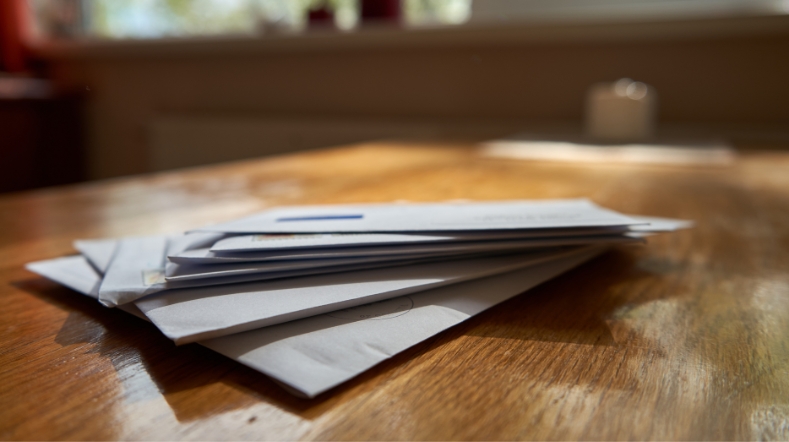
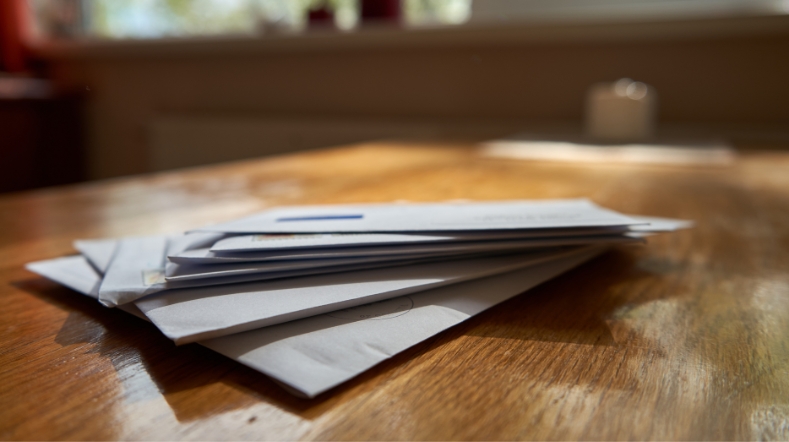
Reducing energy poverty in a targeted way thanks to Privacy-Enhancing Technologies
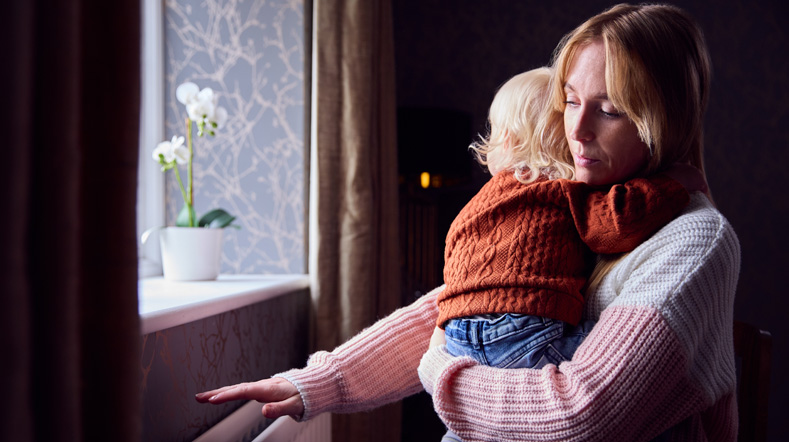
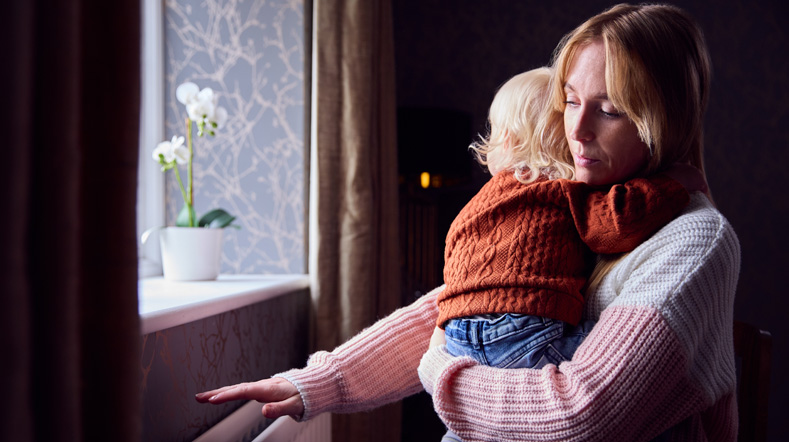