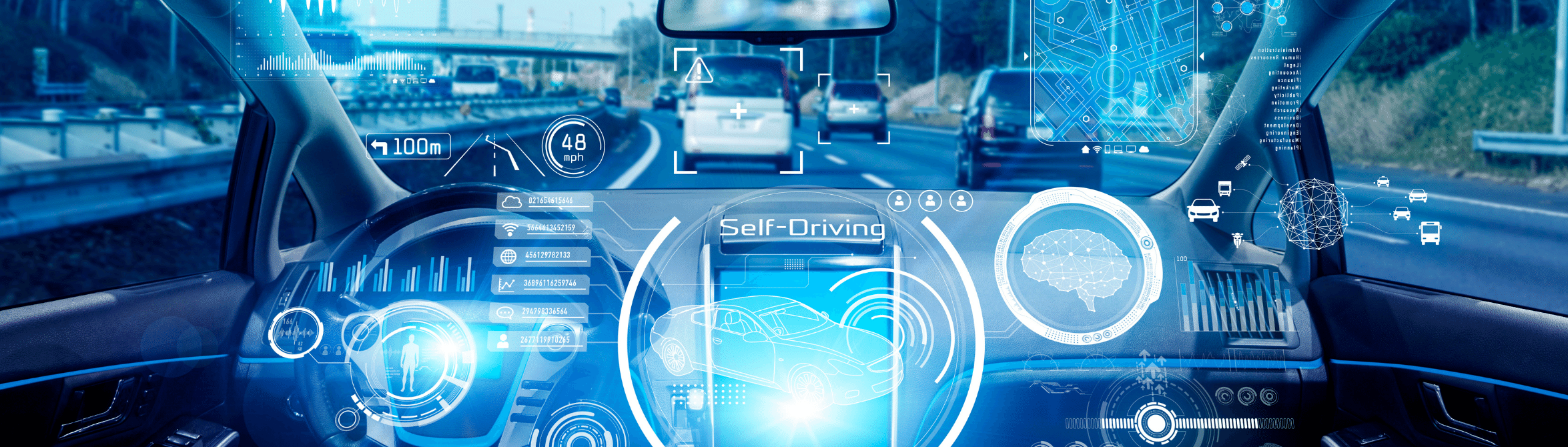
Self-learning adaptive systems accelerate the industrial development process
Whether concerning the effectiveness of batteries in electric vehicles or the reliability of high-speed data communication, unforeseen circumstances can significantly disrupt performance. This is why we’re diligently looking for better ways to respond to these ever-changing circumstances. Self-learning algorithms, able to link existing knowledge to huge amounts of collected data, can play a crucial role in this. Within the context of the AutoAdapt research programme, TNO is investigating the potential of this promising, innovative technology.
Multidisciplinary research
Frank Willems, professor at the Eindhoven University of Technology (TU/e) and principal scientist in TNO’s Traffic & Transport unit, and Gert Witvoet, associate professor at the TU/e and senior specialist in TNO’s Industry unit, got talking eighteen months ago. Willems: ‘I was just setting up the AutoAdapt research programme (pdf). We turned out to have more in common than we thought. This, being involved in so many different disciplines at the same time, is one of the great aspects of working for TNO. This multidisciplinary research programme, for example, is made up of colleagues from no fewer than five units: Traffic & Transport, where I work, Industry, to which Gert is attached, as well as the ICT, Energy Transition, and Defence units. All these people bring their own expertise to the table.’
'The potential of self-learning systems is enormous, a fact that’s especially true for complex systems.'
Self-learning systems
According to Willems, the goal of the research being done in the AutoAdapt programme is to combine existing knowledge with data. ‘To this end, you can ask questions like, “How will a vehicle behave ten years from now?” “How will it be used during this period?” “Will it still be possible to guarantee the lowest operating costs despite widely varying usages?” To answer these and other questions, you’d currently have to do an awful lot of laboratory testing.’ According to Willems, adaptive systems make it possible to use available data to make accurate predictions about all kinds of circumstances, as well as anticipate their occurrence. ‘Monitoring the course of real-life performance in combination with the effects of previous interventions allows these adaptive algorithms to learn. Such algorithms drastically reduce development and testing times, making it possible for new products to find their way to market much faster. The potential of self-learning systems is enormous, a fact that’s especially true for complex systems. But there is also a huge gap between the situation in the lab and their industrial application. AutoAdapt intends to bridge that gap.’
Demonstrating in practice
In the coming years, we aim to demonstrate in practice how self-learning adaptive methods, which link existing knowledge to high-quality data, can be used to accelerate innovation. One such promising innovative technology is that of optical satellite communication, which uses laser beams to transfer huge amounts of data. Witvoet: ‘Self-learning algorithms are used to determine the configuration of the laser beam, ensuring optimal performance; in other words, high speeds that don’t sacrifice the connection’s stability or availability.’ Concerning vehicles – electric and hybrid lorries in particular, the focal point of Willems’ research – self-learning algorithms ensure proper decision-making. Such decisions may relate to charging times and battery charging profiles for plug-in hybrid and electric lorries, but may also concern their routes. Willems: ‘The last thing you want is for a vehicle to suddenly stop due to an empty battery. At the same time, you don’t want the battery to depreciate too quickly either. Using self-learning algorithms, in future, vehicles will be able to make informed decisions about the right times to stop and recharge. In this, a lot of data can be uploaded into the cloud, as Tesla is already doing.’
'One of the greatest challenges related to self-learning adaptive systems concerns determining the appropriate interventions needed to continue achieving optimal performance, despite risk factors.'
Risk factors
Willems’ and Witvoet’s research areas intersect in the area of dealing with unforeseen operational circumstances. Willems: ‘Consider, for example, changing weather conditions or vehicle traffic flows. One of the greatest challenges related to self-learning adaptive systems concerns determining the appropriate interventions needed to continue achieving optimal performance, despite risk factors.’ Battery life, for example, is highly dependent on usage and charging profiles. At the same time, hot or cold weather, driving behaviour, or the selected route chosen can significantly impact the energy demand. Despite such variations, we want to maximise the uptime of lorries or buses to allow for as much cargo or as many people as possible to be transported. According to Witvoet, internal and external factors (such as turbulence in the atmosphere and satellite vibrations, as well as the properties and state of individual transmitters and receivers) can also disturb the data line of optical satellite communication. ‘Vibrations can affect the precision of the beam. Narrower beams result in faster data traffic, but are also more sensitive to interference. Broader beams, on the other hand, are more stable, but this comes at the cost of slower data-transferring speeds.’
Adaptibility
How can you replace the human programmer at the controls, optimally configuring everything, with self-learning, adaptive systems that use existing knowledge and data to adapt to the desired situation? Willems: ‘You could equip vehicles with an operating system able to make all kinds of decisions using self-learning, adaptive algorithms. There are a lot of benefits to this. Consider an increase in traffic safety and optimal battery usage, for example. And, concerning hybrid vehicles, better usage of combustion engines – with all the positive consequences that this entails, such as lower nitrogen and CO2 emissions. In the run-up to 2030, we need to move towards zero-emission inner cities. The smart use of route and traffic-flow data, as well data about the state of their battery, will mean that this reality will also be within arm’s reach for hybrid vehicles.’ Witvoet is also highly optimistic about the possibilities of self-learning algorithms. ‘Equipping transmitters and receivers in optical satellite communication with self-adaptive capabilities allows them to adapt their configuration to changing circumstances. In doing so, we can always get the best from the optical links, thereby guaranteeing the optimal and constant flow of data.’
Early Research Programme: from research to innovation
The AutoAdapt programme is a so-called Early Research Programme, in which research is taking place beyond the borders of different disciplines, Willems explains. More importantly, the research being done in the programme is usually way ahead of an innovation’s application. ‘That doesn’t mean we’re just a bunch of researchers sitting at our desks in a room together; the research we’re doing may be at an early stage, we nonetheless have the express intention for it to be applied in industry.’
Dot on the horizon
As far as Willems is concerned, that is also the ultimate dot on the horizon. ‘We’d like our insights to generate results that can be demonstrated in practice using demos. To this end, we like talking to industrial partners with relevant user cases. We want to show the results of our research in ‘real-world’ situations. We, for example, already have a small demo installation on the automotive campus in Helmond, where we can demonstrate real-world battery performance – albeit in a partially virtual environment using a virtual vehicle.’ The researchers like to think big, however. Witvoet: ‘The insights we’re generating through AutoAdapt are also expected to lead to huge savings in terms of production costs and times. This could, for example, include less strict material or production-tolerance requirements. By putting our innovations into practice together with industrial partners, we’re hoping to reduce costs.’
Want to know more or cooperate?
We’d like to get in touch with parties with similar ambitions. Together, we’re committed to developing and offering the most innovative and advanced self-learning adaptive systems. To find out more, we invite you to get in touch with TNO’s Frank Willems.