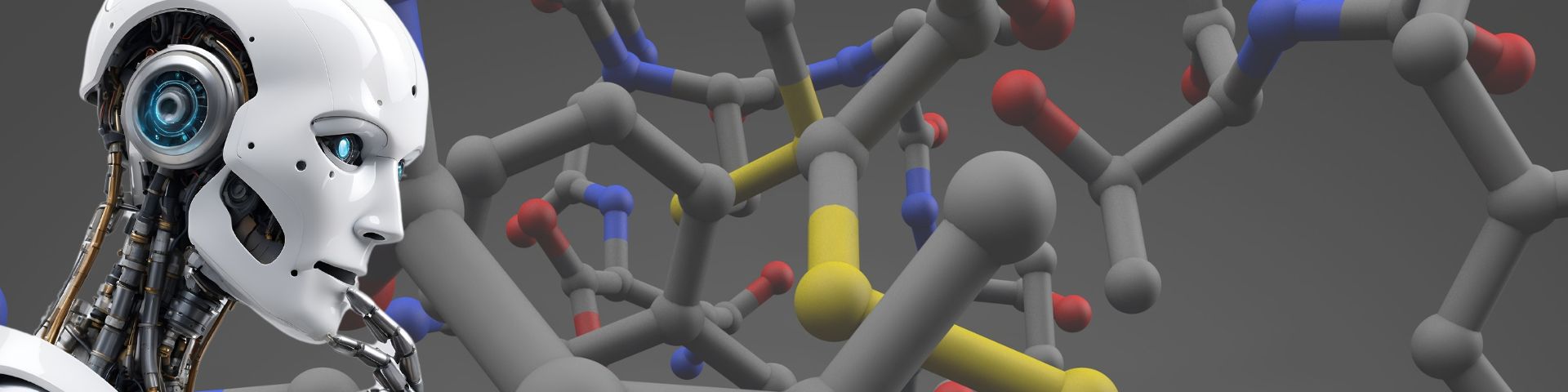
Biopolymer design by machine learning
We are talking about the year 2050. Picture a sustainable society with a sustainable chemical industry delivering sustainable materials. Where waste is a resource and biomass and CO2 are used to replenish those materials that cannot be kept in the loop.
A key question to answer in the years to come: Will we be using today’s plastic materials, derived from fossil resources, or can we reap the benefits from the materials that can be obtained from biomass?
Unlocking sustainable materials
Of course, there is no silver bullet. For some applications, today’s plastics are best suited and efficient pathways need to be developed for sustainable resources like biomass or CO2. For other applications, novel plastics might outperform today’s plastic materials and we will need to scale up technology and achieve full-scale market introduction.
But how do we find these outperforming plastics (i.e. polymers with/without additives)? There are so many options, whilst the current materials portfolio has been developed over the last 100 years, mostly based on empirical research on structures, properties, applications and processes. There is no time for a similar time-consuming exercise for CO2- or biobased polymers: 2050 is only 1 investment cycle away.
Our proposed solution: data science meets material science
This is where data science meets material science. With unprecedented computing power, novel machine learning algorithms have been developed over the past decade for various domains. Also for material science. Machine learning has large potential for the prediction of properties of new polymers and for the design of new polymers with desired properties.
Data-driven material innovation: promise & challenges
Hence, the promise of machine learning is to accelerate material design processes, saving both time and money. With machine learning, it is possible to design for functionality, sustainability and appropriate end of life (e.g. recyclability or biodegradation) simultaneously.
To unlock the potential and realise the promise, several challenges need to be addressed in parallel:
- Data science requires large amounts of data to deliver accurate models, whilst data on polymers and their properties is scarce and fragmented;
- Accurate models require machine learning algorithms that represent polymers adequately, whilst current algorithms use oversimplified polymer representations (so-called fingerprints), lacking essential polymer properties, the role of additives and the influence of processing history;
- Novel materials should be safe & sustainable by design, with appropriate end-of-life options, whilst current machine learning models only design on the basis of functionality.
PolySCOUT programme: advancing biopolymers
In 2022, TNO initiated a programme called polySCOUT, sustainable polymer design by machine learning.
The vision: polySCOUT seeks to unlock the potential of data-driven material innovation. By synergising knowledge from different fields, it aims to revolutionise how we design and create novel (polymer) materials.
The approach: research groups of TNO are uniting their expertise in data and material science to create predictive models. This collaborative effort aims to tackle multifaceted challenges across various research domains. (see picture below).
The tangible outcomes: polySCOUT doesn’t stop at theoretical advancements. Along its journey, it produces tangible results—enhanced models and novel materials and empowers collaboration with external partners. The experimentally validated models pave the way for designing materials that align with industrial requirements.
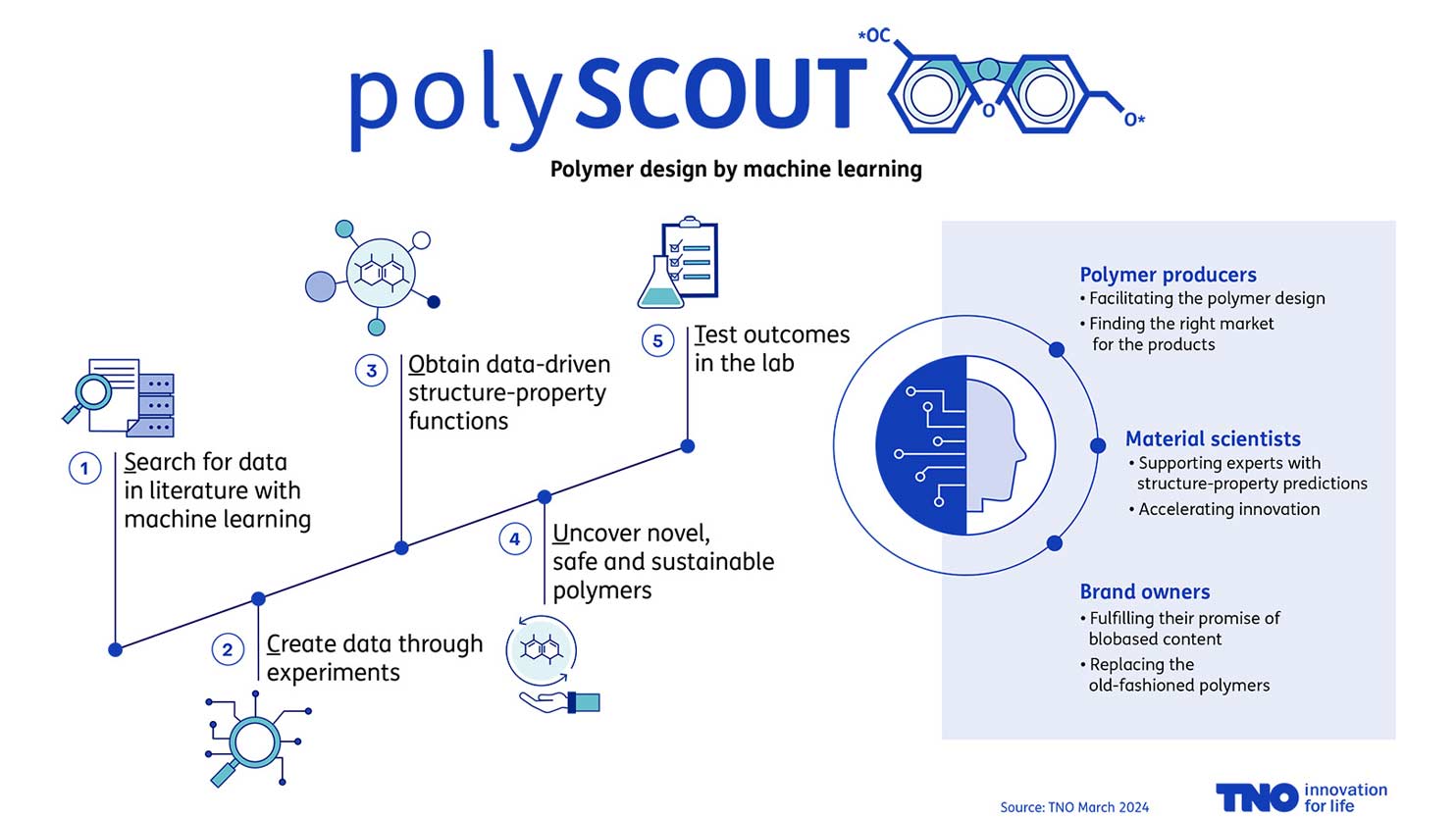
We create novel and/or improved safe and sustainable polymers. Based on a comprehensive, high-quality polymer database, developed with validated machine learning methods and extensively tested in the lab. To fulfil the needs of polymer producers, material scientists and brand owners.
Example of collaboration
A nice example of such a collaboration is the JTF-project BIOTTEK. Here Senbis is aiming to develop a biodegradable polyester as textile fibre. This novel product potentially mitigates a major source of microplastics in marine environments. In the project, TNO is further developing the machine learning algorithms and models to assist Senbis and RUG/NHL-Stenden with their polymer development.
We invite industry to co-develop and validate our model for its application domains of interest. In this way, you will obtain leads for novel polymers that match requirements.
Get inspired
Biobased materials
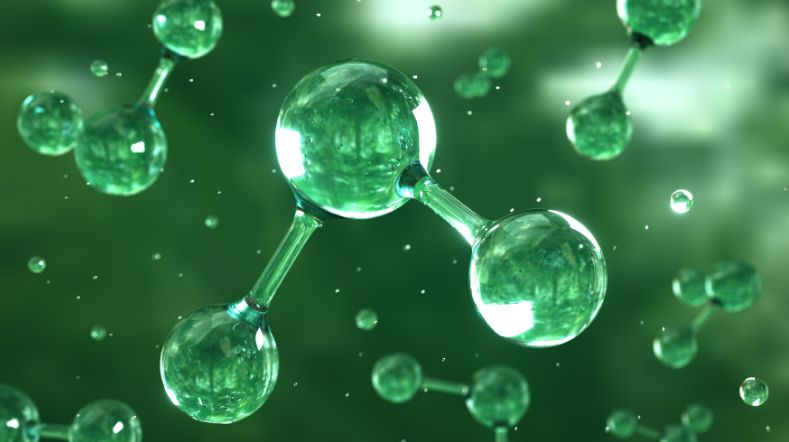
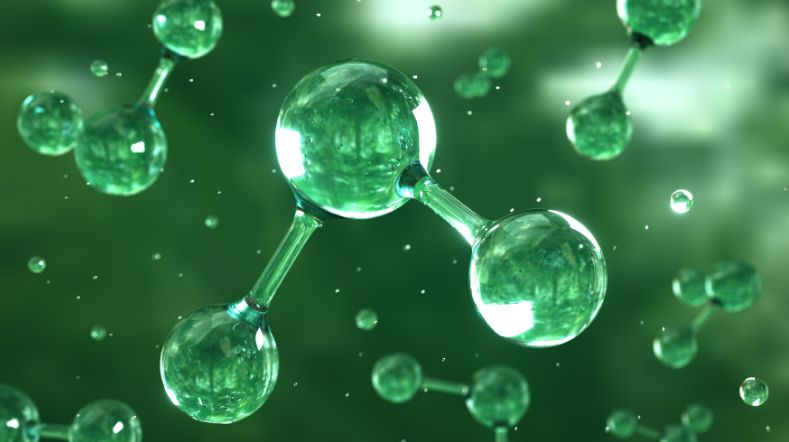
Enhanced solvolysis contributes to the future of composite recycling
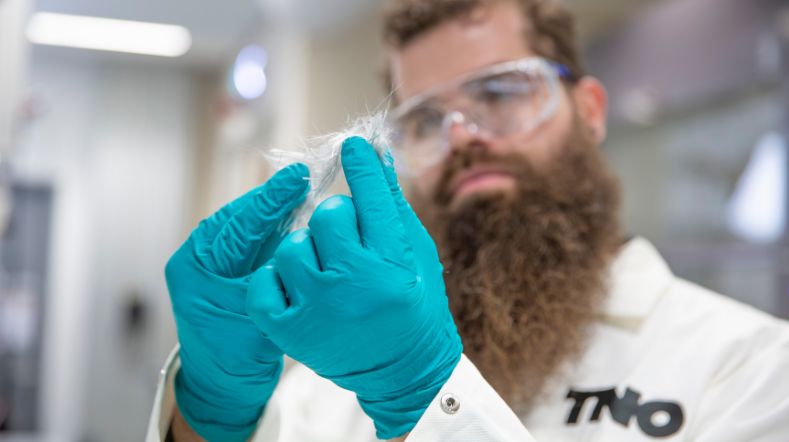
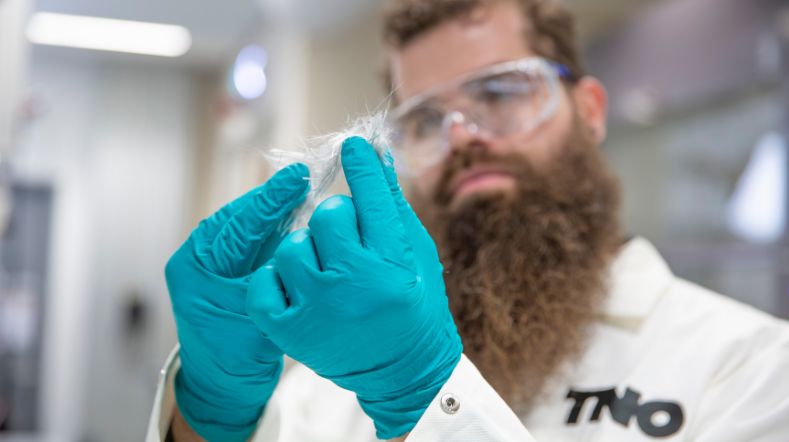
Collaboration for sustainability: better results through joint innovation
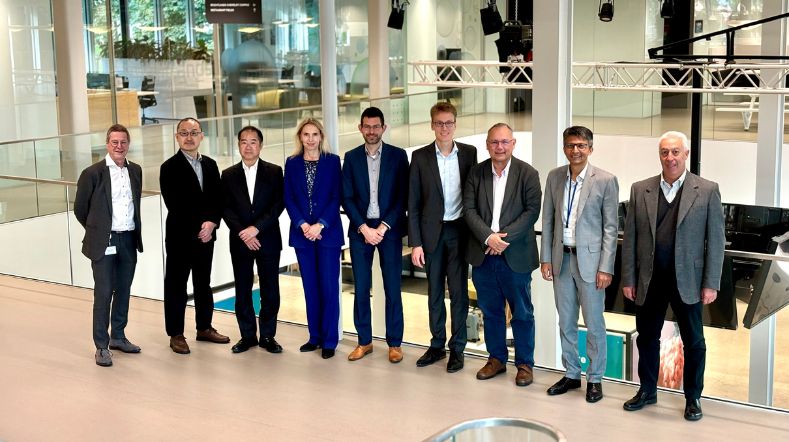
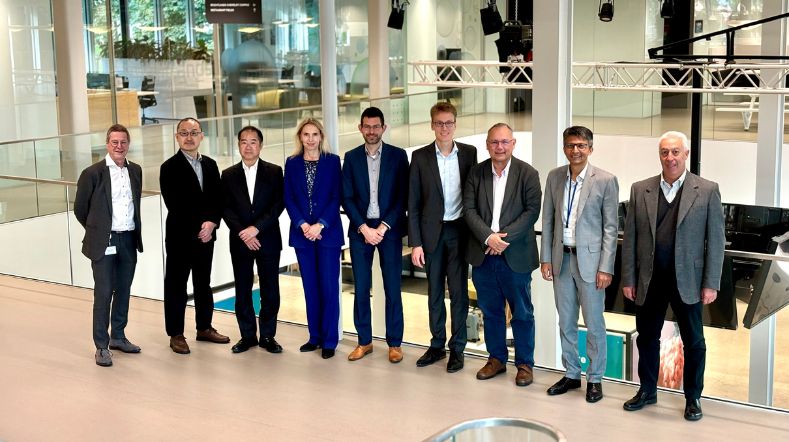
Webinar: Biobased plastics in a sustainable future
Biobased plastics
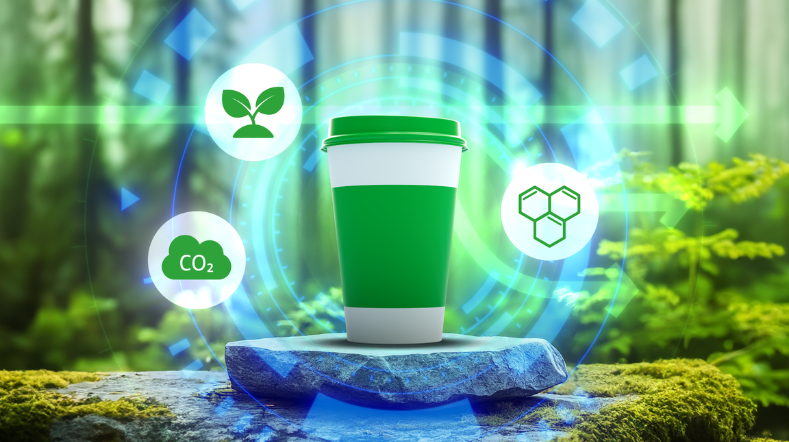
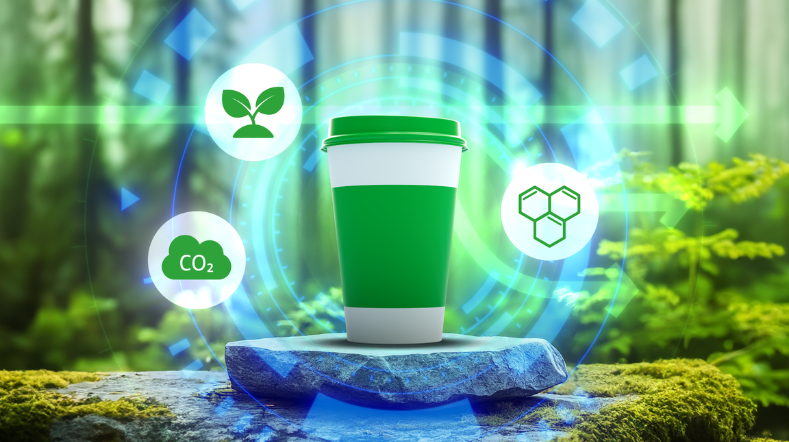