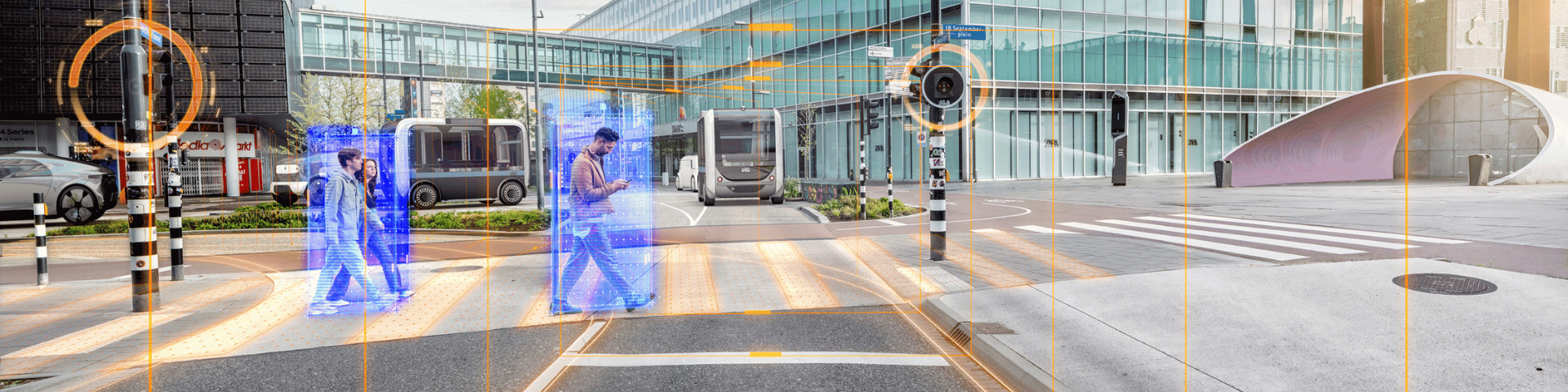
StreetWise: scenario based safety assessment for automated driving
In cooperation with
Siemens, AVL, Daimler Truck
With ever more advanced Automated Driving Functions (ADFs), safety validation becomes more important and complex. ADF needs to be tested in a high number of possible scenarios. Therefore virtual simulation – in addition to proving-ground and field testing – is essential to discover if safety requirements are met with reasonable efforts and throughput time. TNO StreetWise uses data representative for real-world scenarios.
To optimally calculate the safety risks, virtual simulations need to be based on realistic test cases and characteristics. TNO StreetWise uses public road driving data as input for scenario identification and storage, and test cases are based on such scenarios. Thanks to this method the virtual test cases are representative for real-world scenarios. To ensure a safe implementation of Automated Driving (AD) technologies and to achieve a positive risk balance, TNO offers innovative risk quantification methods and scenario statistics.
Advanced safety methodology
Our Safe & Social programme consists of automated systems to exhibit 'responsible, predictable, and conflict-avoiding behaviour. Where this programme aims at adopting driving behaviour that contributes to road safety, the innovation programme StreetWise focuses on preventing unsafe situations that could lead to harm people. Based on TNO’s extensive experience with vehicle safety assessment, the TNO StreetWise methodology addresses operational safety. This is a translation of functional safety (ISO26262) and safety of the intended functionality (ISO/DIS 21448:2021 SOTIF). [2] [4] [7].
After all, the automation technology takes over tasks from the human driver and should be prepared for all situations in the operational domain designed for (ODD). This advanced methodology is substantiated by a series of scientific articles.
Safer automated driving
Challenges for the tested systems are determined and quantified by combining systematic descriptions of the vehicle’s surroundings in scenarios, with systematic (statistical) analysis of the vehicle behaviour in such scenarios. By using statistical data from the exposure to different scenarios, we can quantify risk based on these simulations [2] [5].
By doing so, our industrial clients can make significant steps towards safer automated driving. Authorities responsible for type approval can use TNO StreetWise’s quantified risk estimation to determine the safety of a vehicle before allowing it for deployment onto the public road.
Efficient and flexible pipeline
The TNO StreetWise pipeline automatically processes your driving data into activities, scenarios, parameters and statistics. New types of scenarios can be added quickly using the underlying framework. Python algorithms, running in a cloud environment, ensure flexibility and scalability The pipeline is ready for connected vehicles as well (V2X, I2V).
A webGUI provides easy access to the scenario database for extraction of OpenSCENARIO test cases, including the related Euro NCAP tests. Test automation is supported through an API, as successfully demonstrated with AVL ModelConnect, Vires VTD and Siemens Simcenter PreScan. Sensitive driving data can be processed at the client’s premises.
Use of AI
TNO is working on a system that can reason based on knowledge, rather than just data. We want to use AI in a way that remains explainable. Chris van der Ploeg’s research into the use of AI helps make vehicles safer. Some self-driving cars currently interpret waving flags as flashing traffic lights. This poses an obvious safety risk and is an example of an issue to be detected during safety assessment. For such cases StreetWise surfaces the relevant information from data supporting the designers of AI systems to understand interaction between the system and the environment.
CCAM community: Valuable network
Our clients benefit from the extensive TNO network of partners. For example, we are champion in a certified Euro NCAP test body, and working on the development of the Cyclist-AEB test protocol (CATS). TNO is also working on the way how Euro NCAP can use scenario databases.
The list of partners in B2B and EU projects includes most of the CCAM community (e.g., SAKURA [1], NTU CETRAN (Singapore), AVL [9], Siemens, Itility, Magna [9], IDIADA [1], BMW [7], Volvo [7], Continental [7], ika [1] [7], Virtual Vehicle [9], and VEDECOM).
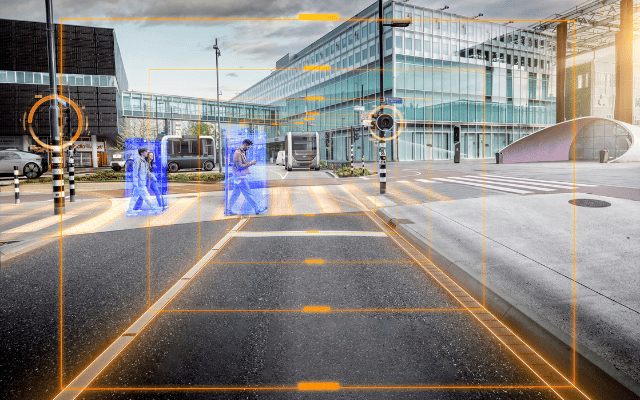
Download the paper
The paper Scenario-Based Safety Assessment of Automated Driving Systems is essential for anyone involved in scenario-based safety assessment or seeking to gain insights into its evaluation process.
More about this project
-
De Gelder, E., Op den Camp, O. "Scenario-based assessment of automated driving systems: How (not) to parameterize scenarios?", 2024. https://arxiv.org/pdf/2409.01117
-
De Gelder, E., Buermann, M., Op den Camp, O. "Coverage Metrics for a Scenario Database for the Scenario-Based Assessment of Automated Driving Systems", 2024. https://arxiv.org/pdf/2409.01139
- De Gelder, E., Paardekooper, J.-P., Khabbaz Saberi, A., Elrofai, H., Op den Camp, O., Kraines, S., Ploeg, J., De Schutter, B., "Towards an Ontology for Scenario Definition for the Assessment of Automated Vehicles: An Object-Oriented Framework," in IEEE Transactions on Intelligent Vehicles, vol. 7, no. 2, pp. 300-314, June 2022, DOI: 10.1109/TIV.2022.3144803.
- Op den Camp, O., van de Sluis, J., de Gelder, E., and Yalcinkaya, I., “Generation of Tests for Safety Assessment of V2V Platooning Trucks”, in 27th ITS World Congress, Hamburg, 2021.
- Van de Sluis, J., Op den Camp, O., Broos, J., Yalcinkaya, I., and de Gelder, E., “Describing I2V Communication in Scenarios for Simulation-Based Safety Assessment of Truck Platooning.” Electronics 10, 1 (2021), https://doi.org/10.3390/electronics10192362.
-
Zlocki, A., Op den Camp, O., Arrúe, Á., Taniguchi, S., Umeda, M., Watanabe, S., and Antona-Makoshi, J., “Towards the Harmonization of Safety Assessment Methods of Automated Driving: SAKURA – SIP-adus - HEADSTART, White Paper” December 9, 2021.
-
De Gelder, E., Elrofai, H., Khabbaz Saberi, A., Op den Camp, O., Paardekooper, J.-P., and De Schutter, B., “Risk Quantification for Automated Driving Systems in Real-World Driving Scenarios”, IEEE Access 9, vol. 9, pp. 168953-168970, 2021, DOI: 10.1109/ACCESS.2021.3136585.
- “ISO/TR 21934-1:2021 Road Vehicles — Prospective Safety Performance Assessment of Pre-Crash Technology by Virtual Simulation — Part 1: State-of-the-Art and General Method Overview.” https://www.iso.org/cms/render/live/en/sites/isoorg/contents/data/standard/07/64/76497.html
- De Gelder, E., Manders, J., Grappiolo, C., Paardekooper, J.-P., Op den Camp, O., and De Schutter, B., "Real-World Scenario Mining for the Assessment of Automated Vehicles," 2020 IEEE 23rd International Conference on Intelligent Transportation Systems (ITSC), 2020, pp. 1-8, DOI: 10.1109/ITSC45102.2020.9294652.
-
De Gelder, E., Op den Camp, O., and de Boer, N., “Scenario Categories for the Assessment of Automated Vehicles – version 1.7”, Technical Report, Nanyang Technological University, Singapore: CETRAN, 2020. http://cetran.sg/wp-content/uploads/2020/01/REP200121_Scenario_Categories_v1.7.pdf.
-
Kalisvaart, S., Slavik, Z., and Op den Camp, O., “Using Scenarios in Safety Validation of Automated Systems.” In: Leitner, A., Watzenig, D., Ibanez-Guzman, J. (eds) Validation and Verification of Automated Systems. Springer, 2020, Cham. https://www.springer.com/gp/myspringer/chapters.
-
Paardekooper, J.-P., van Montfort, S., Manders, J., Goos, J., de Gelder, E., Op den Camp, O., Bracquemond, A., and Thiolon, G., “Automatic Identification of Critical Scenarios in a Public Dataset of 6000 km of Public-Road Driving”, in 26th International Technical Conference on the Enhanced Safety of Vehicles (ESV), Eindhoven, 2019.
-
Elrofai, H., Paardekooper, J.-P., de Gelder, E., Kalisvaart, S., and Op den Camp, O., “Scenario-Based Safety Validation of Connected and Automated Driving.”, Vision Paper TNO, 2018.
https://publications.tno.nl/publication/34626550/AyT8Zc/TNO-2018-streetwise.pdf (pdf) . - Op den Camp, O., van Montfort, S., Uittenbogaard, J., and Welten, J., “Cyclist Target and Test Setup for Evaluation of Cyclist-Autonomous Emergency Braking”, in International Journal of Automotive Technology, Vol. 18, No. 6, pp. 1085-1097, 2017. DOI: 10.1007/s12239−017−0106−5
- EU H2020 Hi-Drive (2021-2025): Large-scale pilot for prolonged automation without interruptions (continuous ODD). Data analysis, edge cases, simulation.
- TKI StreetWise Plus (2021-2022): Extension of scenario database for multi-actor simulation, I2V messages and roadside data sources. With Siemens and Itility.
- EU H2020 ARCADE (2018-2022): Coordination and support project for CCAM projects. Stakeholder workshops on common evaluation methodology (Nov 2020) and edge cases (May 2021).
- EU H2020 Headstart (2018-2021): Scenario-based safety assessment methodology including V2X, localisation and cyber security. Detailed methods for scenario selection and allocation to test methods. Use case truck platooning.
- TNO PUMAS (2018-2020): Multi-partner scenario database project with AVL, Magna, Siemens and a Japanese OEM. Proof of concept of sharing scenarios from diverse driving datasets for 1,000 hours of data. Including completeness metrics.
- EU H2020 L3Pilot (2017-2021): Large-scale pilot for L3 automation. Data analysis and impact assessment for Jaguar Land Rover and Aptiv. Evaluation of lane-change detection.
- EU ECSEL ENABLE-S3 (2016-2019): Virtual verification and validation of automated systems. Methodology, scenario working group, valet parking use case, image-guided surgery use case, dataset inventory.
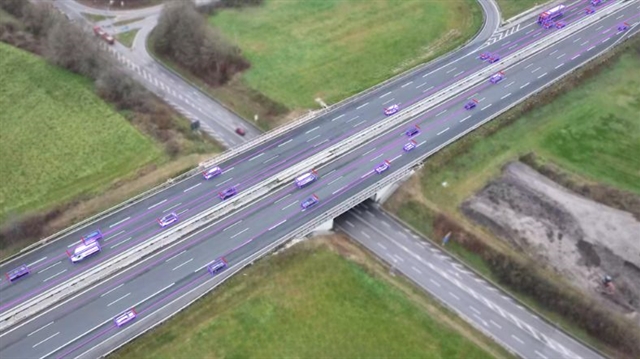
Releasing autonomous software faster with DeepScenario and TNO’s StreetWise
Traditional data collection using prototypes is costly and slow, and analysing the data demands advanced tools. To solve this, DeepScenario and TNO offer a data-driven solution already used by Torc Robotics and other automotive companies.
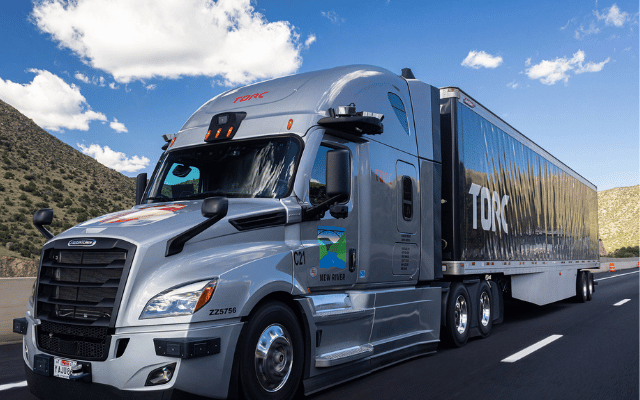
TNO and Torc Robotics collaborate to use real-world data for autonomous truck validation
Torc Robotics, an independent subsidiary of Daimler Truck and TNO are working together to substantiate the safety of self-driving trucks using scenario-based safety validation.
Get inspired
Releasing autonomous software faster with DeepScenario and TNO’s StreetWise
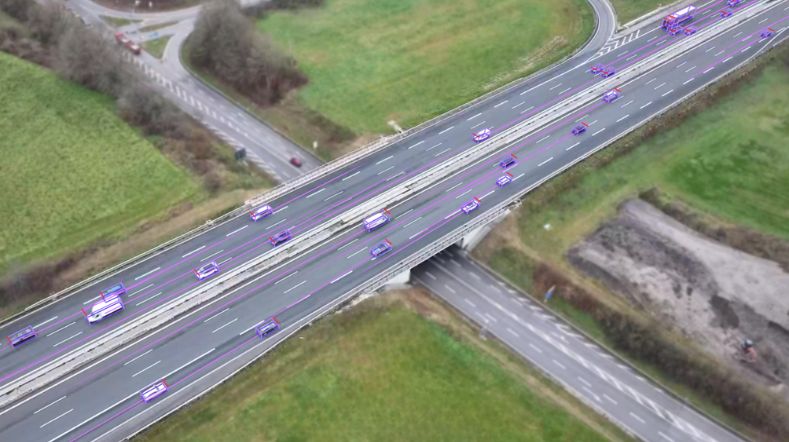
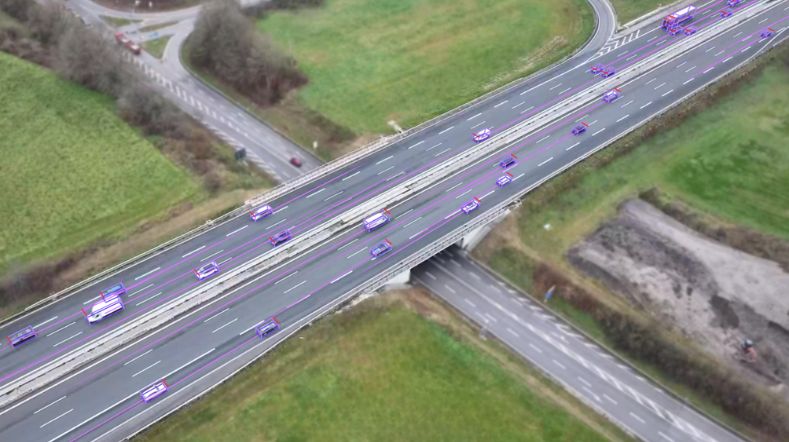
MARQ opens its doors: a place to collaborate on the mobility of the future
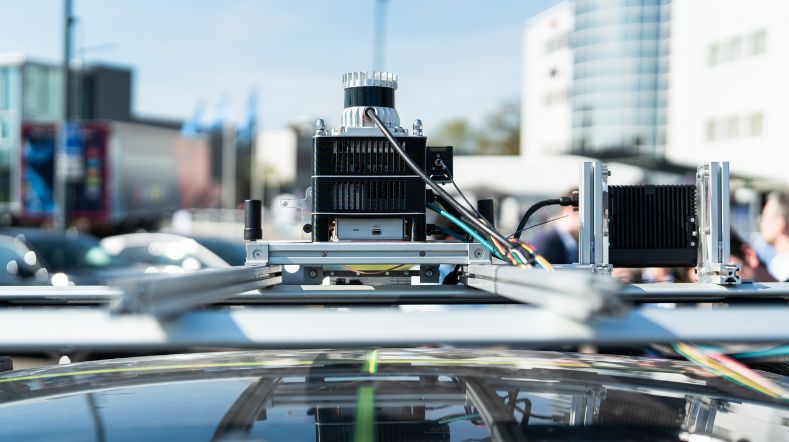
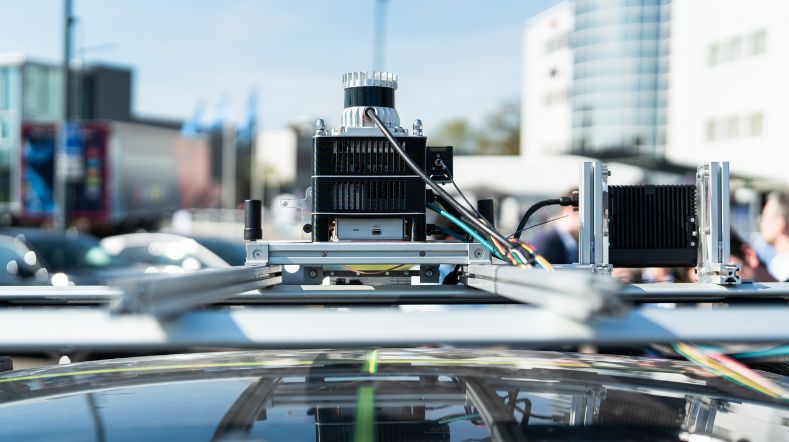
Demonstrations of automated driving and charging for logistics at Maasvlakte
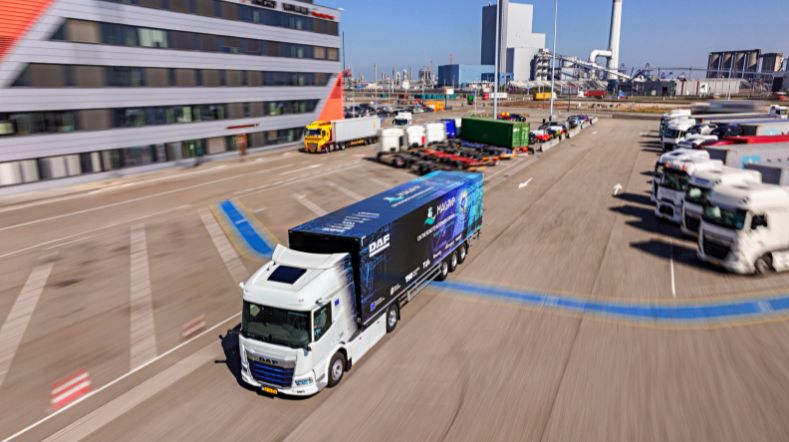
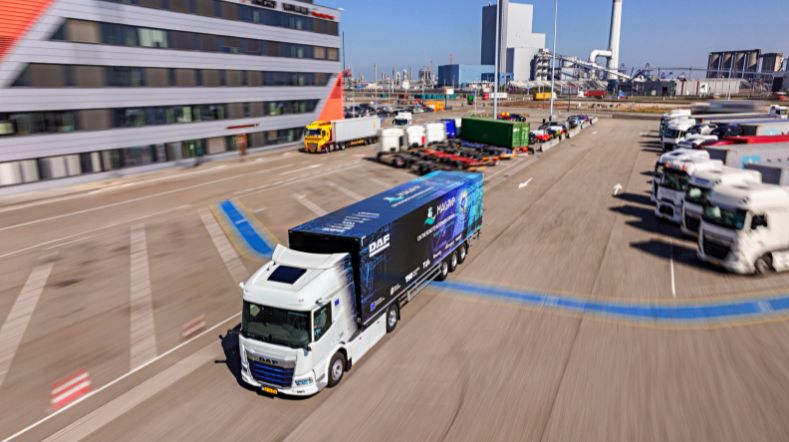
Getting on board with autonomous transport? Five things you need to know
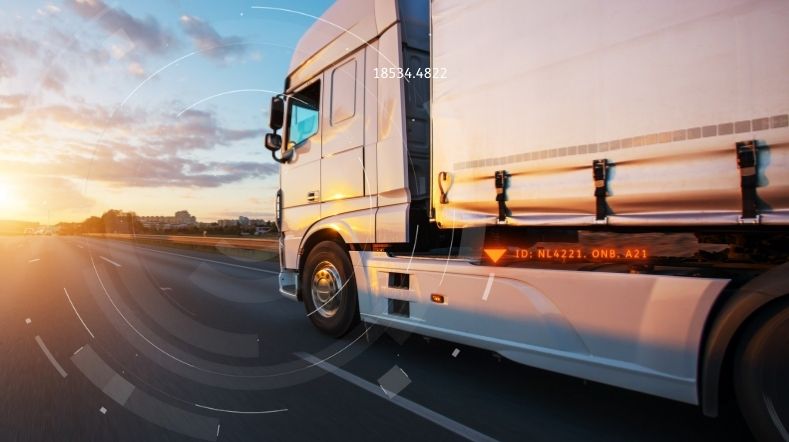
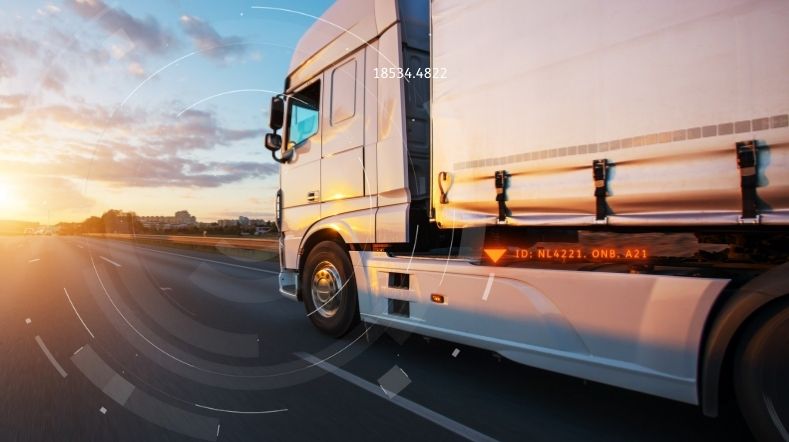
Connected mobility
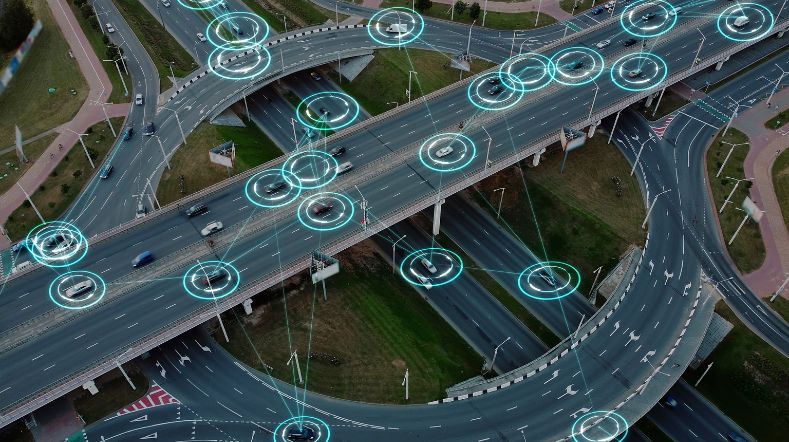
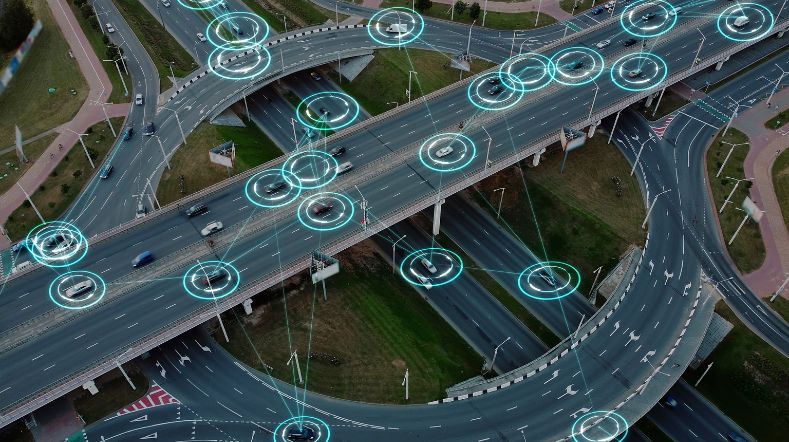