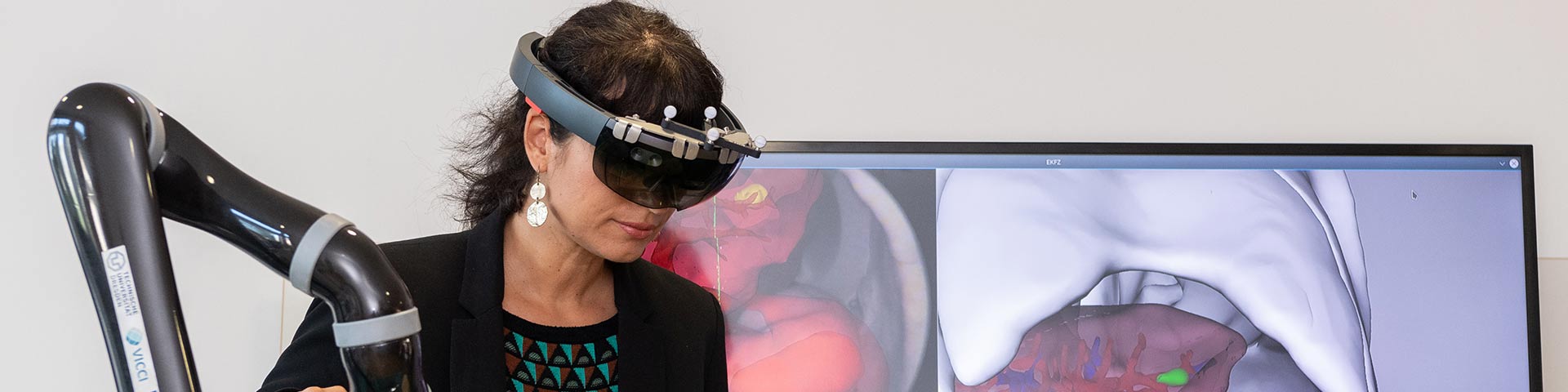
Responsible decision-making between people and machines
Prejudices in facial recognition and recruitment systems. Accidents involving self-driving cars. This type of failure shows that much remains to be done in the development of AI. The fastest way of moving that development forward is for AI and people to work closely together.
Let us not deny that there have been some major success stories thanks to artificial intelligence. For example, there are AI systems that are able to lip read or recognise tumours, with the help of deep learning. But AI systems also regularly slip up, and that can have very serious consequences. This is especially the case with ethically sensitive applications or in situations in which safety is at stake.
It is therefore time for the next step – a closer partnership between AI and people. This will enable us to develop AI systems that can assist us when taking complex decisions, and with which we can work enjoyably and safely.
AI still faces a stiff learning curve
To start with, AI has a tendency to unquestioningly replicate human prejudices. This is most particularly a problem if social and ethically sensitive applications are involved. Examples include the recruiting of new employees or predicting the likelihood of a delinquent lapsing into crime.
Another case in point is that of opaque decision-making processes. AI systems have to learn to be more transparent. This is certainly true if they are to be used in sensitive contexts, such as law enforcement or detecting social security fraud.
AI systems must not be rigid. They have to adapt to their users and to the changes in society, without losing sight of ethical and legal principles.
Finally, data analysed by AI may be private and confidential. This is especially the case when businesses and organisations operate together in a decision-making system.
What artificial intelligence still needs
TNO is currently investigating what is needed to achieve a responsible decision-making process between people and machines. There are four points to consider:
- Responsible AI: by incorporating ethical and legal principles into AI.
- Accountable AI: by enabling different types of user to understand and act upon advice and recommendations given by the system.
- Co-learning: by adapting, with the help of people, to a changing world. This should be done in a way that ensures that ethical and legal principles are anchored in the system.
- Secure learning: by learning from data without actually sharing them with other parties.
The aim: reliable and fair AI
TNO is helping to bring about reliable AI systems that clearly operate fairly. Always. And that includes complex and fast-changing environments. We are seeking to develop AI systems that can explain to different types of user why they take a particular decision.
Delving deep with use cases
It’s perhaps also useful to know that, in addition to this area of research, we are also working on safe autonomous systems in an open world. In other words, we are exploring solutions to today’s challenges facing AI from a range of perspectives. But the focus here is very much on ‘responsible decision-making processes between people and machines’. With the help of the following use cases, we are trying out our AI technology in practical situations:
- Augmented worker for smart industry
- Diagnosing for printer maintenance
- Energy balancing for smart homes
- Fair decision making in justice
- Fair decision making in the job market
- Innovation monitoring in policy
- Predictive AI in healthcare
- Secure learning in oncology research
- Secure learning in money laundering detection
- Subsidence monitoring
Get inspired
Working on reliable AI
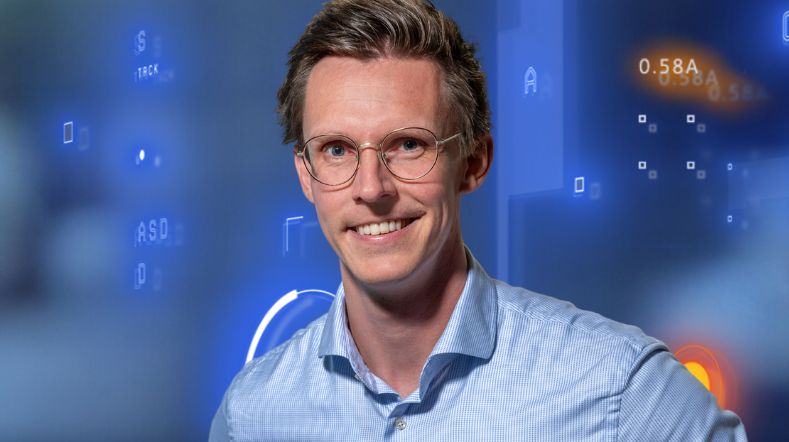
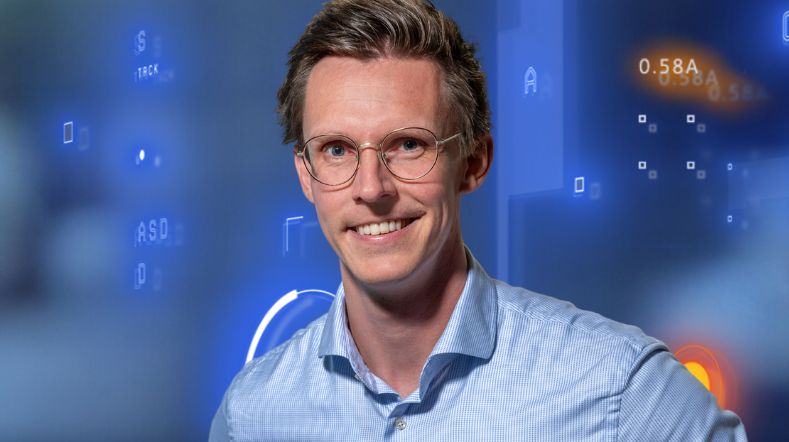
AI model for personalised healthy lifestyle advice
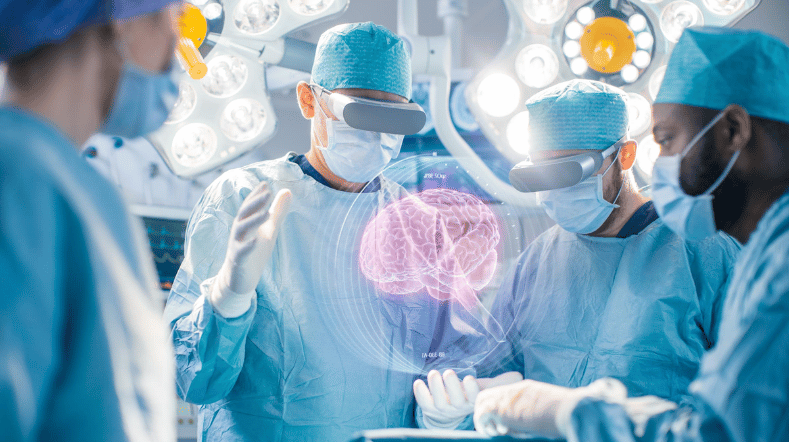
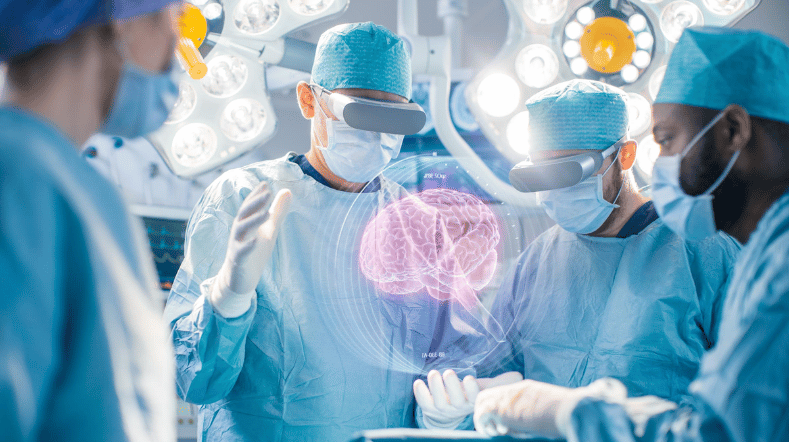
AI in training: FATE develops digital doctor's assistant
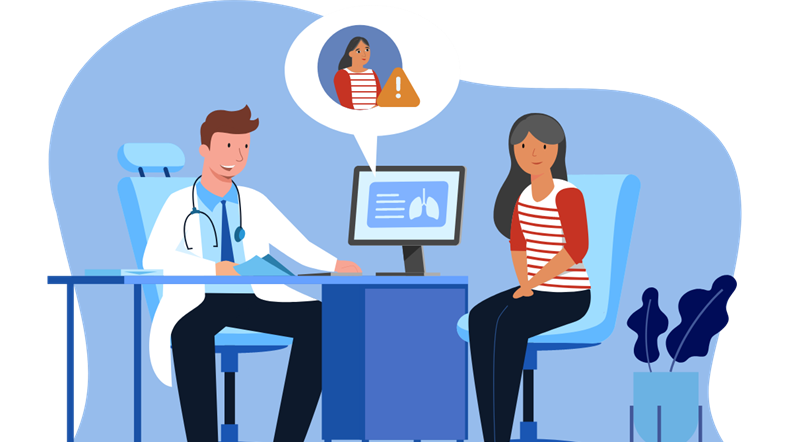
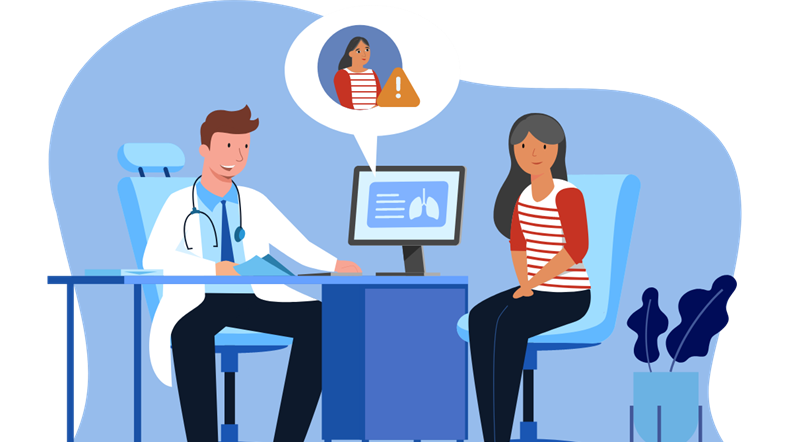
Boost for TNO facilities for sustainable mobility, bio-based construction and AI
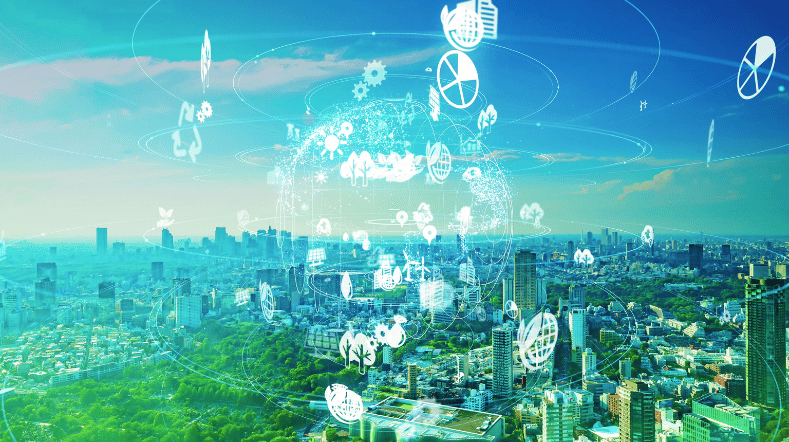
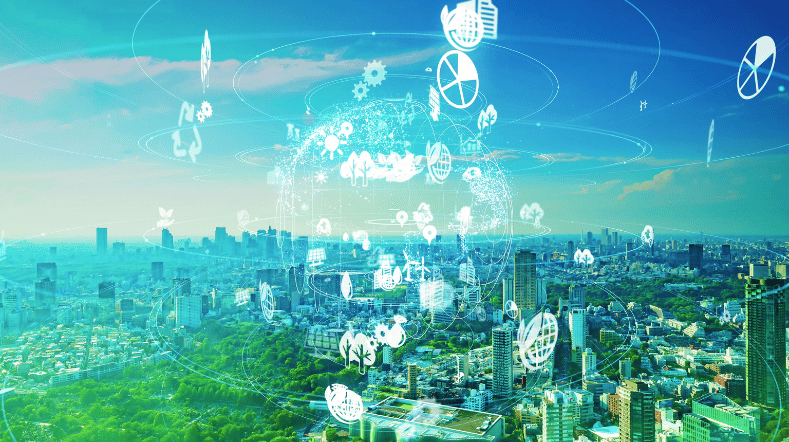
GPT-NL boosts Dutch AI autonomy, knowledge, and technology
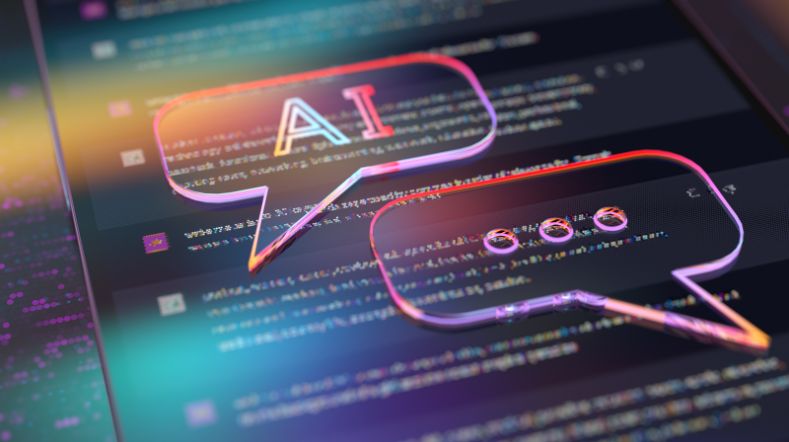
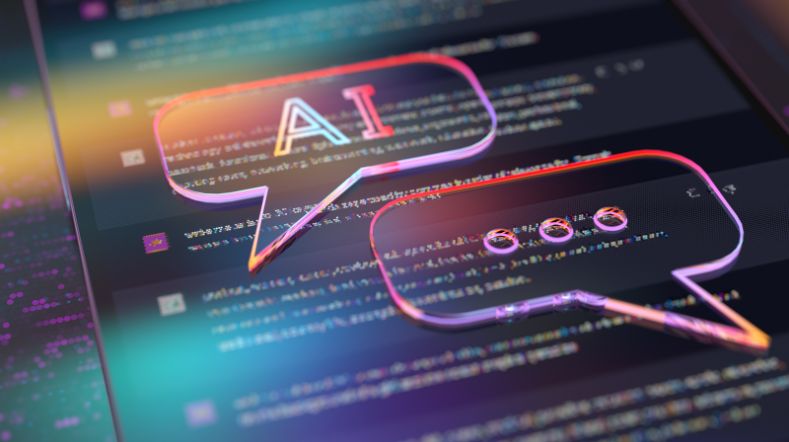