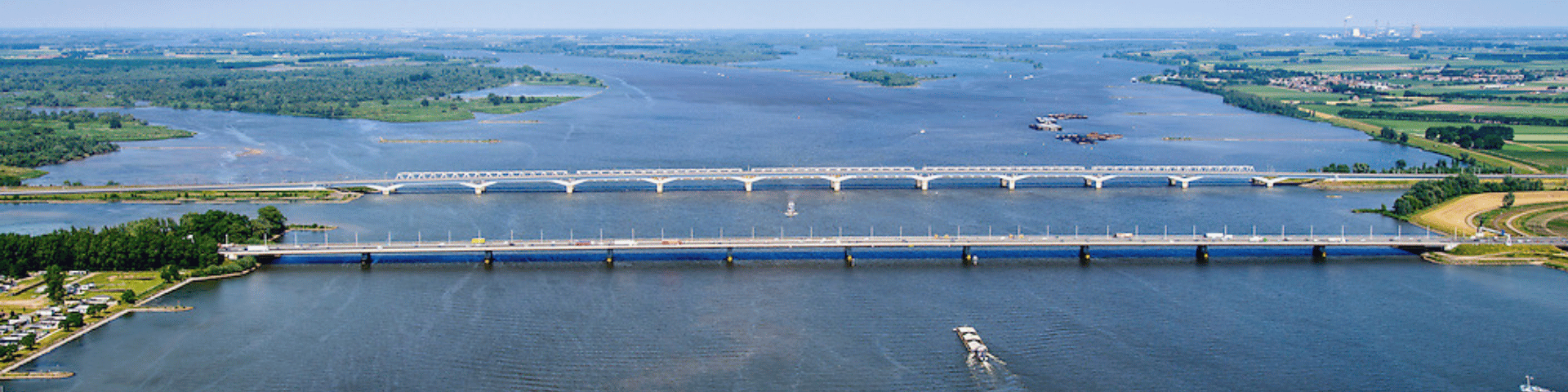
Predictive Twin Lab for the built environment
Predictive twins can help predict and influence the future behaviour and use of structures, or even entire neighbourhoods and cities. With the Predictive Twin Lab, TNO inspires and stimulates builders, designers, managers, policymakers and companies to work together on applicable forms of predictive twins. In this way, we can jointly make an impact for a future-proof living environment for everyone.
Future-proof living environment
Predictive twins offer promising applications for the major societal challenges that impact our living environment. With increasing urbanisation in our country and great transitions in energy and sustainability, it is becoming increasingly difficult to plan, build, and keep cities future-proof.
The expansion of the power grid to prevent grid congestion, the substantial renovation and replacement task of our civil infrastructure, as well as the large housing construction task, and the facilitation of our future mobility, increasingly require foresight.
The implementation of predictive twins can assist us in achieving that. A predictive twin goes beyond the capabilities of a standard digital twin by making future predictions based on measurement data. Buildings are generating more and more data, so the deployment of predictive twins can provide valuable insights about their future use and behaviour.
How does a predictive twin work?
The predictive twin is a digital replica of a physical structure or collection of structures. This could be a bridge, tunnel, house or office, but also, for example, a neighbourhood or an entire city.
Unlike a Building Information Model (BIM), a predictive twin is actually connected to the physical structure via sensors. The predictive twin can monitor and assess the physical twin during its life cycle and then also learn from it.
This allows builders, designers, managers and policymakers to make proactive decisions about renovation, replacement or maintenance.
Examples of predictive twins
Time setters: Urban Strategy maps bottlenecks in city expansion
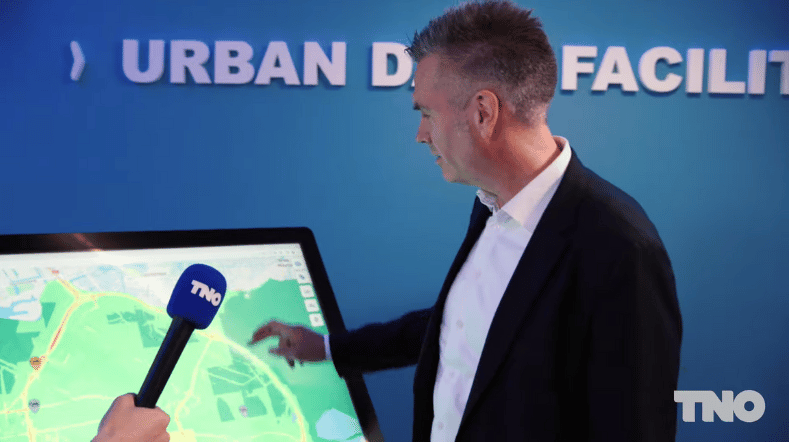
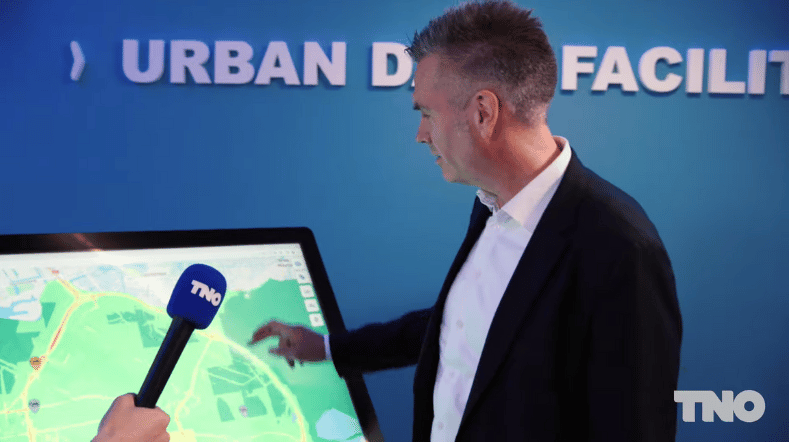
In2Innovation: Digital replicas with predictive capability
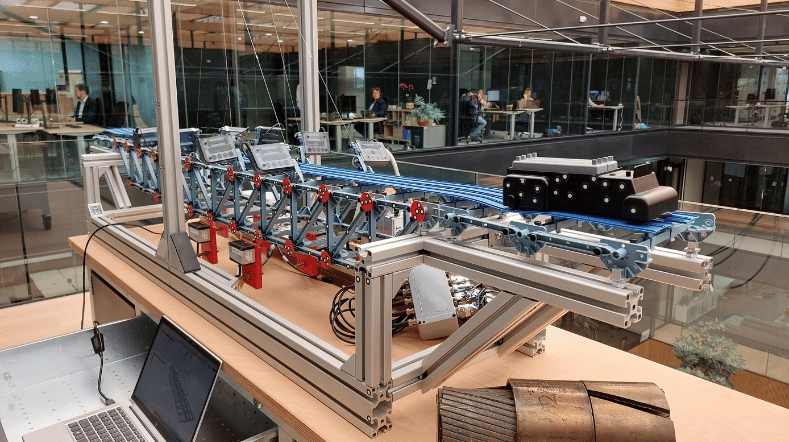
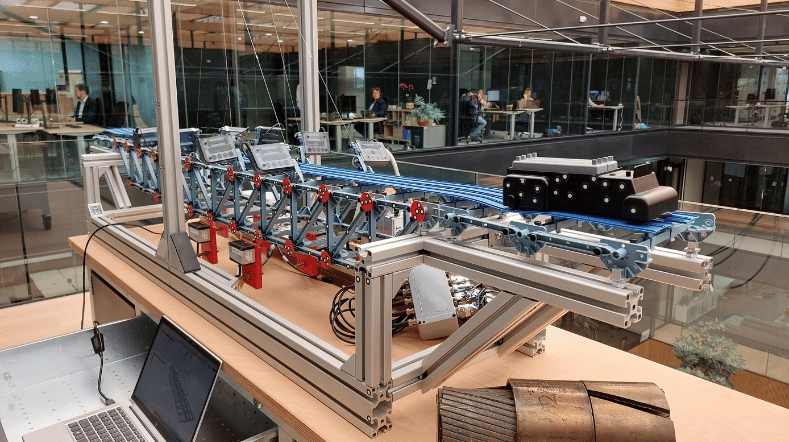
XCARCITY: effective digital twins for tomorrow’s low-traffic city
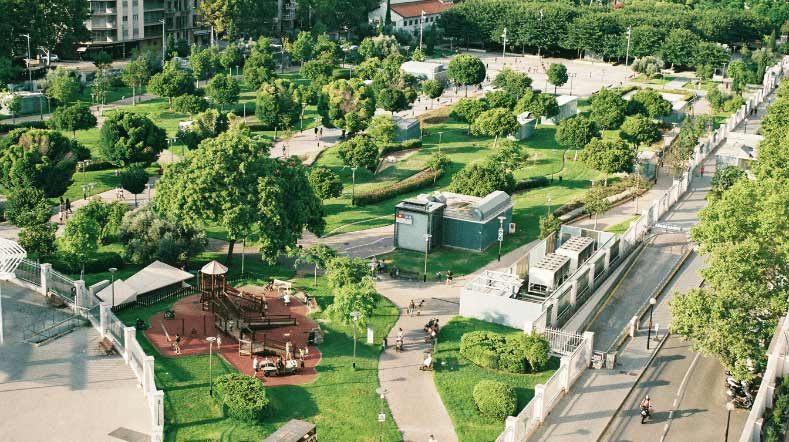
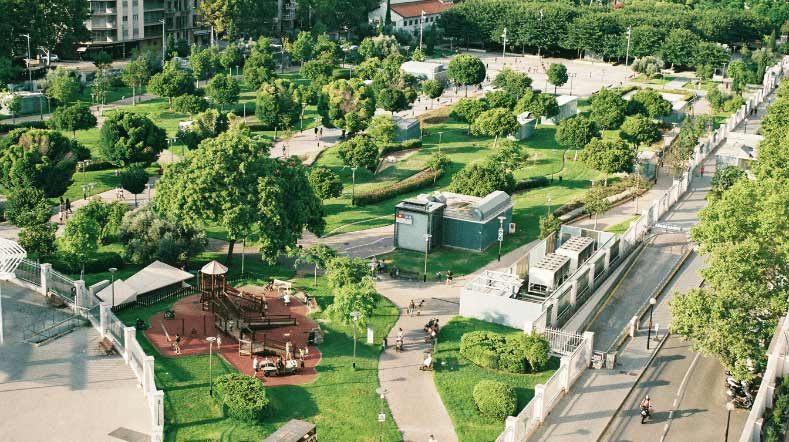
Urban strategy: Local digital twins for sustainable mobility and liveable cities
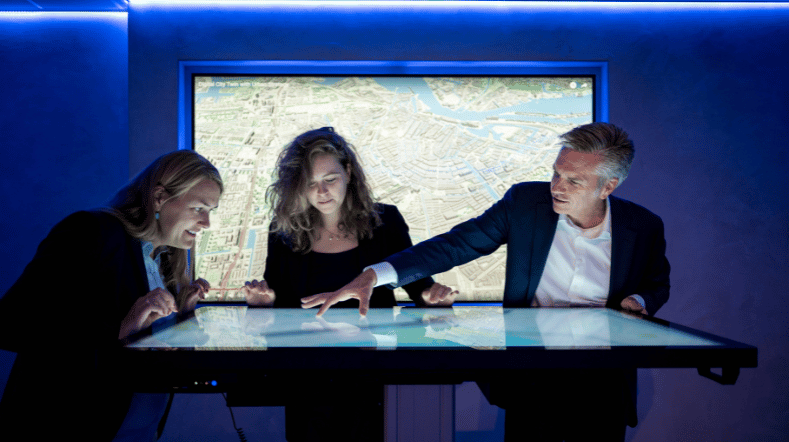
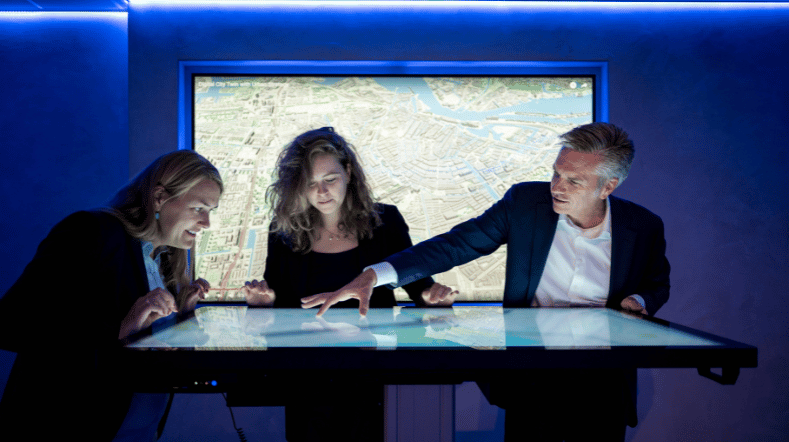
Cutting-edge testing facilities
To explore the unique possibilities and numerous applications of these digital replicas, TNO has set up the Predictive Twin Lab in Delft. This lab closes the gap between the real and virtual worlds of models, offering partners cutting-edge facilities and insights to collaboratively propel innovative use cases for digital replicas.
In the replacement and renovation task of infrastructure, predictive twins can help us accurately predict technical lifespan and plan maintenance. Most bridges in our country date from the 1960s and 1970s. The timing for maintenance or replacement, driven by safety concerns, relies on the current load and condition.
In the Predictive Twin Lab, TNO utilises a digital replica of a miniature bridge connected via sensors. Real-time data from the physical bridge's sensors, including the regular load from truck traffic, is fed into the digital replica, endowing it with predictive capabilities. Predictive twins of this nature offer significantly improved insights into the bridge's condition throughout its lifespan, facilitating better planning for replacement and renovation tasks.
This enhanced planning leads to reduced inconvenience and lower costs. While the lab employs a miniature model for demonstration and testing purposes, the outcomes will be further tested and validated in practical scenarios, specifically on the Moerdijk bridge.
Planning maintenance in public spaces is often a complex task. Besides the cost of maintenance itself, there are indirect costs, due to the risk of failure or mitigation measures, such as closing streets or parking spaces.
In addition to accessibility, air quality is also a significant factor. In Amsterdam, addressing the maintenance and renovation of quay walls poses a considerable challenge. To assess the repercussions and costs, the City of Amsterdam employs Urban Strategy. This multidisciplinary planning platform from TNO utilizes Predictive Twins to compute urban planning considerations and policies, evaluating their impact on accessibility, sustainability, and noise pollution.
Using digital replicas, simulations were carried out for quay maintenance within an area of 70 quay walls over a 20-year period. Based on 25 variables, so-called action-state pairs, the direct and indirect costs of maintenance and renovation were examined. This ultimately resulted in an optimal maintenance strategy with the lowest possible cost and impact on the city and its residents.
The Netherlands holds a global leadership position in greenhouse technology. The thriving exports of high-tech greenhouses amount to approximately €2 billion annually, primarily for vegetable cultivation. Entrepreneurs in greenhouse horticulture place substantial reliance on sustainable and efficiently operating greenhouse structures to guarantee a dependable supply to their customers.
Unforeseen damages or maintenance work can result in immediate and significant consequences, both financially and in terms of food production security. In the Predictive Twin Lab, we use a scale model of a greenhouse deck to demonstrate potential real-life effects. This approach enables the accurate determination of the optimal timing for maintenance or repair. Sensors installed on the greenhouse structure track loads induced by crops, wind, and snow. Simulation models analyse real-time measurement data, predicting the impact on the greenhouse structure and allowing for timely intervention measures.
The predictive twin technology holds significance not only for horticulturists but also for greenhouse builders who provide warranties for the greenhouse's lifespan. These companies can monitor adherence to design agreements and ensure the greenhouse's usage aligns with specifications. Furthermore, predictive twins assist greenhouse builders in refining optimal greenhouse constructions based on performance-maximizing predictions.
TNO is also in the process of developing a predictive twin to monitor and adjust climate conditions and plant growth in a physical greenhouse, leveraging predictions and AI optimization for enhanced efficiency. These applications illustrate the power of predictive twins, strengthening the competitive position of Dutch greenhouse horticulture.
Space is an increasingly scarce commodity in cities, leading to more frequent use of high-rise buildings. Tall buildings have a major impact on their surroundings and construction involves uncertainties and risks. For instance, the vibration behaviour of new high-rise buildings proves difficult to predict, especially on the relatively soft subsoil we have in the Netherlands. Excessive vibrations can have a negative impact on residents' or employees' comfort and sense of safety.
For existing tall buildings, structural reliability is often difficult to estimate. Due to the structural uncertainties of the calculation model, calculations are made more conservatively, resulting in additional material use and higher costs. In the Predictive Twin Lab, TNO uses a physical scale model of a residential tower and a digital twin to investigate measurement techniques to better predict vibration behaviour.
These techniques are then validated with real-life monitoring of three tall structures in Rotterdam: De Zalmhaventorens, New Orleans and Delftse Poort. This provides reliable data on the overall stiffness and foundation stiffness of tall buildings, making calculations for planned high-rise buildings more reliable, and the reassessment of existing buildings more realistic. In this manner, predictive twins can play a vital role in creating comfortable, reliable high-rise buildings while minimizing the use of materials.
Get inspired
TNO nitrogen approach a blueprint for all sustainability challenges
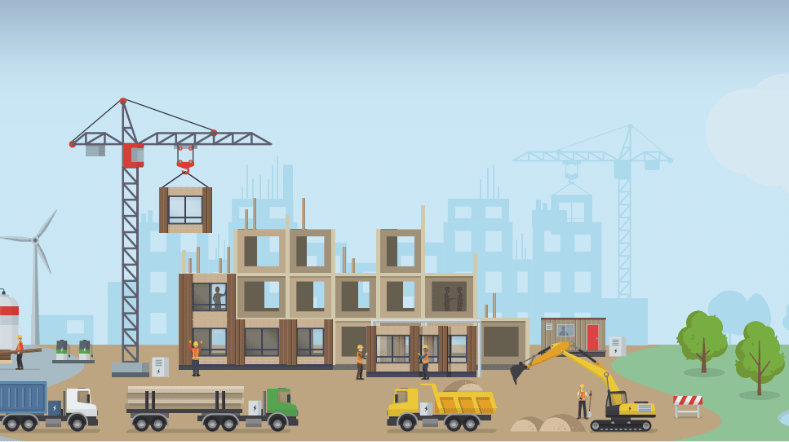
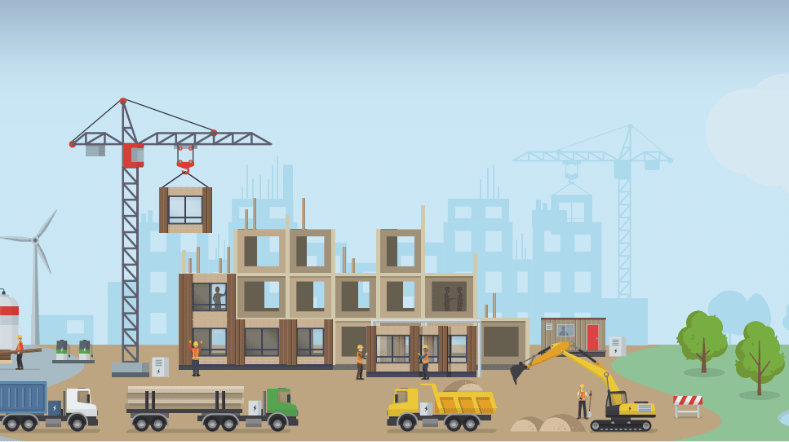
Building transition gets off to a quicker start thanks to Zero-Emission Construction
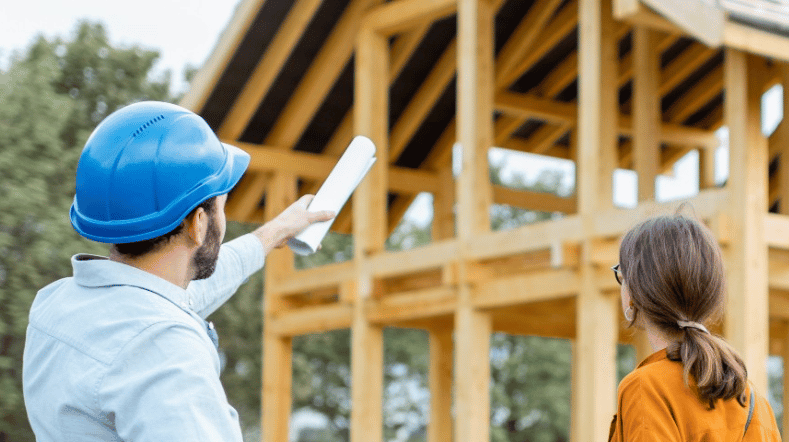
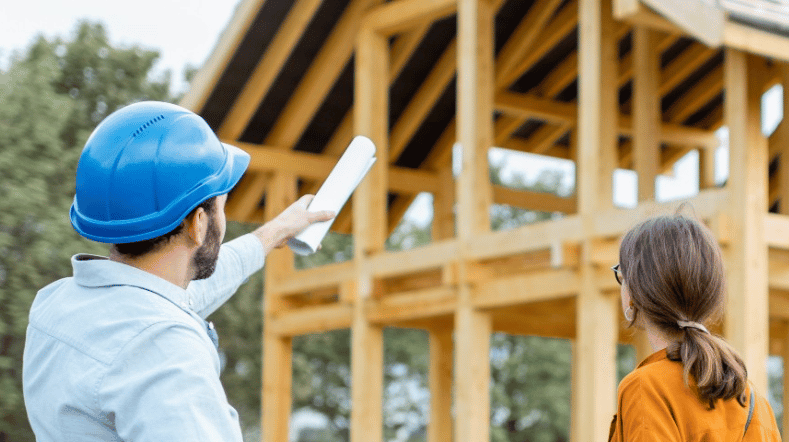
SHM NEXT: time travelling into infrastructure integrity with predictive twins
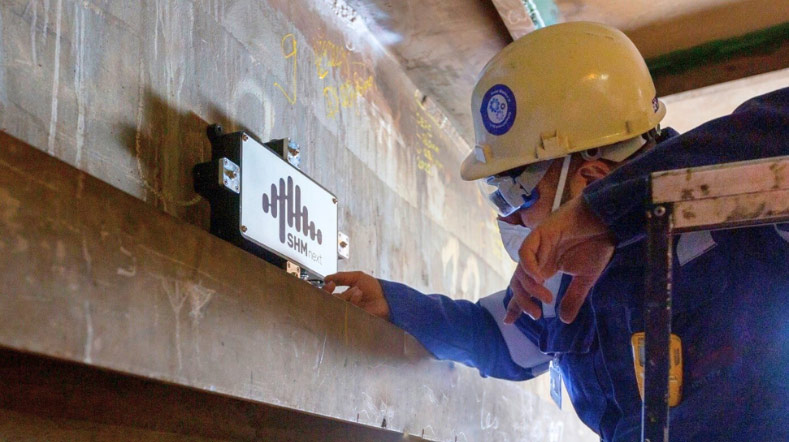
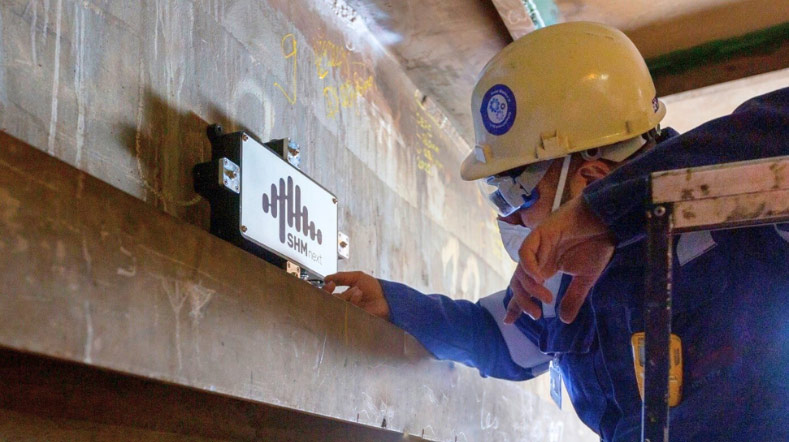
Data-driven approach increases road maintenance efficiency and sustainability
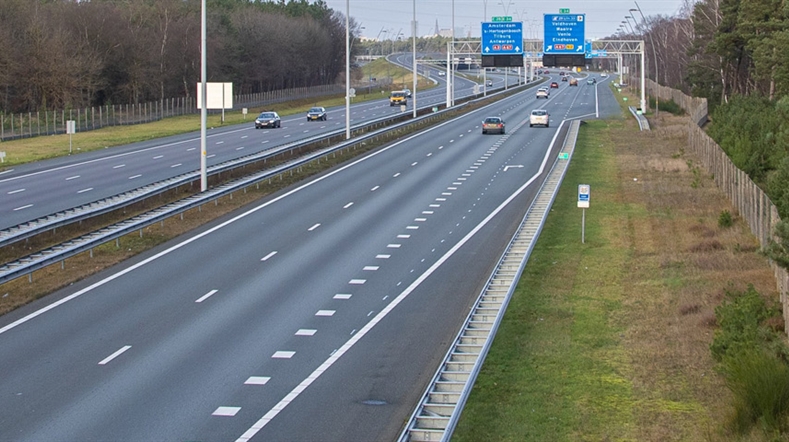
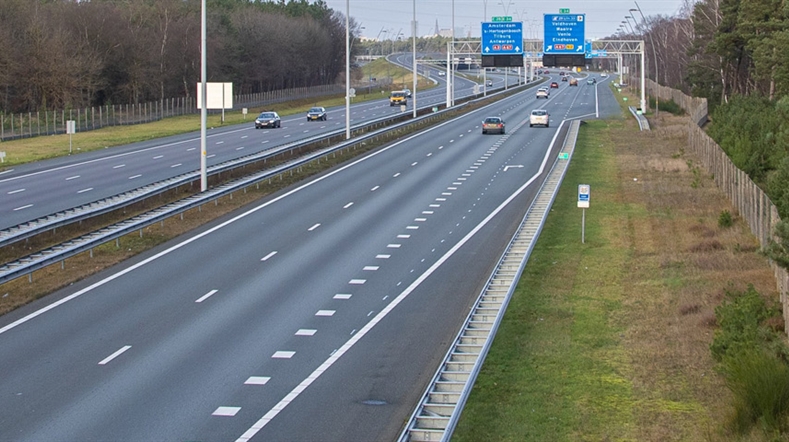
In2Innovation: Digital replicas with predictive capability
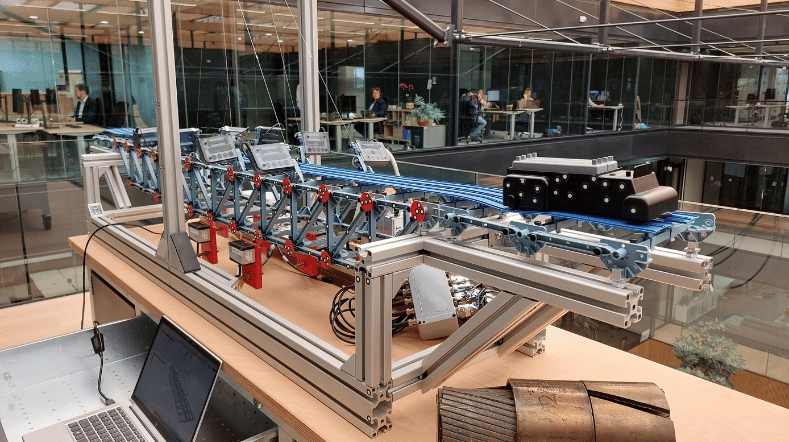
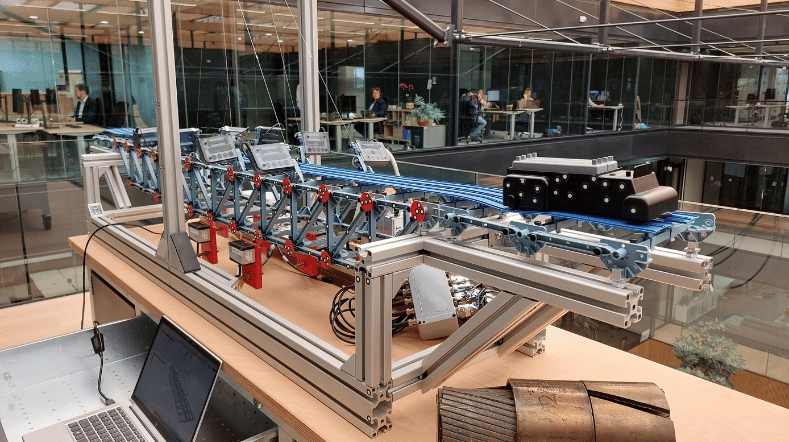