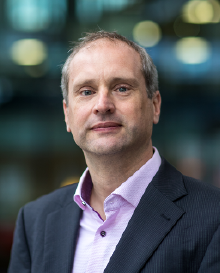
Frank Willems
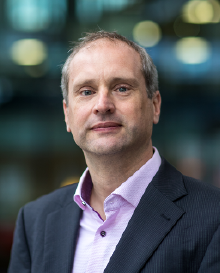
In de transitie naar klimaatneutrale mobiliteit is de ontwikkeling van zelflerende controlesystemen voor duurzame aandrijflijnen cruciaal om minimale energieconsumptie en operationele kosten te garanderen en om ontwikkelingstijd en -kosten te minimaliseren.
Leerstoel
Self-learning powertrains (Faculty Mechanical Engineering, Control Systems Technology, Eindhoven University of Technology).
Onderzoeksgebied
Het meeste goederenvervoer vindt plaats per vrachtwagen of schip. Deze vervoersmiddelen zijn voorzien van heavy-duty aandrijfsystemen die worden aangedreven door verbrandingsmotoren. In de komende decennia blijven verbrandingsmotoren de belangrijkste vorm van aandrijving. In de transitie naar klimaatneutrale mobiliteit neemt de complexiteit van aandrijfsystemen in de toekomst alleen maar toe als gevolg van de elektrificatie en introductie van warmteterugwinning en steeds efficiëntere verbrandingsconcepten.
Regelsystemen vormen het brein achter het aandrijfsysteem. Dergelijke systemen zijn essentieel om het brandstofverbruik te minimaliseren, te zorgen voor betrouwbare rijprestaties in de praktijk en het gebruik van een breed scala aan duurzame brandstoffen mogelijk te maken, waaronder groene waterstof. De ontwikkelingstijd en -kosten voor het ontwikkelen van aandrijfsystemen met de gebruikelijke regelsystemen beginnen zo onaanvaardbaar ver op te lopen dat de industrie in de nabij toekomst voor een keerpunt zal komen te staan.
Om te kunnen omgaan met de complexiteit en diversiteit van de aankomende ultraschone en -efficiënte voertuigen en de ontwikkelingstijd en -kosten tot een minimum te beperken, moeten aandrijfsystemen zelflerend worden. Hiervoor moeten energie- en emissiemanagementstrategieën in het systeem worden geïntegreerd. Mijn onderzoek is gericht op de ontwikkeling van zelflerende regelconcepten, waarbij de energie-efficiëntie van het gehele aandrijfsysteem wordt geoptimaliseerd door middel van slimme sensoren en route-informatie.
Belangrijkste publicaties
- Xia, B. de Jager, M. Donkers & F. Willems (2020). Robust constrained optimization for RCCI engines using nested penalized particle swarm. Control Engineering Practice, 99, [104411]. https://doi.org/10.1016/j.conengprac.2020.104411
- Willems (2018). Is cylinder pressure-based control required to meet future HD legislation? IFAC-PapersOnLine, 51(31), 111-118. https://doi.org/10.1016/j.ifacol.2018.10.021
- Feru, N. Murgovski, B. de Jager, & F. Willems (2016). Supervisory control of a heavy-duty diesel engine with an electrified waste heat recovery system. Control Engineering Practice, 54, 190-201 . https://doi.org/10.1016/j.conengprac.2016.06.001
Helmond - Automotive Campus
Automotive Campus 30
5708 JZ Helmond
Postadres
Postbus 756
5700 AT Helmond