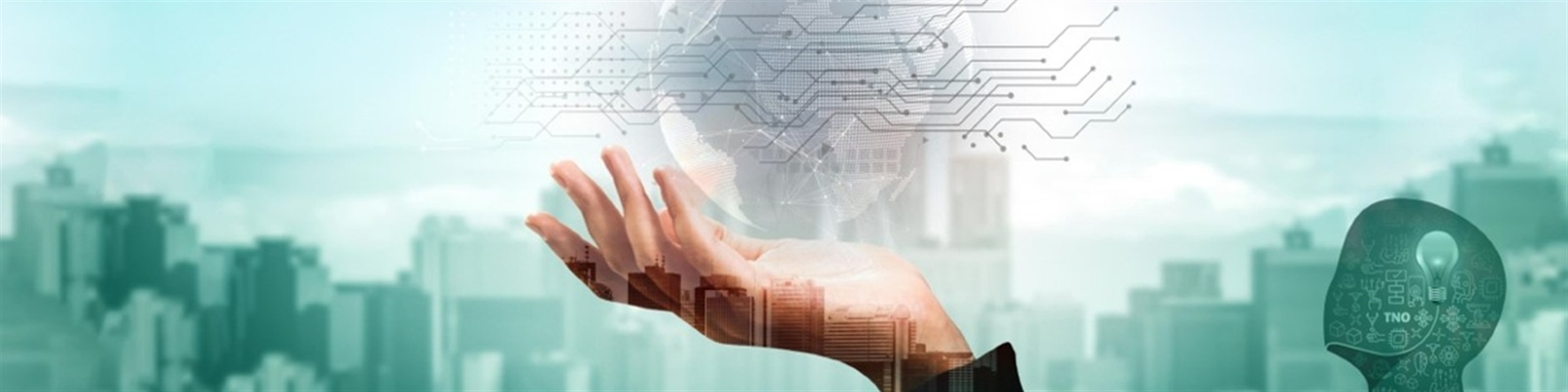
Artificial intelligence: from research to application
The development of Artificial Intelligence (AI) is going fast, and the possibilities are great. As TNO, we’re at the European forefront of AI development. We do so with hundreds of scientists, business developers, and consultants. The big challenge? Bringing together AI expertise and domain knowledge into practical applications. And that’s our strength.
TNO's APPL.AI programme
APPL.AI is the TNO programme that unites many AI developments and areas of expertise. The aim of the programme is to develop human-centred AI that can be responsibly deployed to address societal challenges.
This involves several domains, such as healthcare, mobility, and safety. And it extends beyond research into the technology and potential of AI.
As TNO, we also look at framework conditions (such as regulatory and legal frameworks), and how these can be included in the development and deployment of AI applications.
3 Appl.AI programme lines
The APPL.AI programme currently has three programme lines:
- Safe, autonomous systems in an open world. The development of AI algorithms and software that allow autonomous systems to be deployed safely and effectively in unpredictable and dynamic environments.
- Responsible decision-making between humans and machines. Research in this programme line focuses on responsible and explainable AI, with specific attention to the correct use of data. To arrive at responsible decisions, a safe environment in which humans and AI can learn from each other (co-learning and secure learning) is needed.
- AI Systems Engineering & Lifecycle Management. As TNO, we look at the entire lifecycle of AI systems and seek technical and organisational solutions that improve AI applications’ lifecycle, reliability, and deployability.
3 Angles Appl.AI programme
In the APPL.AI programme, TNO researchers from all disciplines come together to build bridges between AI-based technological innovations and their practical application in organisations. The power of this is:
- AI knowledge – our scientists deploy their broad knowledge of AI in the private and public sector to together arrive at the best-suited technologies and applications. As TNO, we also initiate new research and use the knowledge we’ve gained from the projects we’ve been involved in at other organisations. This is how we’re helping the Netherlands’ continued innovation.
- Domain knowledge – as TNO, we innovate together with the private and public sector across various domains and sectors. Thus, we have intimate knowledge of the current state of affairs. We subsequently link this knowledge to generic issues in business and society.
- First-Time Engineering – our studies show that we’re able to apply AI in a manner well-suited to stakeholders’ needs and situations. We don’t stop at a publication or the creation of a generic tool, but show that it works in practice, and continues to work after learning and updates.
AI applications in 5 domains
We aim to develop AI algorithms and systems that contribute to the goals of the private and public sector. Solutions that make valuable contributions to the economy and society.
We’re therefore involved in AI developments for various domains. Some examples are:
We are working to improve healthcare by developing of a privacy-safe data sharing system for analyzing heath data with AI.
For example, we are working on an AI system that will enable preventive healthcare to better to predict type 2 diabetes.
There are many possibilities with AI in mobility and safety. Today's self-driving vehicles know what to do in commonly occurring situations, but can they also function in unfamiliar environments?
We are therefore facilitating research into situation awareness technology (SAT), which improves the functioning of vehicles in unexpected situations.
The decision making in the autonomous car also plays a role. By training AI algorithms, an autonomous car can make the right decisions on its own.
We’re making strides in the field of cyber security. For instance, we are working as a partner in the development of 'anomaly detection software', offering faster cyberattack detection.
Robots can play an increasingly important role in working with people. That is why we are testing how robots can support people in healthcare, hospitality, and high-risk professions
For example, we are conducting tests with robot dog Spot to deploy robots in dangerous situations instead of humans.
We are developing AI workflows that can support energy production and transportation decisions. In doing so, we are working towards an energy-neutral 2050.
An advanced data analysis model can better predict energy consumption to reduce future energy consumption of homes and industries.
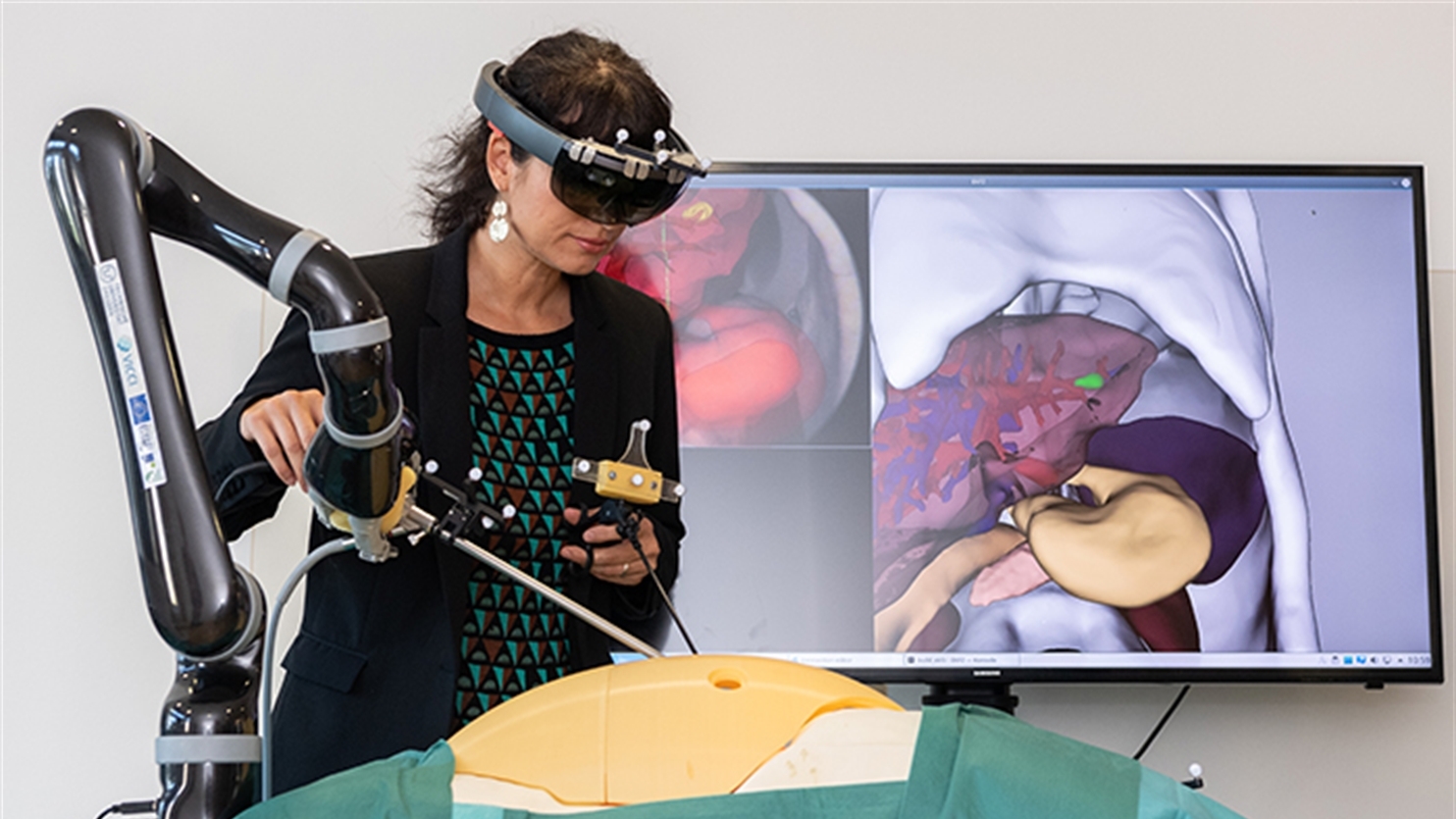
AI technologies
Since we at TNO have helped develop many AI technologies, we understand the main concerns. We proudly help the private and public sector arrive at fitting and responsible AI applications. We’re currently making progress on the following AI technologies:
Future vision of AI in 2032
A lot is already happening. But what is needed to arrive at the desired AI solutions to make the Netherlands safer, healthier, more prosperous, and more sustainable?
During TNO's 90th anniversary in 2022, experts from the organisation wrote a future vision of AI. In it, the expectations of artificial intelligence in ten years and the predictions.
Read TNO's vison of AI
Download the vision paper or watch videos featuring prominent figures on AI, including David Deutsch and Georgette Fijneman.
Diving deep with use cases
To get a good in-depth picture of what TNO Appl.AI does and delivers in concrete terms, you will find more information on the Appl.AI Labs portal.
Collaborating on AI innovations
Are you looking for a partner to explore, further develop and test products and services with AI? Or do you want to test your own AI innovations?
In collaboration, we explore opportunities and experiment with new AI applications. We know the research and development funds available and ways to deploy them where possible.
Get inspired
Working on reliable AI
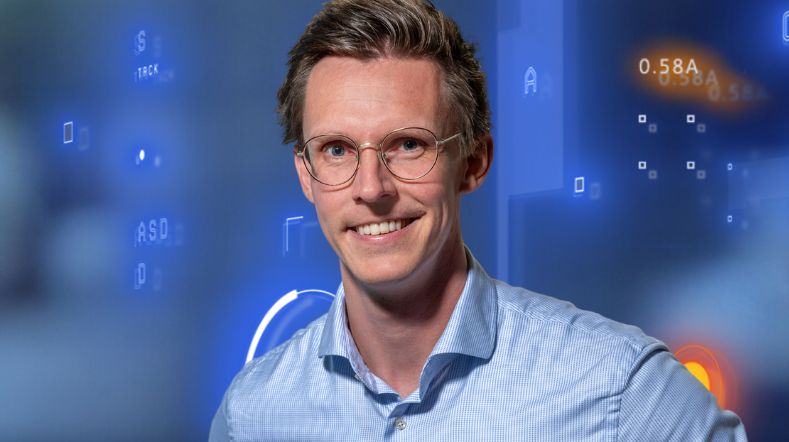
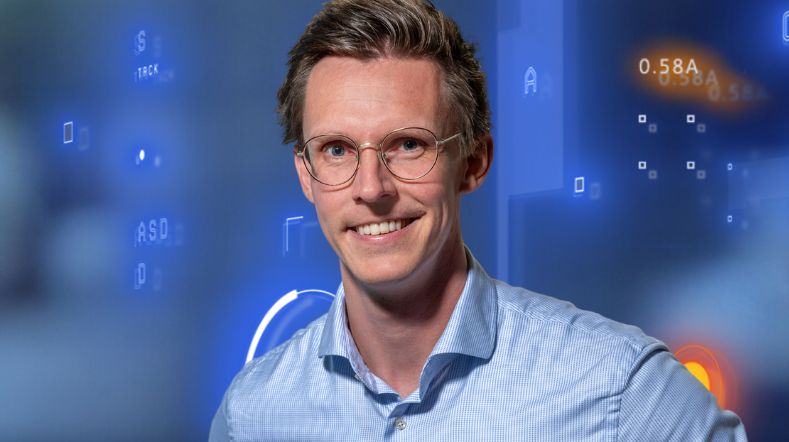
AI model for personalised healthy lifestyle advice
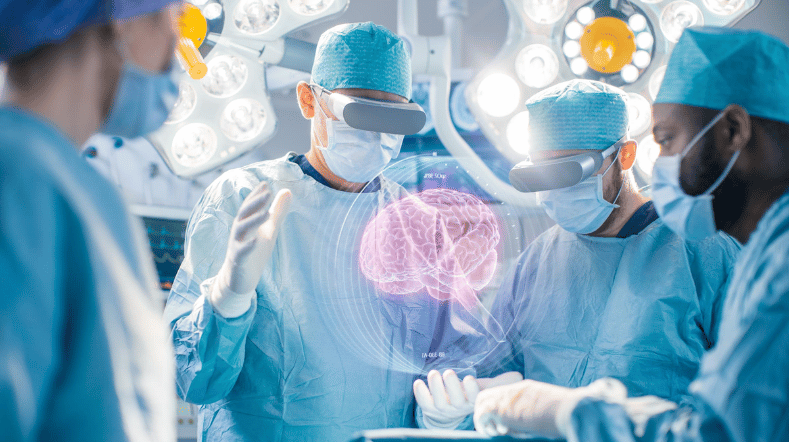
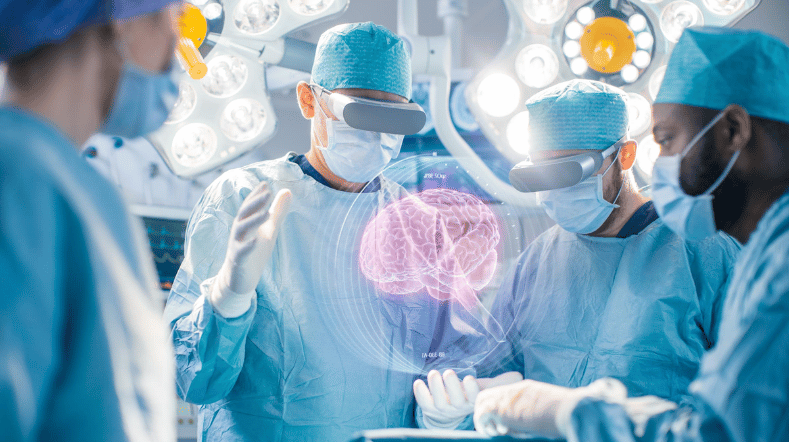
AI in training: FATE develops digital doctor's assistant
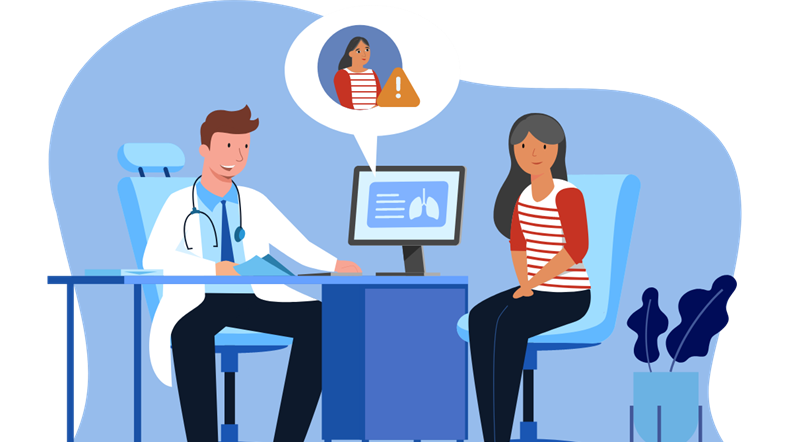
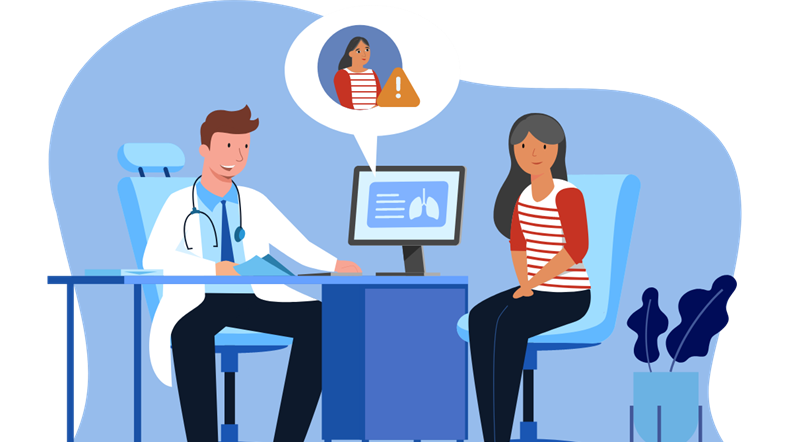
Boost for TNO facilities for sustainable mobility, bio-based construction and AI
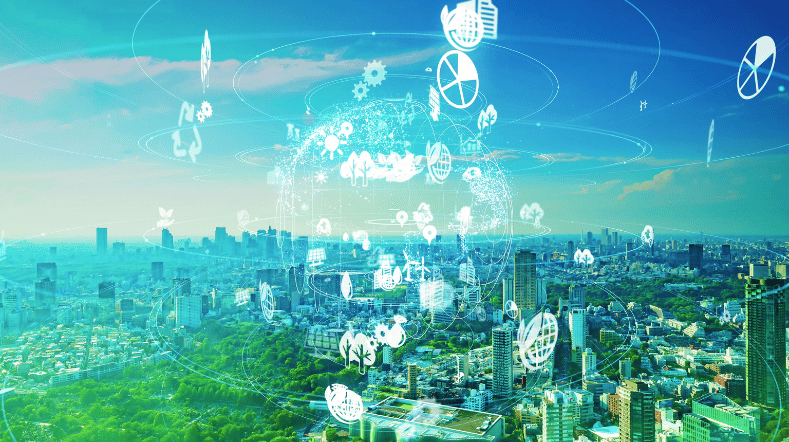
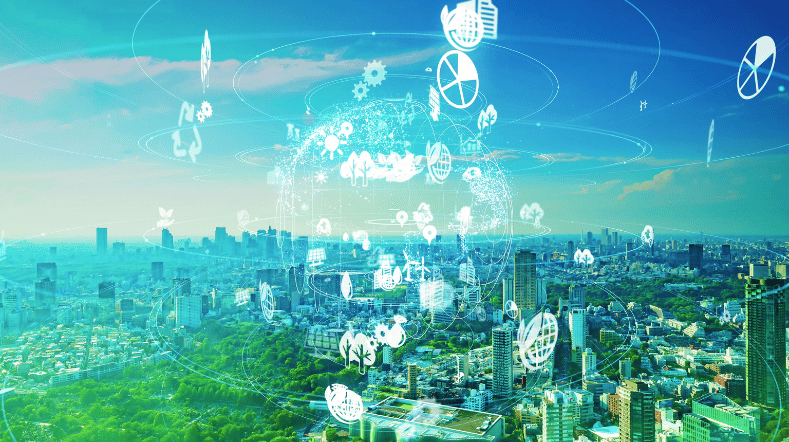
GPT-NL boosts Dutch AI autonomy, knowledge, and technology
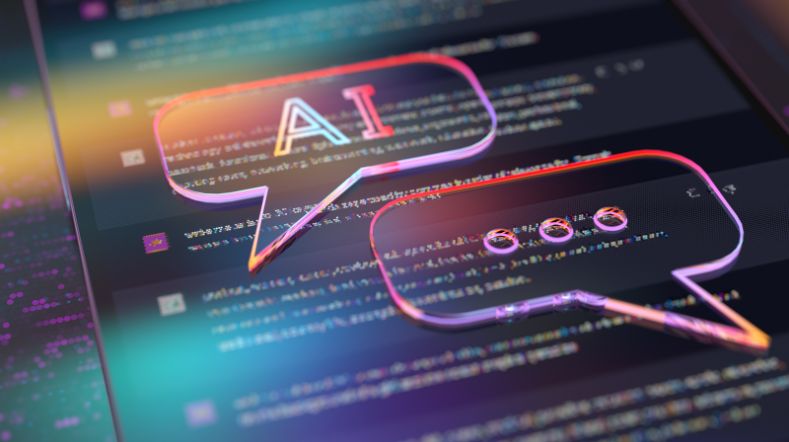
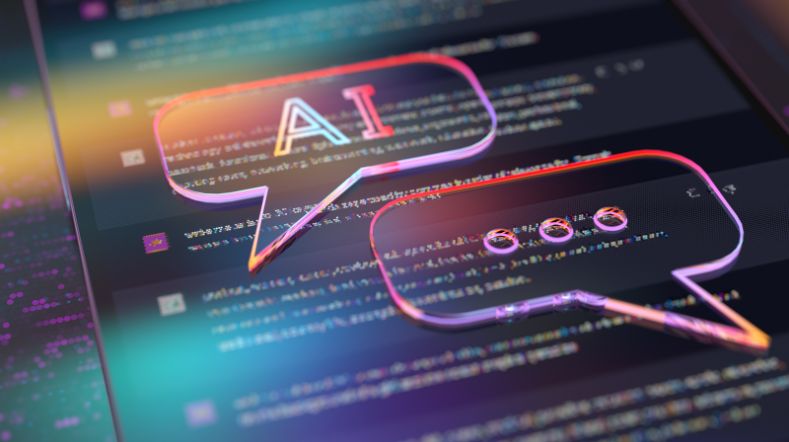